Rethinking Token Reduction in MLLMs: Towards a Unified Paradigm for Training-Free Acceleration
Yuhang Han, Xuyang Liu, Pengxiang Ding, Donglin Wang, Honggang Chen, Qingsen Yan, Siteng Huang
2024-11-27
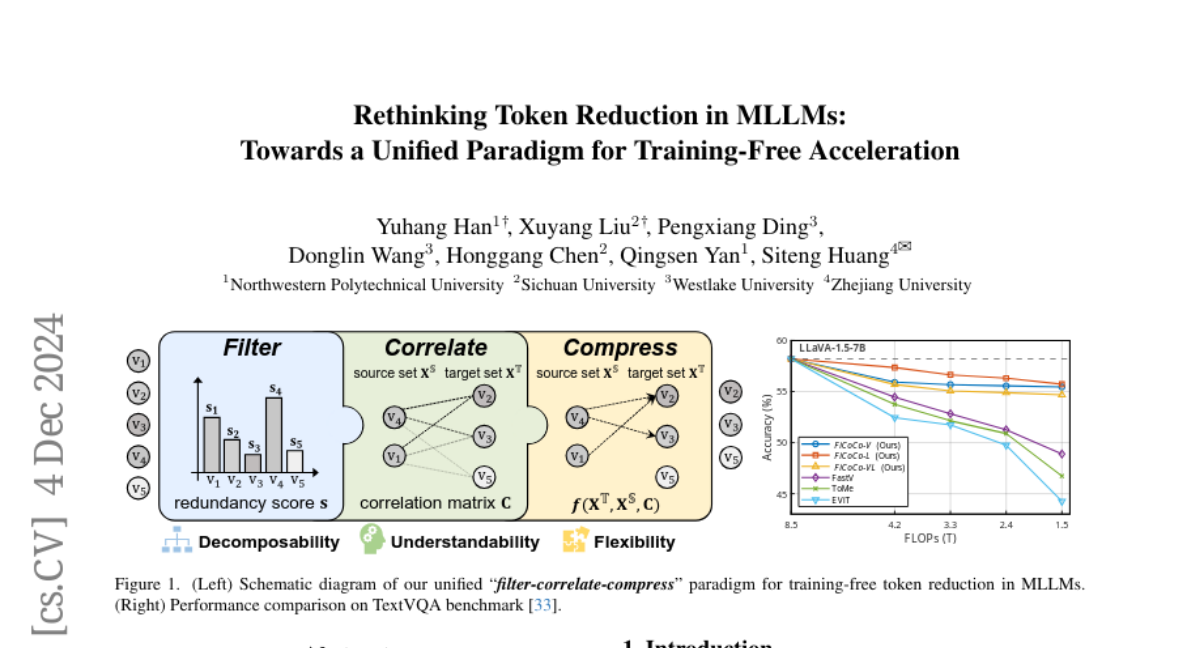
Summary
This paper discusses a new approach to making Multimodal Large Language Models (MLLMs) faster and more efficient by reducing the number of tokens they use without needing additional training.
What's the problem?
MLLMs are powerful tools that can handle both text and images, but they often require a lot of computational resources, which makes them slow and expensive to use. Current methods for reducing the number of tokens (the pieces of data that models process) are not very clear or effective, leading to confusion about how to improve these models.
What's the solution?
The authors propose a unified approach called the 'filter-correlate-compress' paradigm, which breaks down the token reduction process into three clear stages: filtering out unnecessary tokens, correlating important tokens, and compressing the data for efficiency. This method allows for better comparisons and improvements across different models. They also show that their approach can significantly reduce computational costs while maintaining high performance in various tasks.
Why it matters?
This research is important because it helps make advanced AI models more accessible and efficient. By improving how these models handle data, the authors aim to enable faster processing and lower costs, which can benefit a wide range of applications in artificial intelligence, from image recognition to natural language processing.
Abstract
To accelerate the inference of heavy Multimodal Large Language Models (MLLMs), this study rethinks the current landscape of training-free token reduction research. We regret to find that the critical components of existing methods are tightly intertwined, with their interconnections and effects remaining unclear for comparison, transfer, and expansion. Therefore, we propose a unified ''filter-correlate-compress'' paradigm that decomposes the token reduction into three distinct stages within a pipeline, maintaining consistent design objectives and elements while allowing for unique implementations. We additionally demystify the popular works and subsume them into our paradigm to showcase its universality. Finally, we offer a suite of methods grounded in the paradigm, striking a balance between speed and accuracy throughout different phases of the inference. Experimental results across 10 benchmarks indicate that our methods can achieve up to an 82.4% reduction in FLOPs with a minimal impact on performance, simultaneously surpassing state-of-the-art training-free methods. Our project page is at https://ficoco-accelerate.github.io/.