ReSyncer: Rewiring Style-based Generator for Unified Audio-Visually Synced Facial Performer
Jiazhi Guan, Zhiliang Xu, Hang Zhou, Kaisiyuan Wang, Shengyi He, Zhanwang Zhang, Borong Liang, Haocheng Feng, Errui Ding, Jingtuo Liu, Jingdong Wang, Youjian Zhao, Ziwei Liu
2024-08-07
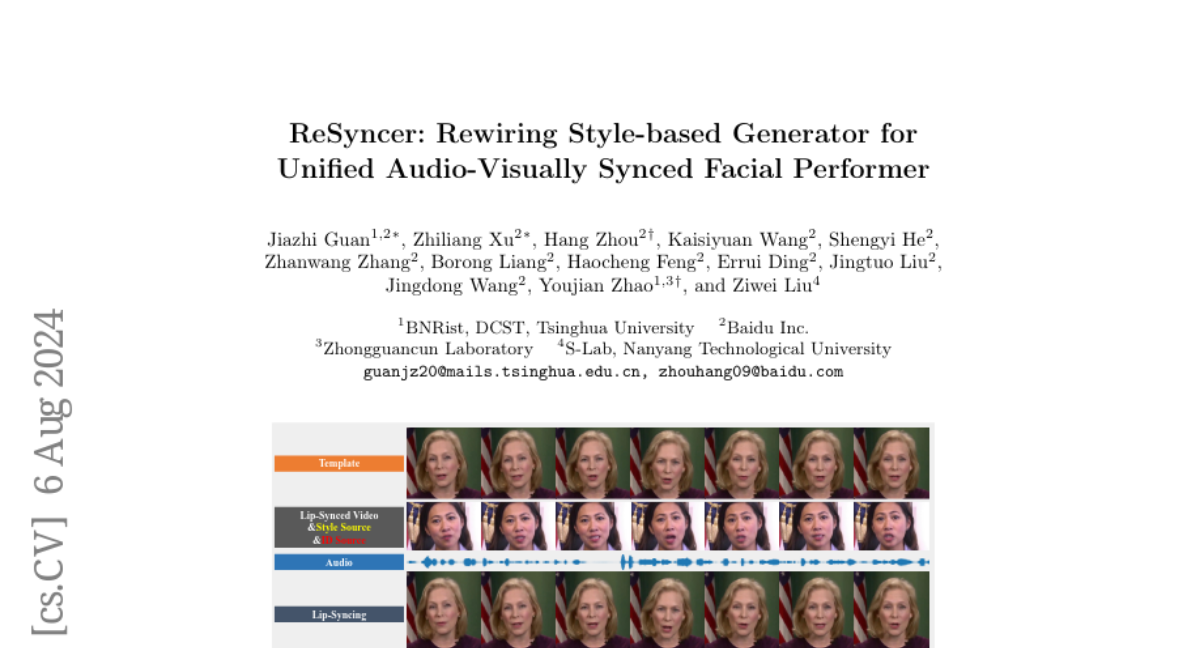
Summary
This paper presents ReSyncer, a new framework designed to create realistic lip-synced videos that match audio perfectly, making it useful for virtual presenters and performers.
What's the problem?
Creating videos where characters' lips move in sync with spoken audio is challenging. Existing methods often require long videos for training or produce noticeable errors, which can make the final product look unnatural. This makes it difficult to create high-quality virtual presenters that can effectively communicate.
What's the solution?
ReSyncer addresses these issues by using a style-based generator that has been reconfigured to better synchronize audio and visual information. It incorporates advanced techniques to predict 3D facial movements and expressions based on audio input. This allows for high-quality lip-syncing while also enabling features like personalized fine-tuning, transferring different speaking styles, and even swapping faces in videos. The framework is designed to work efficiently and effectively across various scenarios.
Why it matters?
This research is significant because it improves the technology behind creating virtual characters that can speak naturally and convincingly. By enhancing lip-syncing capabilities and allowing for flexible customization, ReSyncer opens up new possibilities for applications in entertainment, education, and virtual communication, making digital interactions more engaging and realistic.
Abstract
Lip-syncing videos with given audio is the foundation for various applications including the creation of virtual presenters or performers. While recent studies explore high-fidelity lip-sync with different techniques, their task-orientated models either require long-term videos for clip-specific training or retain visible artifacts. In this paper, we propose a unified and effective framework ReSyncer, that synchronizes generalized audio-visual facial information. The key design is revisiting and rewiring the Style-based generator to efficiently adopt 3D facial dynamics predicted by a principled style-injected Transformer. By simply re-configuring the information insertion mechanisms within the noise and style space, our framework fuses motion and appearance with unified training. Extensive experiments demonstrate that ReSyncer not only produces high-fidelity lip-synced videos according to audio, but also supports multiple appealing properties that are suitable for creating virtual presenters and performers, including fast personalized fine-tuning, video-driven lip-syncing, the transfer of speaking styles, and even face swapping. Resources can be found at https://guanjz20.github.io/projects/ReSyncer.