SALOVA: Segment-Augmented Long Video Assistant for Targeted Retrieval and Routing in Long-Form Video Analysis
Junho Kim, Hyunjun Kim, Hosu Lee, Yong Man Ro
2024-11-27
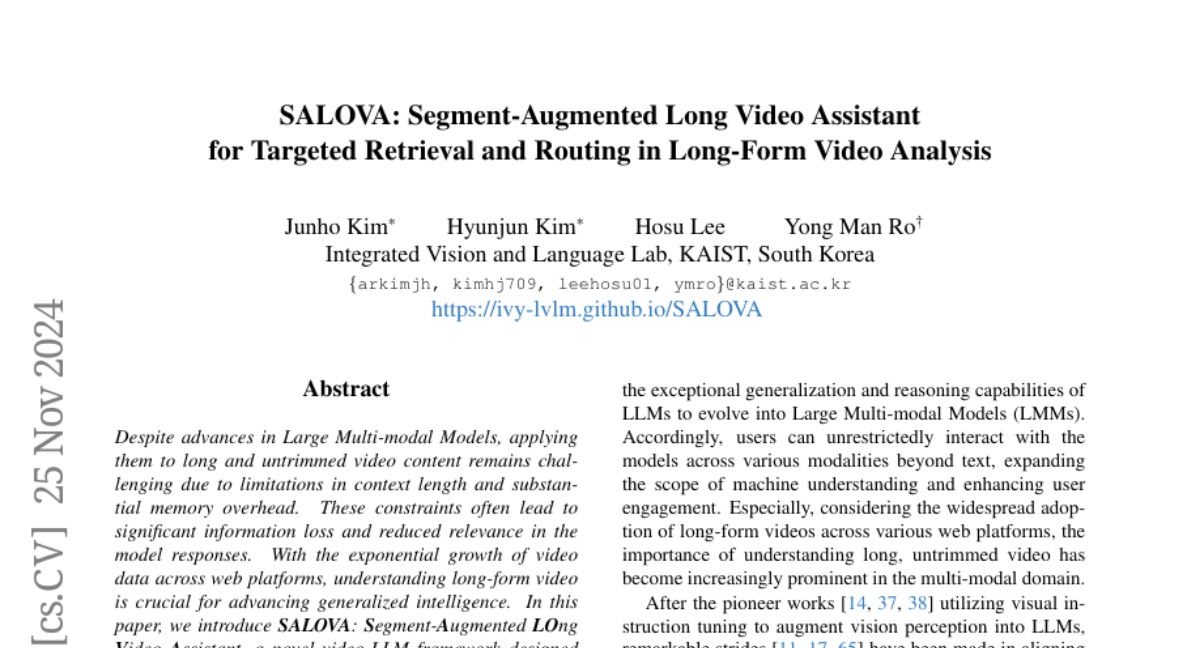
Summary
This paper introduces SALOVA, a new tool designed to help computers better understand long videos by breaking them down into smaller, more manageable segments. It addresses the challenges of processing lengthy video content effectively.
What's the problem?
Long videos can be difficult for computers to analyze because they contain a lot of information, which can overwhelm the system's memory and lead to important details being missed. As more videos are shared online, finding ways to understand these lengthy formats is becoming increasingly important.
What's the solution?
The authors created SALOVA, which uses a special dataset called SceneWalk that includes nearly 88,000 long videos with detailed descriptions for each segment. This allows the computer to focus on relevant parts of the video based on user questions. SALOVA also features advanced design elements that help it efficiently retrieve and process these segments, ensuring that the context of the video is preserved and understood better.
Why it matters?
Understanding long-form videos is crucial for many applications, such as improving search engines, enhancing video recommendations, and developing better AI systems. SALOVA's ability to maintain context and relevance in video analysis represents a significant step forward in making sense of the vast amounts of video data available today.
Abstract
Despite advances in Large Multi-modal Models, applying them to long and untrimmed video content remains challenging due to limitations in context length and substantial memory overhead. These constraints often lead to significant information loss and reduced relevance in the model responses. With the exponential growth of video data across web platforms, understanding long-form video is crucial for advancing generalized intelligence. In this paper, we introduce SALOVA: Segment-Augmented LOng Video Assistant, a novel video-LLM framework designed to enhance the comprehension of lengthy video content through targeted retrieval process. We address two main challenges to achieve it: (i) We present the SceneWalk dataset, a high-quality collection of 87.8K long videos, each densely captioned at the segment level to enable models to capture scene continuity and maintain rich descriptive context. (ii) We develop robust architectural designs integrating dynamic routing mechanism and spatio-temporal projector to efficiently retrieve and process relevant video segments based on user queries. Our framework mitigates the limitations of current video-LMMs by allowing for precise identification and retrieval of relevant video segments in response to queries, thereby improving the contextual relevance of the generated responses. Through extensive experiments, SALOVA demonstrates enhanced capability in processing complex long-form videos, showing significant capability to maintain contextual integrity across extended sequences.