SANA-Sprint: One-Step Diffusion with Continuous-Time Consistency Distillation
Junsong Chen, Shuchen Xue, Yuyang Zhao, Jincheng Yu, Sayak Paul, Junyu Chen, Han Cai, Enze Xie, Song Han
2025-03-14
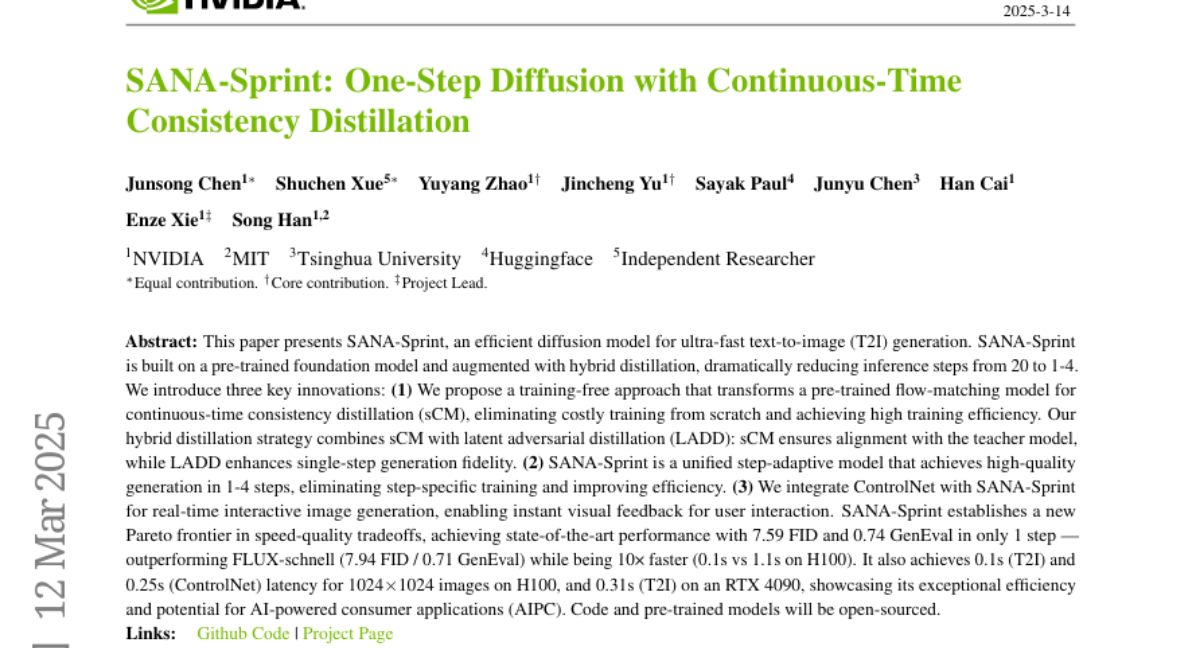
Summary
This paper talks about SANA-Sprint, an AI tool that creates images from text descriptions super fast, needing just 1-4 steps instead of the usual 20, while keeping the pictures high-quality.
What's the problem?
Current AI image generators take too many steps to make pictures, which slows them down and makes them impractical for real-time uses like instant editing.
What's the solution?
SANA-Sprint uses a mix of two smart techniques (sCM and LADD) to simplify the image-making process and works with ControlNet to let users see changes instantly as they type.
Why it matters?
This speeds up AI art tools for things like graphic design apps or social media content, making them responsive enough for live editing on phones or laptops.
Abstract
This paper presents SANA-Sprint, an efficient diffusion model for ultra-fast text-to-image (T2I) generation. SANA-Sprint is built on a pre-trained foundation model and augmented with hybrid distillation, dramatically reducing inference steps from 20 to 1-4. We introduce three key innovations: (1) We propose a training-free approach that transforms a pre-trained flow-matching model for continuous-time consistency distillation (sCM), eliminating costly training from scratch and achieving high training efficiency. Our hybrid distillation strategy combines sCM with latent adversarial distillation (LADD): sCM ensures alignment with the teacher model, while LADD enhances single-step generation fidelity. (2) SANA-Sprint is a unified step-adaptive model that achieves high-quality generation in 1-4 steps, eliminating step-specific training and improving efficiency. (3) We integrate ControlNet with SANA-Sprint for real-time interactive image generation, enabling instant visual feedback for user interaction. SANA-Sprint establishes a new Pareto frontier in speed-quality tradeoffs, achieving state-of-the-art performance with 7.59 FID and 0.74 GenEval in only 1 step - outperforming FLUX-schnell (7.94 FID / 0.71 GenEval) while being 10x faster (0.1s vs 1.1s on H100). It also achieves 0.1s (T2I) and 0.25s (ControlNet) latency for 1024 x 1024 images on H100, and 0.31s (T2I) on an RTX 4090, showcasing its exceptional efficiency and potential for AI-powered consumer applications (AIPC). Code and pre-trained models will be open-sourced.