RM-Bench: Benchmarking Reward Models of Language Models with Subtlety and Style
Yantao Liu, Zijun Yao, Rui Min, Yixin Cao, Lei Hou, Juanzi Li
2024-10-22
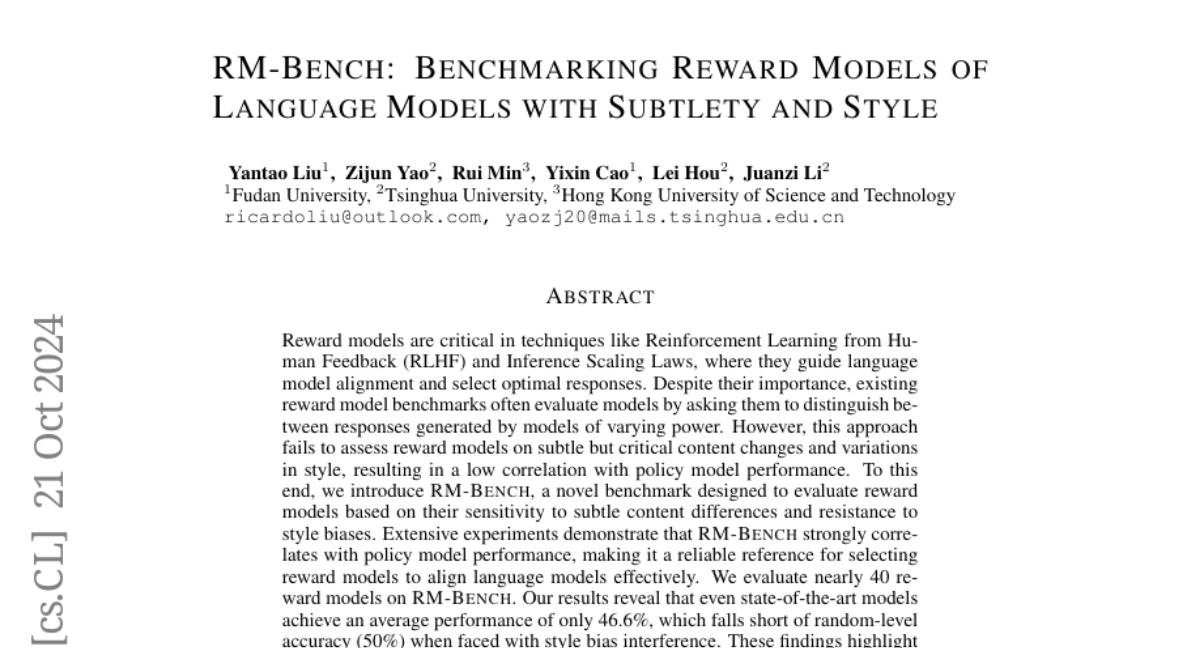
Summary
This paper introduces RM-Bench, a new benchmark designed to evaluate reward models used in language models, focusing on their ability to detect subtle content differences and resist style biases.
What's the problem?
Reward models are essential for aligning language models with human preferences, especially in methods like Reinforcement Learning from Human Feedback (RLHF). However, existing benchmarks often only test these models by comparing their ability to distinguish between responses from different models, which doesn't adequately assess their sensitivity to important content changes or style variations. This can lead to a disconnect between how well reward models perform and how effectively they guide language models.
What's the solution?
To address this issue, the authors developed RM-Bench, which evaluates reward models based on their sensitivity to subtle differences in content and their resistance to biases related to style. They conducted extensive experiments with nearly 40 different reward models and found that RM-Bench correlates well with actual performance in real-world applications. The results showed that even top-performing models struggled under conditions of style bias, achieving only 46.6% accuracy on average, which is below random chance.
Why it matters?
This research is important because it highlights the need for better evaluation methods for reward models, which are crucial for improving AI systems. By providing a more nuanced benchmark like RM-Bench, the authors aim to help researchers identify weaknesses in current reward models and encourage improvements. This can lead to more reliable AI systems that align closely with human values and preferences.
Abstract
Reward models are critical in techniques like Reinforcement Learning from Human Feedback (RLHF) and Inference Scaling Laws, where they guide language model alignment and select optimal responses. Despite their importance, existing reward model benchmarks often evaluate models by asking them to distinguish between responses generated by models of varying power. However, this approach fails to assess reward models on subtle but critical content changes and variations in style, resulting in a low correlation with policy model performance. To this end, we introduce RM-Bench, a novel benchmark designed to evaluate reward models based on their sensitivity to subtle content differences and resistance to style biases. Extensive experiments demonstrate that RM-Bench strongly correlates with policy model performance, making it a reliable reference for selecting reward models to align language models effectively. We evaluate nearly 40 reward models on RM-Bench. Our results reveal that even state-of-the-art models achieve an average performance of only 46.6%, which falls short of random-level accuracy (50%) when faced with style bias interference. These findings highlight the significant room for improvement in current reward models. Related code and data are available at https://github.com/THU-KEG/RM-Bench.