Robin3D: Improving 3D Large Language Model via Robust Instruction Tuning
Weitai Kang, Haifeng Huang, Yuzhang Shang, Mubarak Shah, Yan Yan
2024-10-04
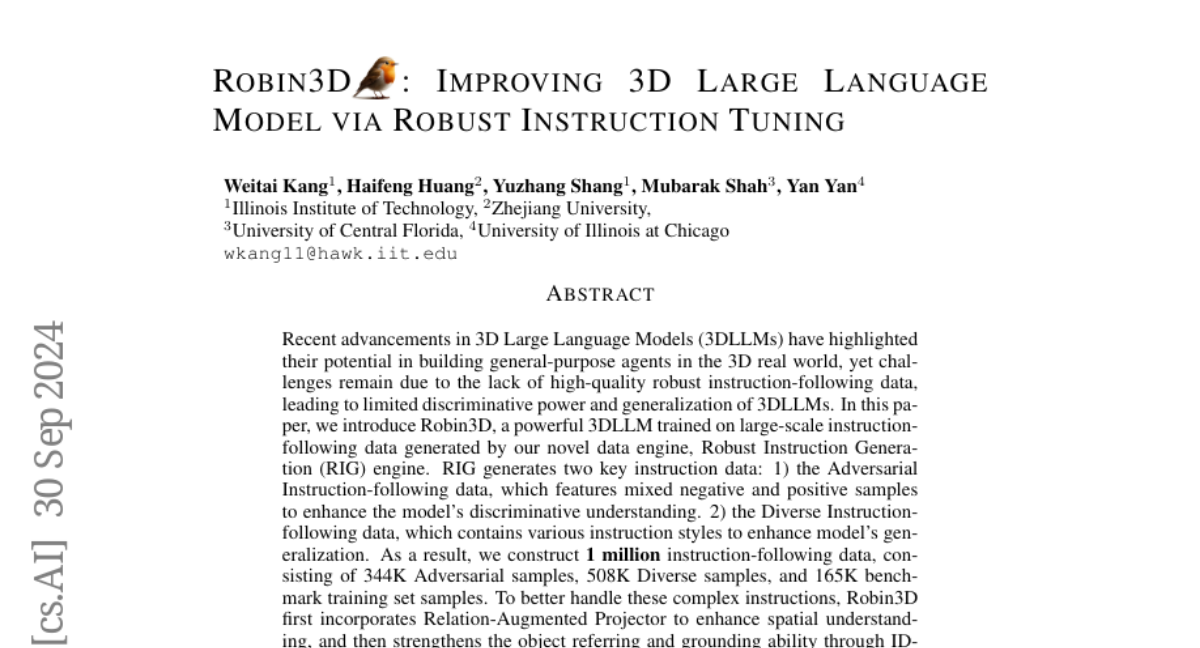
Summary
This paper introduces Robin3D, a new method for improving 3D Large Language Models (3DLLMs) by using robust instruction tuning to enhance their ability to follow complex instructions in various languages.
What's the problem?
3D Large Language Models have great potential for understanding and interacting with the 3D world, but they often struggle due to a lack of high-quality instruction data. This means they don't perform well in tasks that require reasoning and understanding, especially when there isn't enough specific training data available for non-English languages or complex tasks.
What's the solution?
To tackle this issue, the authors developed a data generation engine called Robust Instruction Generation (RIG) that creates two types of instruction data: Adversarial Instruction-following data, which helps the model learn to distinguish between correct and incorrect instructions, and Diverse Instruction-following data, which exposes the model to various ways of giving instructions. They generated a total of one million instruction-following samples and trained Robin3D using these samples. The model also includes techniques like Relation-Augmented Projector and ID-Feature Bonding to improve its understanding of spatial relationships and object recognition.
Why it matters?
This research is significant because it demonstrates how enhancing the training data and methods can lead to better performance in 3DLLMs. By improving how these models understand and follow instructions, Robin3D can be used in applications that require accurate 3D reasoning and interaction, such as robotics, virtual reality, and gaming.
Abstract
Recent advancements in 3D Large Language Models (3DLLMs) have highlighted their potential in building general-purpose agents in the 3D real world, yet challenges remain due to the lack of high-quality robust instruction-following data, leading to limited discriminative power and generalization of 3DLLMs. In this paper, we introduce Robin3D, a powerful 3DLLM trained on large-scale instruction-following data generated by our novel data engine, Robust Instruction Generation (RIG) engine. RIG generates two key instruction data: 1) the Adversarial Instruction-following data, which features mixed negative and positive samples to enhance the model's discriminative understanding. 2) the Diverse Instruction-following data, which contains various instruction styles to enhance model's generalization. As a result, we construct 1 million instruction-following data, consisting of 344K Adversarial samples, 508K Diverse samples, and 165K benchmark training set samples. To better handle these complex instructions, Robin3D first incorporates Relation-Augmented Projector to enhance spatial understanding, and then strengthens the object referring and grounding ability through ID-Feature Bonding. Robin3D consistently outperforms previous methods across five widely-used 3D multimodal learning benchmarks, without the need for task-specific fine-tuning. Notably, we achieve a 7.8\% improvement in the grounding task (Multi3DRefer) and a 6.9\% improvement in the captioning task (Scan2Cap).