RetrieveGPT: Merging Prompts and Mathematical Models for Enhanced Code-Mixed Information Retrieval
Aniket Deroy, Subhankar Maity
2024-11-08
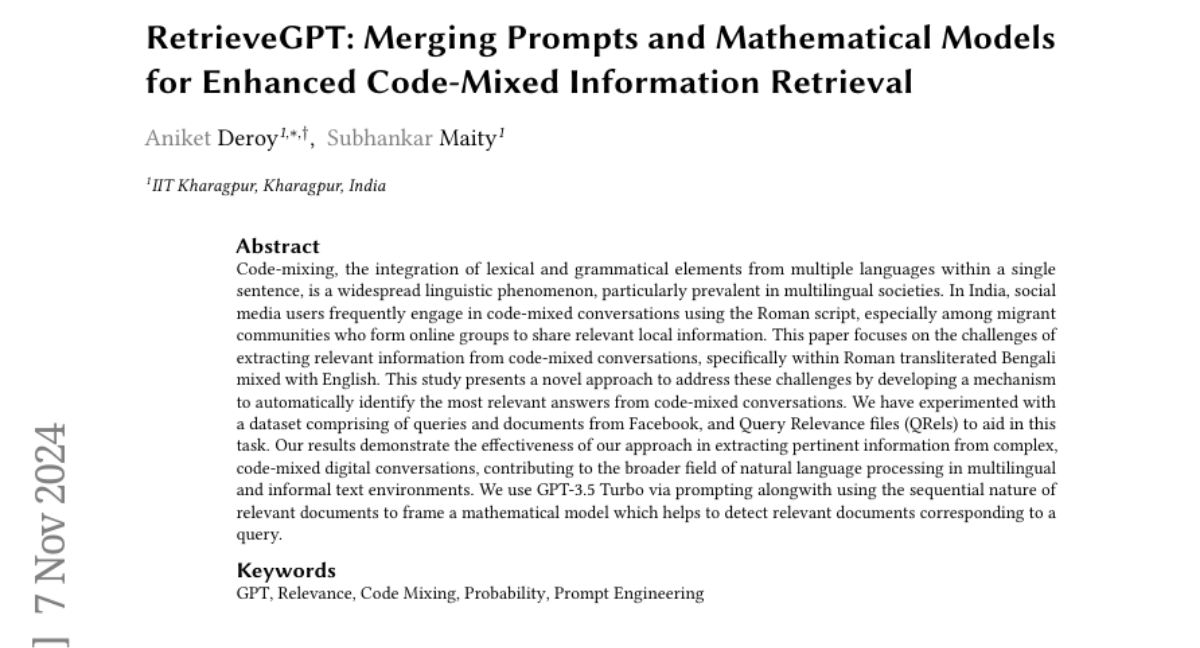
Summary
This paper introduces RetrieveGPT, a new method designed to improve how we find information in conversations that mix different languages, specifically focusing on Bengali written in Roman script combined with English.
What's the problem?
In multilingual settings, people often mix languages in their conversations, which can make it difficult for computers to understand and retrieve relevant information. This is especially true for code-mixed text, where words and grammar from both languages are used together. Current systems struggle to accurately extract useful information from these complex conversations.
What's the solution?
To tackle this challenge, the authors developed a mechanism that uses GPT-3.5 Turbo, a powerful language model, along with a mathematical framework to analyze the relationships between queries and documents. They created a dataset from social media conversations to train and test their approach. The system identifies the most relevant answers from code-mixed discussions by leveraging both prompts and mathematical modeling, which helps improve the accuracy of information retrieval.
Why it matters?
This research is important because it enhances our ability to process and understand code-mixed language, which is increasingly common in today's digital communication. By improving information retrieval in these contexts, RetrieveGPT can help users access relevant information more effectively, benefiting multilingual communities and contributing to advancements in natural language processing.
Abstract
Code-mixing, the integration of lexical and grammatical elements from multiple languages within a single sentence, is a widespread linguistic phenomenon, particularly prevalent in multilingual societies. In India, social media users frequently engage in code-mixed conversations using the Roman script, especially among migrant communities who form online groups to share relevant local information. This paper focuses on the challenges of extracting relevant information from code-mixed conversations, specifically within Roman transliterated Bengali mixed with English. This study presents a novel approach to address these challenges by developing a mechanism to automatically identify the most relevant answers from code-mixed conversations. We have experimented with a dataset comprising of queries and documents from Facebook, and Query Relevance files (QRels) to aid in this task. Our results demonstrate the effectiveness of our approach in extracting pertinent information from complex, code-mixed digital conversations, contributing to the broader field of natural language processing in multilingual and informal text environments. We use GPT-3.5 Turbo via prompting alongwith using the sequential nature of relevant documents to frame a mathematical model which helps to detect relevant documents corresponding to a query.