SAM2Point: Segment Any 3D as Videos in Zero-shot and Promptable Manners
Ziyu Guo, Renrui Zhang, Xiangyang Zhu, Chengzhuo Tong, Peng Gao, Chunyuan Li, Pheng-Ann Heng
2024-08-30
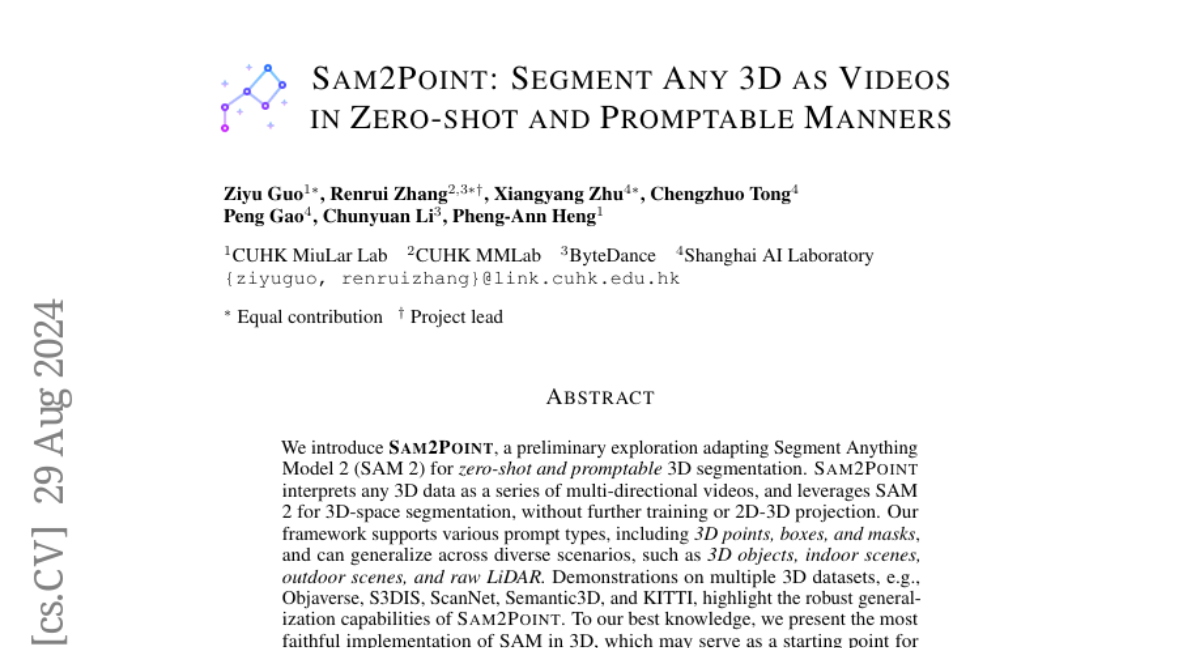
Summary
This paper introduces SAM2Point, a new method that allows for easy segmentation of 3D objects from various views using the Segment Anything Model 2 (SAM 2) without needing extra training.
What's the problem?
Segmenting 3D objects accurately from limited views can be difficult. Traditional methods often require many images or specific training to understand how to separate different parts of a scene, which can be time-consuming and complex.
What's the solution?
SAM2Point tackles this problem by treating 3D data as a series of videos that can be viewed from different angles. It uses the capabilities of SAM 2 to segment the 3D space based on prompts like points, boxes, or masks. This means it can understand and separate objects in various settings, such as indoor or outdoor scenes, without needing additional training or converting 2D images into 3D models.
Why it matters?
This research is significant because it simplifies the process of working with 3D data, making it more accessible for applications in fields like virtual reality, gaming, and robotics. By allowing users to segment objects easily from just a few views, it opens up new possibilities for creating detailed and interactive 3D environments.
Abstract
We introduce SAM2Point, a preliminary exploration adapting Segment Anything Model 2 (SAM 2) for zero-shot and promptable 3D segmentation. SAM2Point interprets any 3D data as a series of multi-directional videos, and leverages SAM 2 for 3D-space segmentation, without further training or 2D-3D projection. Our framework supports various prompt types, including 3D points, boxes, and masks, and can generalize across diverse scenarios, such as 3D objects, indoor scenes, outdoor environments, and raw sparse LiDAR. Demonstrations on multiple 3D datasets, e.g., Objaverse, S3DIS, ScanNet, Semantic3D, and KITTI, highlight the robust generalization capabilities of SAM2Point. To our best knowledge, we present the most faithful implementation of SAM in 3D, which may serve as a starting point for future research in promptable 3D segmentation. Online Demo: https://huggingface.co/spaces/ZiyuG/SAM2Point . Code: https://github.com/ZiyuGuo99/SAM2Point .