ResumeAtlas: Revisiting Resume Classification with Large-Scale Datasets and Large Language Models
Ahmed Heakl, Youssef Mohamed, Noran Mohamed, Ali Sharkaway, Ahmed Zaky
2024-06-28
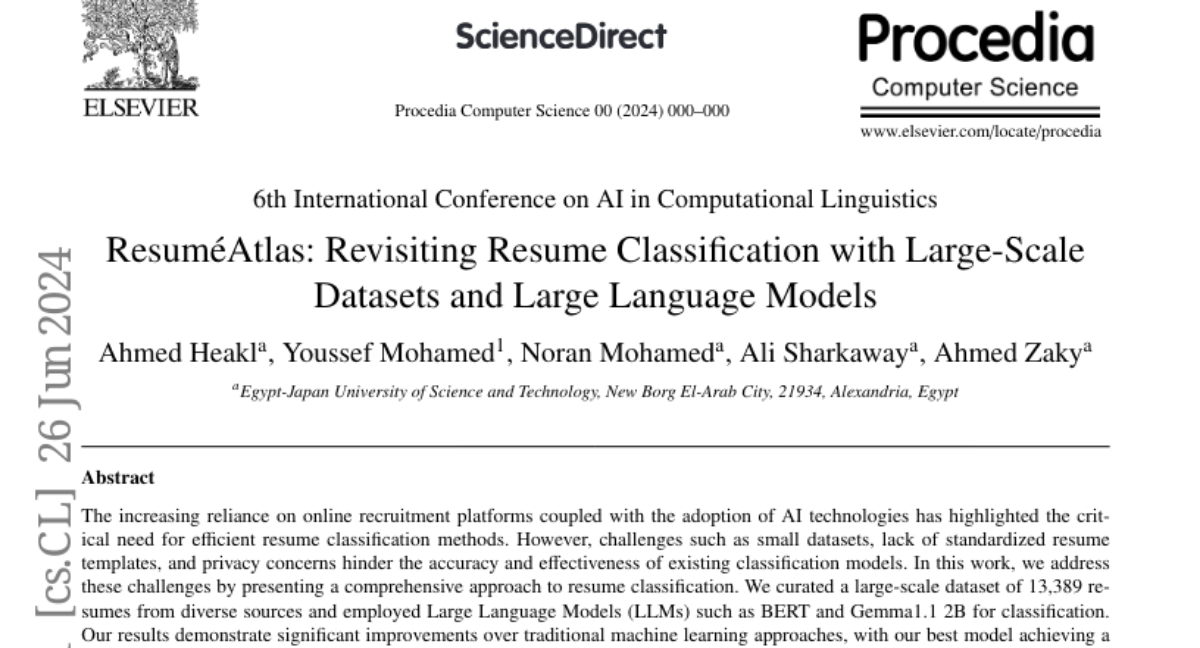
Summary
This paper talks about ResumeAtlas, a new system designed to improve how resumes are classified using large-scale datasets and advanced AI models. It focuses on making the process of sorting resumes more efficient and accurate for online recruitment.
What's the problem?
With the growing use of online job applications, there is a pressing need for better resume classification methods. Current systems face challenges like small datasets that don't represent the variety of resumes out there, a lack of standard formats for resumes, and privacy issues. These limitations can lead to inaccurate results when trying to match candidates with job openings.
What's the solution?
To tackle these issues, the researchers created a large dataset containing 13,389 resumes collected from various sources. They used advanced language models like BERT and Gemma1.1 2B to analyze and classify these resumes. Their approach showed significant improvements over older methods, achieving a top-1 accuracy of 92% and a top-5 accuracy of 97.5%. This means their system can correctly identify the best matches for job roles much more reliably than previous systems.
Why it matters?
This research is important because it enhances the way resumes are processed in online recruitment, making it easier for employers to find suitable candidates. By improving the accuracy of resume classification, ResumeAtlas can help streamline hiring processes, reduce biases in recruitment, and ultimately lead to better job matches for applicants and employers alike.
Abstract
The increasing reliance on online recruitment platforms coupled with the adoption of AI technologies has highlighted the critical need for efficient resume classification methods. However, challenges such as small datasets, lack of standardized resume templates, and privacy concerns hinder the accuracy and effectiveness of existing classification models. In this work, we address these challenges by presenting a comprehensive approach to resume classification. We curated a large-scale dataset of 13,389 resumes from diverse sources and employed Large Language Models (LLMs) such as BERT and Gemma1.1 2B for classification. Our results demonstrate significant improvements over traditional machine learning approaches, with our best model achieving a top-1 accuracy of 92\% and a top-5 accuracy of 97.5\%. These findings underscore the importance of dataset quality and advanced model architectures in enhancing the accuracy and robustness of resume classification systems, thus advancing the field of online recruitment practices.