RP1M: A Large-Scale Motion Dataset for Piano Playing with Bi-Manual Dexterous Robot Hands
Yi Zhao, Le Chen, Jan Schneider, Quankai Gao, Juho Kannala, Bernhard Schölkopf, Joni Pajarinen, Dieter Büchler
2024-08-21
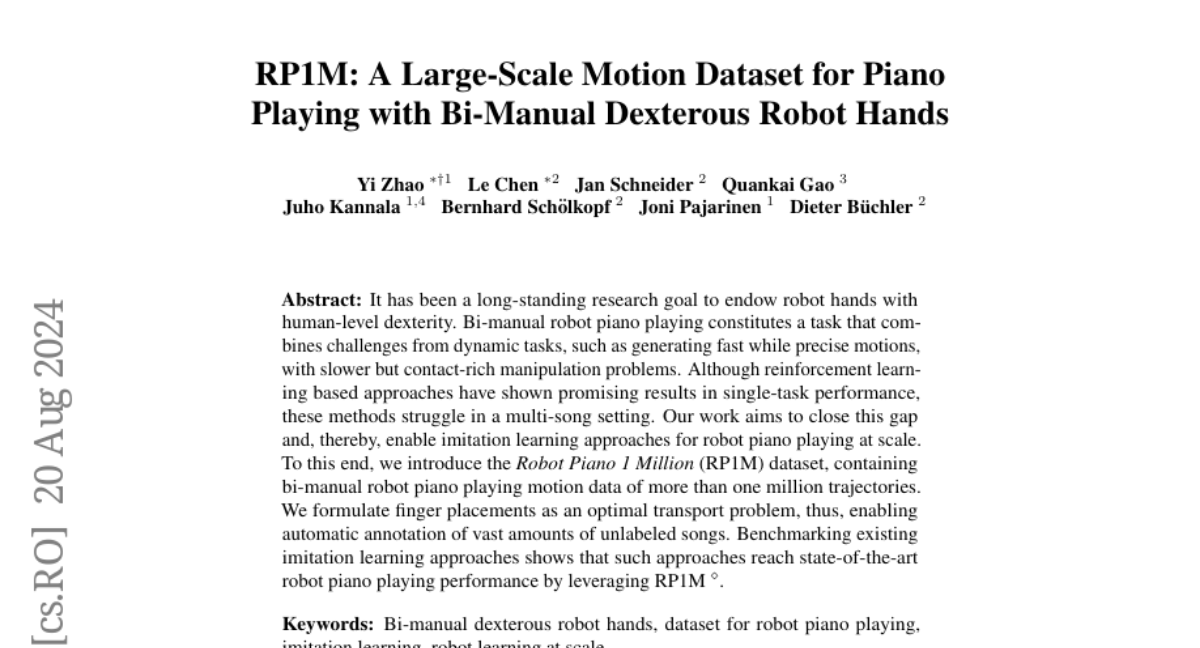
Summary
This paper introduces the Robot Piano 1 Million (RP1M) dataset, which contains a large amount of motion data for robot hands playing the piano, aimed at improving how robots can learn to play music like humans.
What's the problem?
Teaching robots to play the piano with human-like dexterity is difficult because existing methods often work well for single tasks but struggle when trying to play multiple songs. This limits the ability of robots to learn and perform complex musical pieces effectively.
What's the solution?
The authors created the RP1M dataset, which includes over one million motion trajectories of robot hands playing the piano. They developed a method to automatically determine where the robot's fingers should go on the keys, allowing the robot to learn from a wide variety of songs without needing human input for each piece. This dataset enables more effective training of robots to play multiple songs through imitation learning.
Why it matters?
This research is important because it provides a comprehensive resource that can help advance the field of robotics by enabling robots to learn and perform music at scale. By improving how robots can imitate human piano playing, this work could lead to better robotic musicians and applications in entertainment, education, and therapy.
Abstract
It has been a long-standing research goal to endow robot hands with human-level dexterity. Bi-manual robot piano playing constitutes a task that combines challenges from dynamic tasks, such as generating fast while precise motions, with slower but contact-rich manipulation problems. Although reinforcement learning based approaches have shown promising results in single-task performance, these methods struggle in a multi-song setting. Our work aims to close this gap and, thereby, enable imitation learning approaches for robot piano playing at scale. To this end, we introduce the Robot Piano 1 Million (RP1M) dataset, containing bi-manual robot piano playing motion data of more than one million trajectories. We formulate finger placements as an optimal transport problem, thus, enabling automatic annotation of vast amounts of unlabeled songs. Benchmarking existing imitation learning approaches shows that such approaches reach state-of-the-art robot piano playing performance by leveraging RP1M.