Robot See Robot Do: Imitating Articulated Object Manipulation with Monocular 4D Reconstruction
Justin Kerr, Chung Min Kim, Mingxuan Wu, Brent Yi, Qianqian Wang, Ken Goldberg, Angjoo Kanazawa
2024-09-27
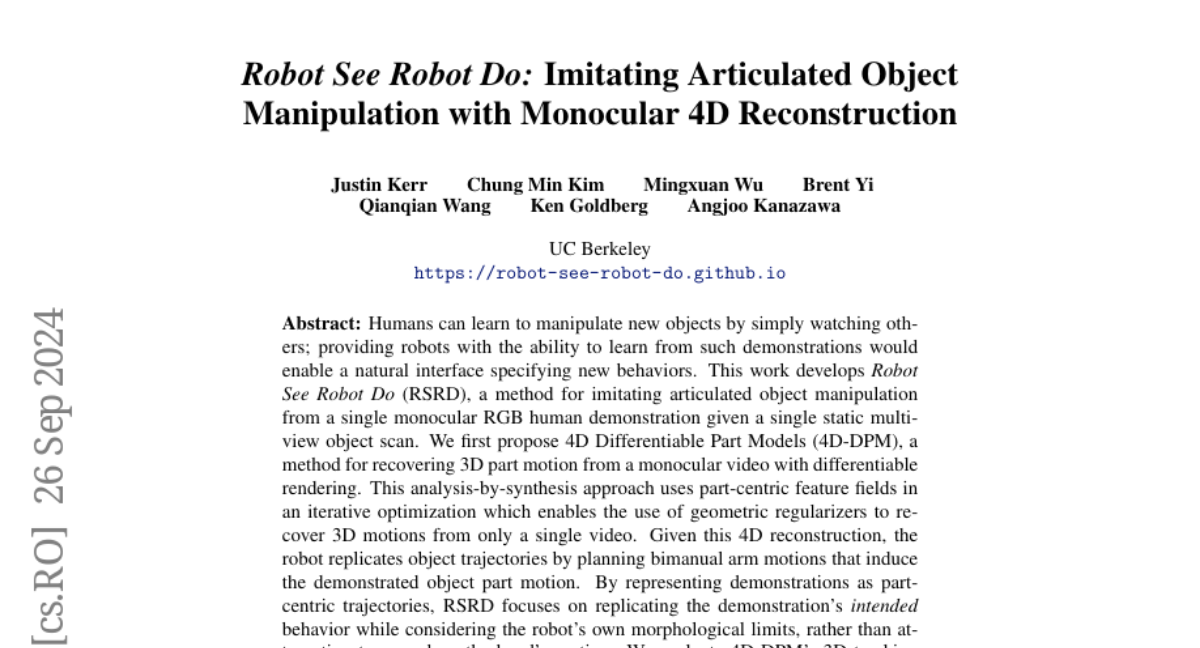
Summary
This paper talks about Robot See Robot Do (RSRD), a method that allows robots to learn how to manipulate objects by watching a single video of a human demonstration. It uses advanced techniques to understand and replicate the movements needed to handle different objects.
What's the problem?
Humans can easily learn how to use new tools or manipulate objects by observing others, but teaching robots to do the same is challenging. Traditional methods require extensive programming for each specific task, which is time-consuming and impractical. Robots need a way to learn from demonstrations in a more natural and efficient manner.
What's the solution?
The researchers developed RSRD, which uses a technique called 4D Differentiable Part Models (4D-DPM) to analyze a video of a human demonstrating how to manipulate an object. This method allows the robot to identify and replicate the movements necessary for handling the object without needing detailed programming. Instead of trying to copy the human's hand movements exactly, RSRD focuses on understanding the intended actions and adapting them to the robot's capabilities. The system was tested on nine different objects, achieving an average success rate of 87% for individual tasks and 60% overall across multiple trials.
Why it matters?
This research is important because it represents a significant step towards making robots more adaptable and capable of learning new tasks quickly by simply observing humans. By reducing the need for extensive programming, RSRD could lead to more efficient robotic systems that can be used in various applications, such as manufacturing, healthcare, and everyday tasks, ultimately making robots more useful in our daily lives.
Abstract
Humans can learn to manipulate new objects by simply watching others; providing robots with the ability to learn from such demonstrations would enable a natural interface specifying new behaviors. This work develops Robot See Robot Do (RSRD), a method for imitating articulated object manipulation from a single monocular RGB human demonstration given a single static multi-view object scan. We first propose 4D Differentiable Part Models (4D-DPM), a method for recovering 3D part motion from a monocular video with differentiable rendering. This analysis-by-synthesis approach uses part-centric feature fields in an iterative optimization which enables the use of geometric regularizers to recover 3D motions from only a single video. Given this 4D reconstruction, the robot replicates object trajectories by planning bimanual arm motions that induce the demonstrated object part motion. By representing demonstrations as part-centric trajectories, RSRD focuses on replicating the demonstration's intended behavior while considering the robot's own morphological limits, rather than attempting to reproduce the hand's motion. We evaluate 4D-DPM's 3D tracking accuracy on ground truth annotated 3D part trajectories and RSRD's physical execution performance on 9 objects across 10 trials each on a bimanual YuMi robot. Each phase of RSRD achieves an average of 87% success rate, for a total end-to-end success rate of 60% across 90 trials. Notably, this is accomplished using only feature fields distilled from large pretrained vision models -- without any task-specific training, fine-tuning, dataset collection, or annotation. Project page: https://robot-see-robot-do.github.io