SaRA: High-Efficient Diffusion Model Fine-tuning with Progressive Sparse Low-Rank Adaptation
Teng Hu, Jiangning Zhang, Ran Yi, Hongrui Huang, Yabiao Wang, Lizhuang Ma
2024-09-11
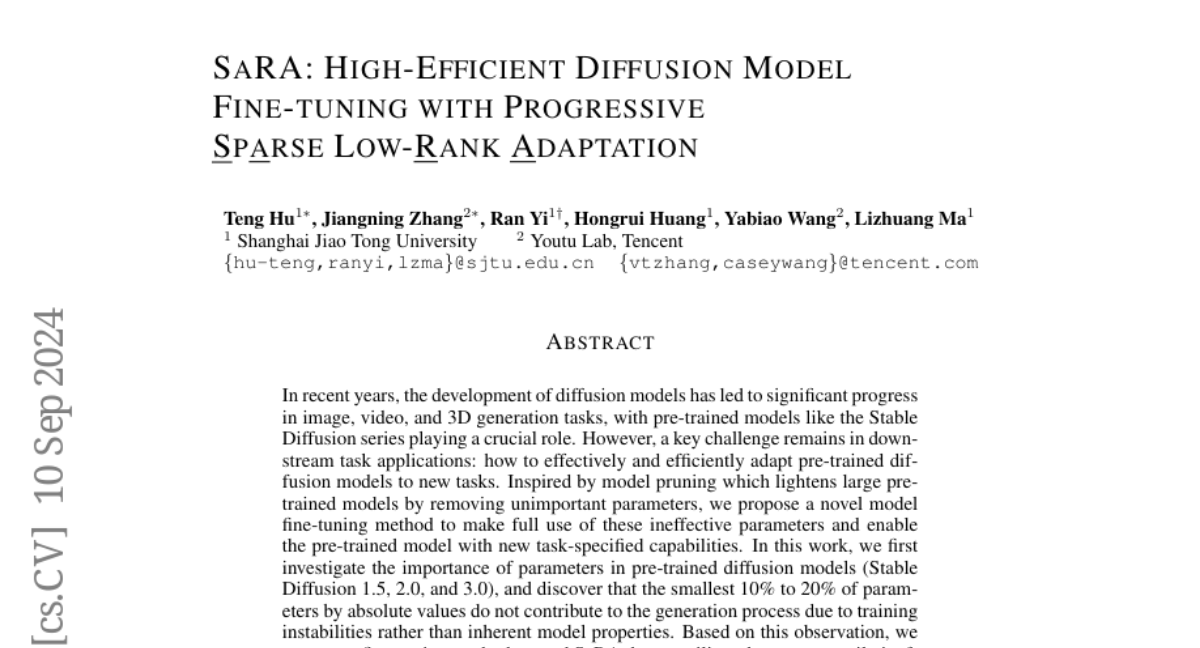
Summary
This paper talks about SaRA, a new method for fine-tuning diffusion models that improves their efficiency and performance by reusing less important parameters.
What's the problem?
Diffusion models, which are used for generating images and videos, often require a lot of resources to fine-tune for specific tasks. Many existing methods do not effectively use all the parameters in these models, leading to inefficiencies and wasted potential. Additionally, fine-tuning can sometimes lead to overfitting, where the model performs well on training data but poorly on new data.
What's the solution?
The authors propose SaRA, which focuses on reusing the least important parameters from pre-trained diffusion models instead of discarding them. They discovered that 10% to 20% of these parameters do not significantly contribute to the generation process. SaRA uses a specialized training strategy that optimizes these parameters while minimizing overfitting. It also includes a new way to adjust parameters progressively during training and a unique backpropagation method that reduces memory usage. Their experiments showed that SaRA improves the generative capabilities of these models compared to traditional methods.
Why it matters?
This research is important because it makes fine-tuning diffusion models more efficient and effective, allowing them to perform better on specific tasks without needing as much computing power. This could lead to advancements in various applications like image and video generation, making these technologies more accessible and practical for real-world use.
Abstract
In recent years, the development of diffusion models has led to significant progress in image and video generation tasks, with pre-trained models like the Stable Diffusion series playing a crucial role. Inspired by model pruning which lightens large pre-trained models by removing unimportant parameters, we propose a novel model fine-tuning method to make full use of these ineffective parameters and enable the pre-trained model with new task-specified capabilities. In this work, we first investigate the importance of parameters in pre-trained diffusion models, and discover that the smallest 10% to 20% of parameters by absolute values do not contribute to the generation process. Based on this observation, we propose a method termed SaRA that re-utilizes these temporarily ineffective parameters, equating to optimizing a sparse weight matrix to learn the task-specific knowledge. To mitigate overfitting, we propose a nuclear-norm-based low-rank sparse training scheme for efficient fine-tuning. Furthermore, we design a new progressive parameter adjustment strategy to make full use of the re-trained/finetuned parameters. Finally, we propose a novel unstructural backpropagation strategy, which significantly reduces memory costs during fine-tuning. Our method enhances the generative capabilities of pre-trained models in downstream applications and outperforms traditional fine-tuning methods like LoRA in maintaining model's generalization ability. We validate our approach through fine-tuning experiments on SD models, demonstrating significant improvements. SaRA also offers a practical advantage that requires only a single line of code modification for efficient implementation and is seamlessly compatible with existing methods.