Retrieval-Augmented Decision Transformer: External Memory for In-context RL
Thomas Schmied, Fabian Paischer, Vihang Patil, Markus Hofmarcher, Razvan Pascanu, Sepp Hochreiter
2024-10-10
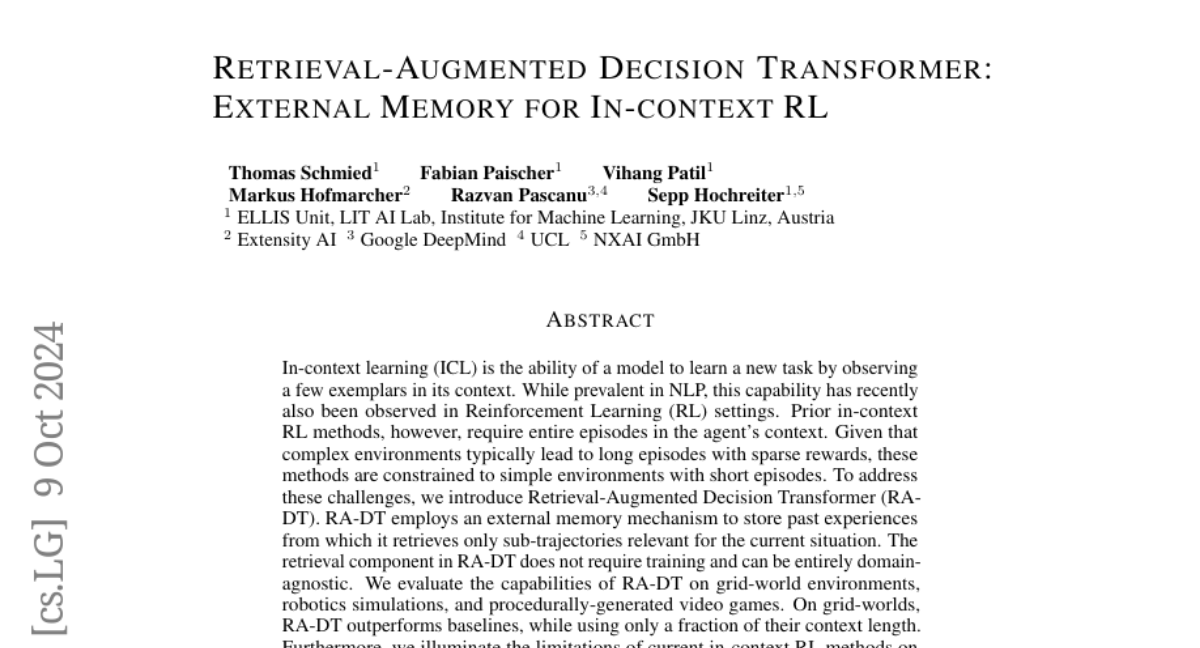
Summary
This paper presents the Retrieval-Augmented Decision Transformer (RA-DT), a new method that improves how reinforcement learning (RL) models learn from past experiences by using external memory.
What's the problem?
Reinforcement learning models often need to learn from long sequences of actions and rewards, but this can be difficult in complex environments where episodes are lengthy and rewards are sparse. Traditional methods require the entire episode to be in the model's context, which limits their effectiveness and makes them suitable only for simpler tasks.
What's the solution?
To solve this problem, the authors introduced RA-DT, which uses an external memory system to store past experiences. Instead of needing the whole episode, RA-DT retrieves only relevant parts of past actions (called sub-trajectories) that help with the current situation. This approach allows the model to learn more efficiently without needing extensive training and works well across various environments like grid-worlds and robotics simulations.
Why it matters?
This research is important because it enhances the capabilities of reinforcement learning models, making them more adaptable and efficient in complex situations. By allowing these models to access relevant past experiences without being overwhelmed by unnecessary information, RA-DT can improve performance in real-world applications such as robotics, gaming, and automated decision-making.
Abstract
In-context learning (ICL) is the ability of a model to learn a new task by observing a few exemplars in its context. While prevalent in NLP, this capability has recently also been observed in Reinforcement Learning (RL) settings. Prior in-context RL methods, however, require entire episodes in the agent's context. Given that complex environments typically lead to long episodes with sparse rewards, these methods are constrained to simple environments with short episodes. To address these challenges, we introduce Retrieval-Augmented Decision Transformer (RA-DT). RA-DT employs an external memory mechanism to store past experiences from which it retrieves only sub-trajectories relevant for the current situation. The retrieval component in RA-DT does not require training and can be entirely domain-agnostic. We evaluate the capabilities of RA-DT on grid-world environments, robotics simulations, and procedurally-generated video games. On grid-worlds, RA-DT outperforms baselines, while using only a fraction of their context length. Furthermore, we illuminate the limitations of current in-context RL methods on complex environments and discuss future directions. To facilitate future research, we release datasets for four of the considered environments.