SAMURAI: Adapting Segment Anything Model for Zero-Shot Visual Tracking with Motion-Aware Memory
Cheng-Yen Yang, Hsiang-Wei Huang, Wenhao Chai, Zhongyu Jiang, Jenq-Neng Hwang
2024-11-21
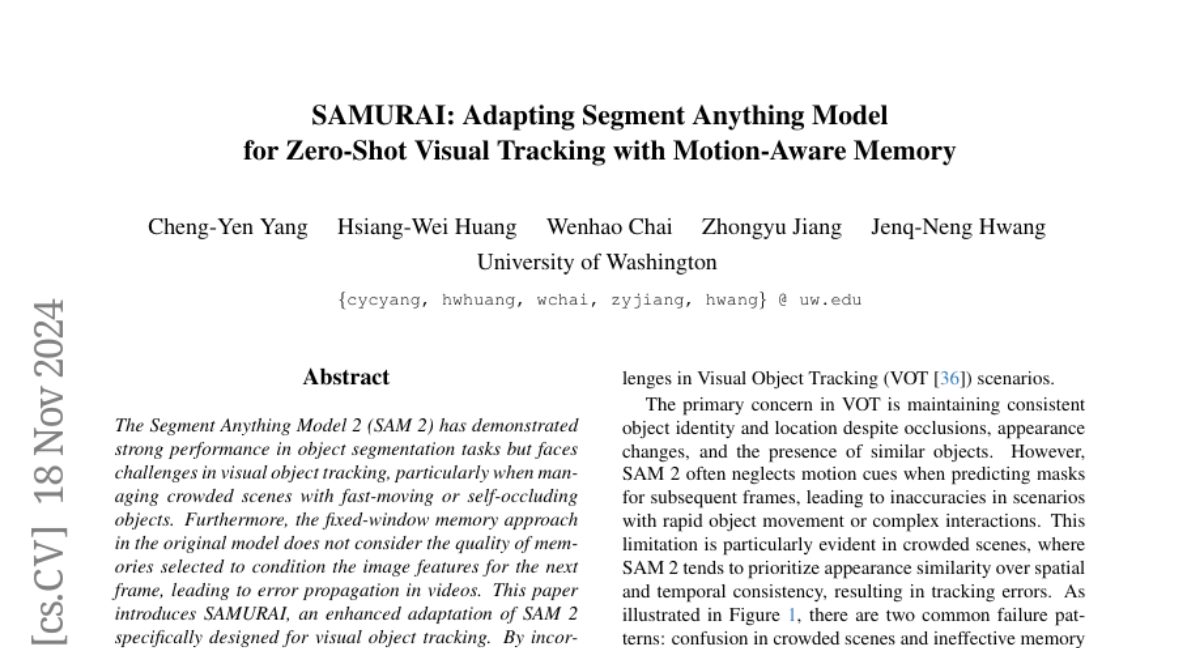
Summary
This paper discusses SAMURAI, a new method that enhances the Segment Anything Model (SAM 2) to improve visual object tracking in videos, especially in crowded or complex scenes.
What's the problem?
Tracking objects in videos can be very challenging due to issues like occlusion (when objects block each other), fast movements, and similar-looking objects in crowded environments. The original SAM 2 model struggled with these problems because its memory system didn't effectively manage the information needed to keep track of objects over time.
What's the solution?
SAMURAI addresses these challenges by introducing a motion-aware memory system that helps predict where an object will move next. It uses two main strategies: incorporating motion cues to enhance mask selection (which helps identify objects) and selecting the best frames from memory based on their quality. This allows SAMURAI to track objects accurately without needing to be retrained for each new scenario, achieving strong performance across various benchmarks.
Why it matters?
This research is important because it allows for better tracking of objects in real-time applications, such as surveillance and robotics, without needing extensive training. By improving how models handle complex scenes, SAMURAI can enhance the capabilities of AI systems in dynamic environments, making them more effective and reliable.
Abstract
The Segment Anything Model 2 (SAM 2) has demonstrated strong performance in object segmentation tasks but faces challenges in visual object tracking, particularly when managing crowded scenes with fast-moving or self-occluding objects. Furthermore, the fixed-window memory approach in the original model does not consider the quality of memories selected to condition the image features for the next frame, leading to error propagation in videos. This paper introduces SAMURAI, an enhanced adaptation of SAM 2 specifically designed for visual object tracking. By incorporating temporal motion cues with the proposed motion-aware memory selection mechanism, SAMURAI effectively predicts object motion and refines mask selection, achieving robust, accurate tracking without the need for retraining or fine-tuning. SAMURAI operates in real-time and demonstrates strong zero-shot performance across diverse benchmark datasets, showcasing its ability to generalize without fine-tuning. In evaluations, SAMURAI achieves significant improvements in success rate and precision over existing trackers, with a 7.1% AUC gain on LaSOT_{ext} and a 3.5% AO gain on GOT-10k. Moreover, it achieves competitive results compared to fully supervised methods on LaSOT, underscoring its robustness in complex tracking scenarios and its potential for real-world applications in dynamic environments. Code and results are available at https://github.com/yangchris11/samurai.