Rethinking Human Evaluation Protocol for Text-to-Video Models: Enhancing Reliability,Reproducibility, and Practicality
Tianle Zhang, Langtian Ma, Yuchen Yan, Yuchen Zhang, Kai Wang, Yue Yang, Ziyao Guo, Wenqi Shao, Yang You, Yu Qiao, Ping Luo, Kaipeng Zhang
2024-06-17
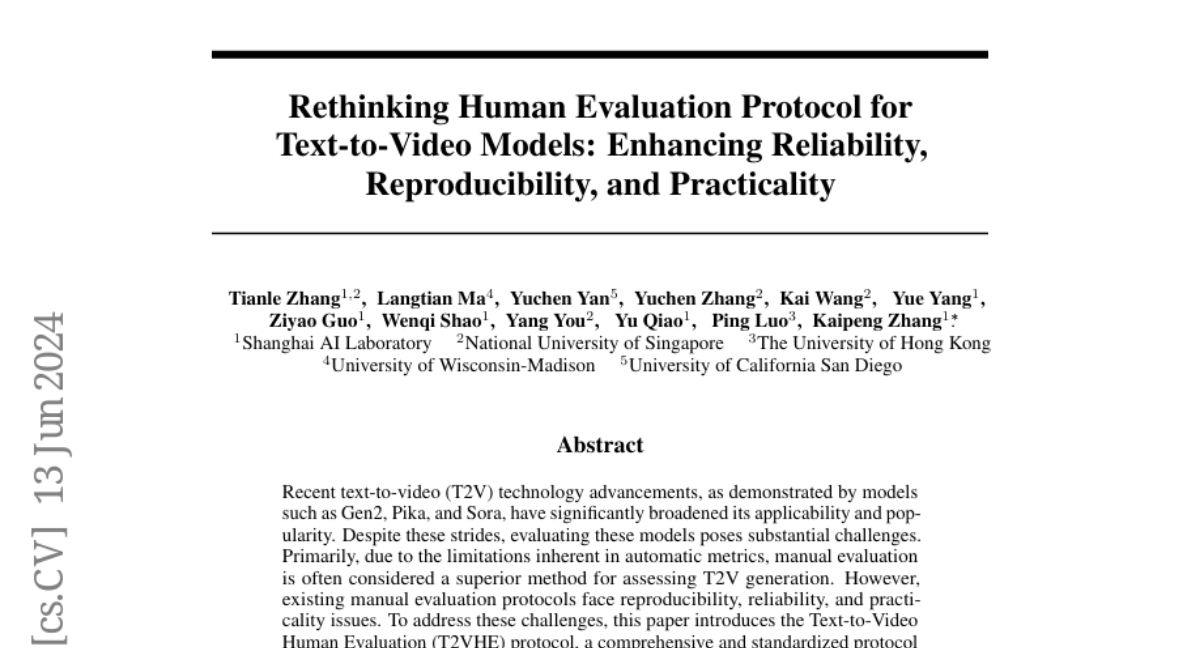
Summary
This paper introduces the Text-to-Video Human Evaluation (T2VHE) protocol, which aims to improve how we evaluate text-to-video models. It addresses the challenges of assessing these models by providing a standardized method for human evaluation.
What's the problem?
As text-to-video technology has advanced, evaluating how well these models perform has become increasingly important. However, current methods for evaluation often rely on automatic metrics that can be unreliable. Manual evaluations are considered better but face issues with reproducibility (getting the same results each time), reliability (trusting the results), and practicality (how easy they are to implement). This makes it difficult to accurately assess the quality of video generated by AI models.
What's the solution?
To solve these challenges, the authors developed the T2VHE protocol, which includes clear metrics for evaluation, comprehensive training for annotators (the people who evaluate the videos), and a dynamic evaluation module that adjusts based on the context. This new protocol helps ensure that evaluations are consistent and high-quality while also reducing costs by nearly 50%. The authors plan to make all aspects of this protocol available to the public, including the workflow and tools needed for implementation.
Why it matters?
This research is significant because it provides a structured way to evaluate text-to-video models, which is crucial as these technologies become more popular and widely used. By improving evaluation methods, T2VHE can help developers create better AI models and assist users in choosing the right tools for their needs. This advancement could lead to higher quality videos generated by AI, enhancing applications in entertainment, education, and more.
Abstract
Recent text-to-video (T2V) technology advancements, as demonstrated by models such as Gen2, Pika, and Sora, have significantly broadened its applicability and popularity. Despite these strides, evaluating these models poses substantial challenges. Primarily, due to the limitations inherent in automatic metrics, manual evaluation is often considered a superior method for assessing T2V generation. However, existing manual evaluation protocols face reproducibility, reliability, and practicality issues. To address these challenges, this paper introduces the Text-to-Video Human Evaluation (T2VHE) protocol, a comprehensive and standardized protocol for T2V models. The T2VHE protocol includes well-defined metrics, thorough annotator training, and an effective dynamic evaluation module. Experimental results demonstrate that this protocol not only ensures high-quality annotations but can also reduce evaluation costs by nearly 50%. We will open-source the entire setup of the T2VHE protocol, including the complete protocol workflow, the dynamic evaluation component details, and the annotation interface code. This will help communities establish more sophisticated human assessment protocols.