Reward Steering with Evolutionary Heuristics for Decoding-time Alignment
Chia-Yu Hung, Navonil Majumder, Ambuj Mehrish, Soujanya Poria
2024-06-24
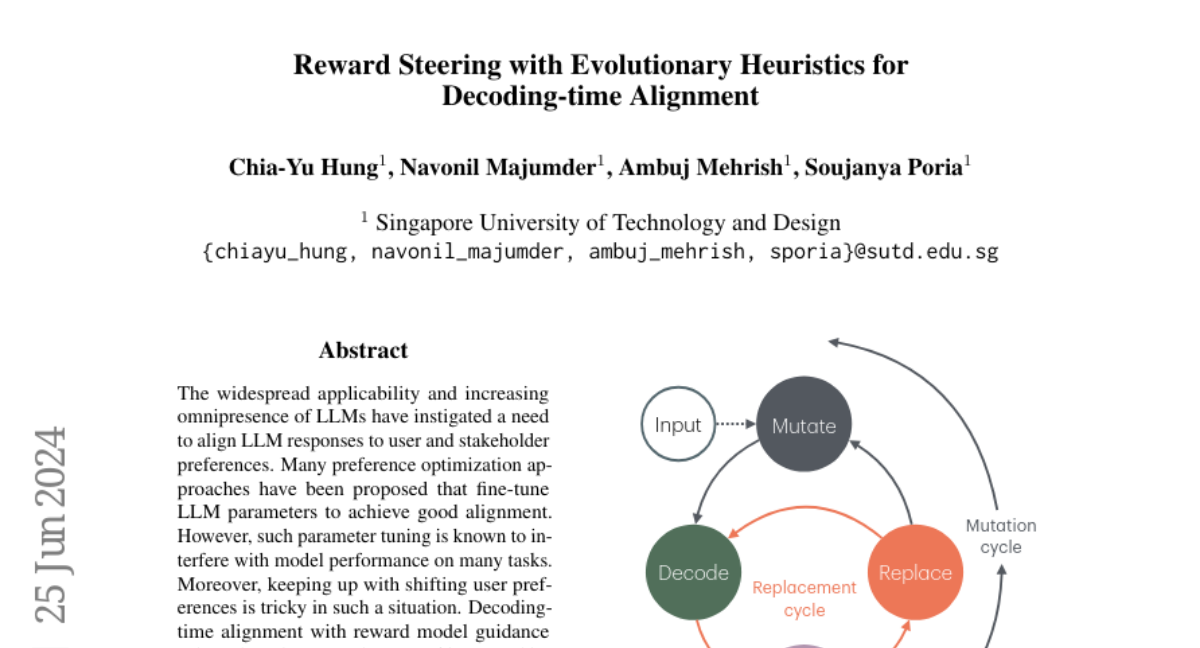
Summary
This paper presents a new method called Reward Steering that uses evolutionary heuristics to improve how large language models (LLMs) align their responses with user preferences during the decoding process.
What's the problem?
As LLMs are used more widely, it's important for them to provide answers that match what users want. Traditional methods to adjust these models often involve fine-tuning their parameters, but this can negatively affect their performance on different tasks. Additionally, user preferences can change over time, making it hard for models to keep up and stay relevant.
What's the solution?
The authors propose a method called decoding-time alignment that uses a reward model to guide the responses of LLMs. They separate the process into two parts: exploration, where the model tries out different variations of instructions, and exploitation, where it replaces less effective responses with better ones based on rewards. By doing this in an evolutionary way, they can improve the quality of the responses while maintaining good performance across tasks. Their experiments show that this approach works better than many existing methods on popular evaluation benchmarks.
Why it matters?
This research is significant because it offers a more effective way to ensure that LLMs produce responses that align with what users want. By improving how these models adapt to user preferences in real-time, Reward Steering can enhance user satisfaction and make AI systems more useful in various applications, from customer service to content generation.
Abstract
The widespread applicability and increasing omnipresence of LLMs have instigated a need to align LLM responses to user and stakeholder preferences. Many preference optimization approaches have been proposed that fine-tune LLM parameters to achieve good alignment. However, such parameter tuning is known to interfere with model performance on many tasks. Moreover, keeping up with shifting user preferences is tricky in such a situation. Decoding-time alignment with reward model guidance solves these issues at the cost of increased inference time. However, most of such methods fail to strike the right balance between exploration and exploitation of reward -- often due to the conflated formulation of these two aspects - to give well-aligned responses. To remedy this we decouple these two aspects and implement them in an evolutionary fashion: exploration is enforced by decoding from mutated instructions and exploitation is represented as the periodic replacement of poorly-rewarded generations with well-rewarded ones. Empirical evidences indicate that this strategy outperforms many preference optimization and decode-time alignment approaches on two widely accepted alignment benchmarks AlpacaEval 2 and MT-Bench. Our implementation will be available at: https://darwin-alignment.github.io.