RoundTable: Leveraging Dynamic Schema and Contextual Autocomplete for Enhanced Query Precision in Tabular Question Answering
Pratyush Kumar, Kuber Vijaykumar Bellad, Bharat Vadlamudi, Aman Chadha
2024-08-26
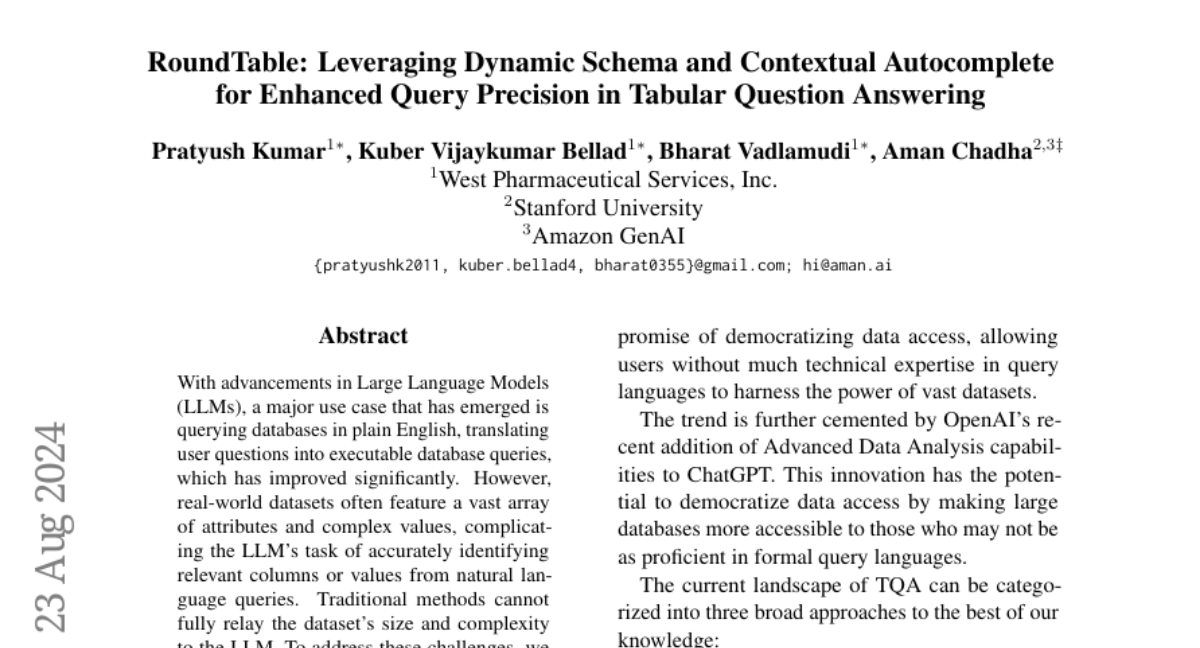
Summary
This paper presents RoundTable, a new system that improves how people can ask questions about data tables using natural language, making it easier to get accurate answers.
What's the problem?
Asking questions in plain English to get information from complex databases can be difficult because these databases often have many different attributes and complicated values. Current systems struggle to understand user queries accurately, especially when the data is large and complex.
What's the solution?
The authors propose a framework that uses Full-Text Search (FTS) to improve how the system identifies relevant columns and values in a database. This allows for more precise detection of specific data points and makes the search space smaller, which helps the language model provide better answers. Additionally, RoundTable includes a custom autocomplete feature that suggests possible queries based on the data in the table, enhancing user interaction.
Why it matters?
This research is important because it simplifies the process of querying complex datasets, allowing more people to access and utilize data effectively without needing technical expertise. By improving how users interact with databases, RoundTable can enhance decision-making in various fields such as business, research, and education.
Abstract
With advancements in Large Language Models (LLMs), a major use case that has emerged is querying databases in plain English, translating user questions into executable database queries, which has improved significantly. However, real-world datasets often feature a vast array of attributes and complex values, complicating the LLMs task of accurately identifying relevant columns or values from natural language queries. Traditional methods cannot fully relay the datasets size and complexity to the LLM. To address these challenges, we propose a novel framework that leverages Full-Text Search (FTS) on the input table. This approach not only enables precise detection of specific values and columns but also narrows the search space for language models, thereby enhancing query accuracy. Additionally, it supports a custom auto-complete feature that suggests queries based on the data in the table. This integration significantly refines the interaction between the user and complex datasets, offering a sophisticated solution to the limitations faced by current table querying capabilities. This work is accompanied by an application for both Mac and Windows platforms, which readers can try out themselves on their own data.