ROCKET-1: Master Open-World Interaction with Visual-Temporal Context Prompting
Shaofei Cai, Zihao Wang, Kewei Lian, Zhancun Mu, Xiaojian Ma, Anji Liu, Yitao Liang
2024-10-28
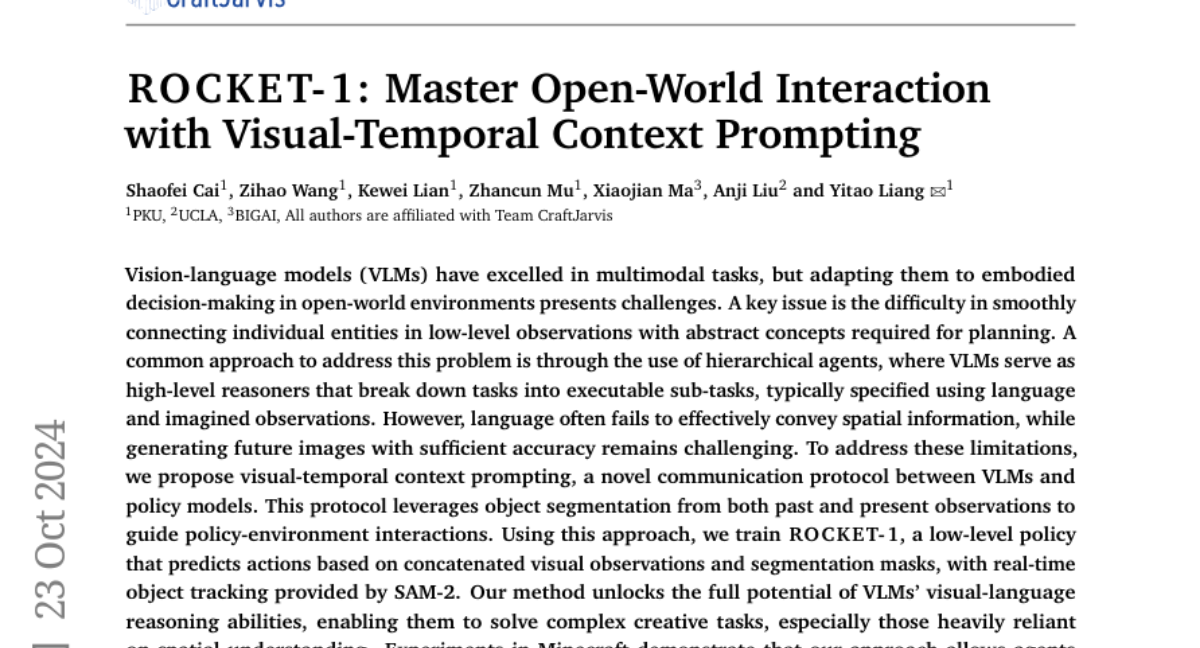
Summary
This paper introduces ROCKET-1, a new method that helps AI agents interact better in open-world environments by using visual and temporal information to enhance decision-making.
What's the problem?
AI models that understand both images and language (called vision-language models) often struggle when placed in complex environments where they need to make decisions based on what they see and the tasks they need to perform. A major challenge is connecting detailed observations of objects with the abstract concepts required for planning actions. Traditional methods often fail because they can’t effectively communicate spatial information or predict future scenarios accurately.
What's the solution?
To solve these issues, the authors propose a new communication method called visual-temporal context prompting. This approach allows AI agents to use object segmentation from both past and present observations, helping them make better decisions in their environment. They trained ROCKET-1, a low-level policy model, to predict actions based on combined visual data and segmentation masks, with real-time tracking of objects. Experiments conducted in Minecraft showed that this method enabled AI agents to complete tasks that were previously impossible.
Why it matters?
This research is important because it enhances how AI can operate in dynamic, unpredictable environments. By improving the way AI understands and interacts with its surroundings, ROCKET-1 could lead to more advanced applications in robotics, gaming, and other fields where intelligent decision-making is crucial.
Abstract
Vision-language models (VLMs) have excelled in multimodal tasks, but adapting them to embodied decision-making in open-world environments presents challenges. A key issue is the difficulty in smoothly connecting individual entities in low-level observations with abstract concepts required for planning. A common approach to address this problem is through the use of hierarchical agents, where VLMs serve as high-level reasoners that break down tasks into executable sub-tasks, typically specified using language and imagined observations. However, language often fails to effectively convey spatial information, while generating future images with sufficient accuracy remains challenging. To address these limitations, we propose visual-temporal context prompting, a novel communication protocol between VLMs and policy models. This protocol leverages object segmentation from both past and present observations to guide policy-environment interactions. Using this approach, we train ROCKET-1, a low-level policy that predicts actions based on concatenated visual observations and segmentation masks, with real-time object tracking provided by SAM-2. Our method unlocks the full potential of VLMs visual-language reasoning abilities, enabling them to solve complex creative tasks, especially those heavily reliant on spatial understanding. Experiments in Minecraft demonstrate that our approach allows agents to accomplish previously unattainable tasks, highlighting the effectiveness of visual-temporal context prompting in embodied decision-making. Codes and demos will be available on the project page: https://craftjarvis.github.io/ROCKET-1.