SAM2Long: Enhancing SAM 2 for Long Video Segmentation with a Training-Free Memory Tree
Shuangrui Ding, Rui Qian, Xiaoyi Dong, Pan Zhang, Yuhang Zang, Yuhang Cao, Yuwei Guo, Dahua Lin, Jiaqi Wang
2024-10-22
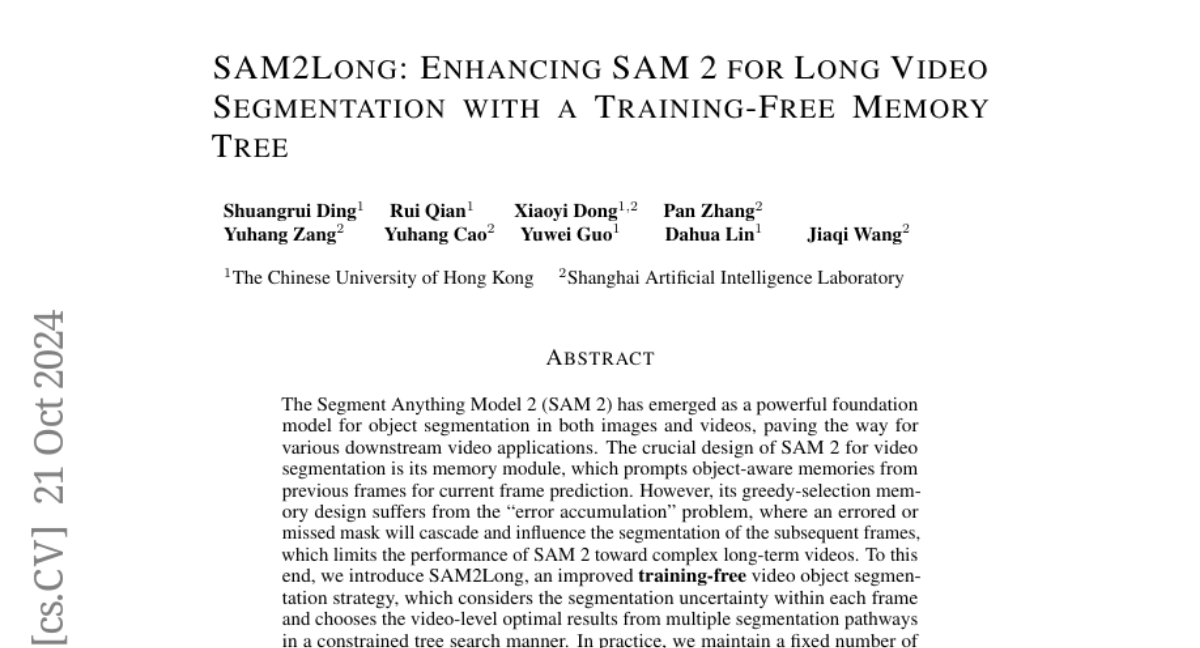
Summary
This paper presents SAM2Long, an enhanced version of the Segment Anything Model 2 (SAM 2) that improves how it segments and tracks objects in long videos without needing additional training.
What's the problem?
While SAM 2 is effective for segmenting objects in images and videos, it struggles with long videos due to a problem called 'error accumulation.' This occurs when an incorrect segmentation mask affects the results of subsequent frames, leading to mistakes that can compound over time. As a result, SAM 2 may fail to accurately track objects, especially when they are occluded (blocked from view) or reappear after being hidden.
What's the solution?
To solve this issue, the authors developed SAM2Long, which uses a new method that creates multiple potential segmentation paths for each frame in a video. Instead of relying on just one mask for each frame, SAM2Long proposes several masks and evaluates them based on their performance. It then selects the best-performing masks to use for the next frame. This approach helps reduce errors and improves the model's ability to handle complex scenes with occlusions and object reappearances. Importantly, SAM2Long does all this without requiring additional training or parameters.
Why it matters?
This research is significant because it enhances the capabilities of video segmentation models, making them more reliable for real-world applications like surveillance, autonomous driving, and video editing. By improving how models track and segment objects over long periods, SAM2Long can lead to better performance in scenarios where accuracy is crucial.
Abstract
The Segment Anything Model 2 (SAM 2) has emerged as a powerful foundation model for object segmentation in both images and videos, paving the way for various downstream video applications. The crucial design of SAM 2 for video segmentation is its memory module, which prompts object-aware memories from previous frames for current frame prediction. However, its greedy-selection memory design suffers from the "error accumulation" problem, where an errored or missed mask will cascade and influence the segmentation of the subsequent frames, which limits the performance of SAM 2 toward complex long-term videos. To this end, we introduce SAM2Long, an improved training-free video object segmentation strategy, which considers the segmentation uncertainty within each frame and chooses the video-level optimal results from multiple segmentation pathways in a constrained tree search manner. In practice, we maintain a fixed number of segmentation pathways throughout the video. For each frame, multiple masks are proposed based on the existing pathways, creating various candidate branches. We then select the same fixed number of branches with higher cumulative scores as the new pathways for the next frame. After processing the final frame, the pathway with the highest cumulative score is chosen as the final segmentation result. Benefiting from its heuristic search design, SAM2Long is robust toward occlusions and object reappearances, and can effectively segment and track objects for complex long-term videos. Notably, SAM2Long achieves an average improvement of 3.0 points across all 24 head-to-head comparisons, with gains of up to 5.3 points in J&F on long-term video object segmentation benchmarks such as SA-V and LVOS. The code is released at https://github.com/Mark12Ding/SAM2Long.