SaulLM-54B & SaulLM-141B: Scaling Up Domain Adaptation for the Legal Domain
Pierre Colombo, Telmo Pires, Malik Boudiaf, Rui Melo, Dominic Culver, Sofia Morgado, Etienne Malaboeuf, Gabriel Hautreux, Johanne Charpentier, Michael Desa
2024-07-30
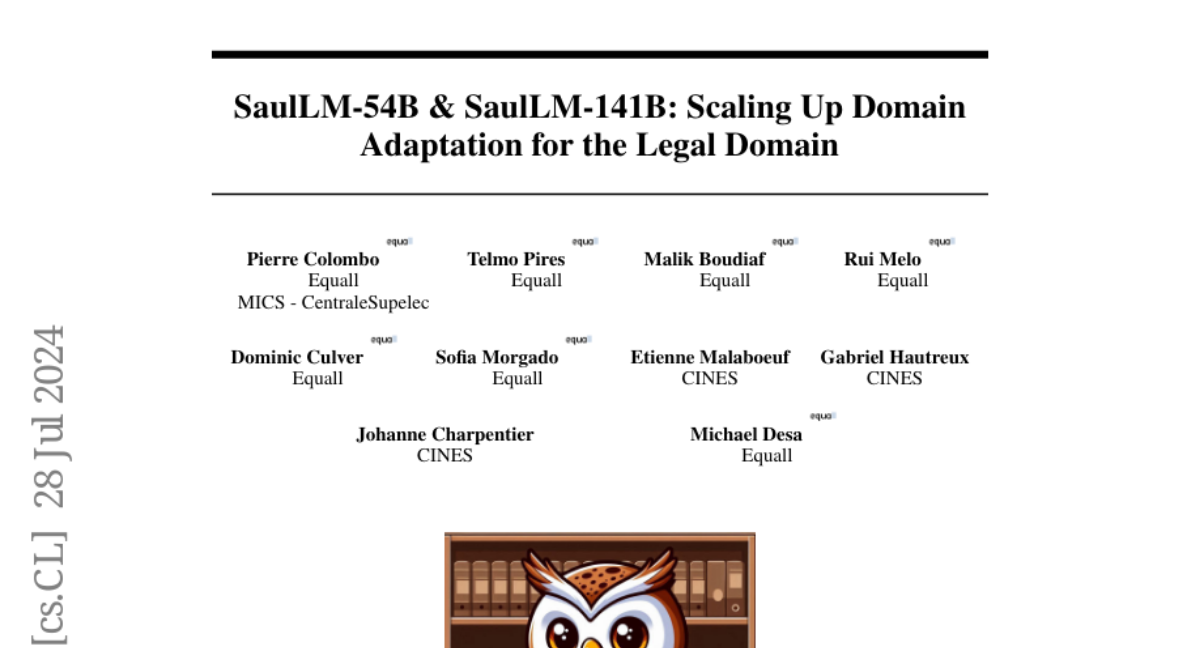
Summary
This paper discusses SaulLM-54B and SaulLM-141B, two advanced language models specifically designed for the legal field. These models are built to better understand and process legal texts by using a large amount of legal data and specialized training techniques.
What's the problem?
Understanding and processing legal documents can be very challenging due to the complex language and specific terminology used in the legal field. Traditional language models often struggle with this because they are not specifically trained on legal texts, which can lead to inaccuracies and misunderstandings in legal applications.
What's the solution?
To solve this problem, the authors developed two large language models, SaulLM-54B and SaulLM-141B, which contain 54 billion and 141 billion parameters, respectively. They used a method called domain adaptation, which involves three main strategies: continuing to train the models with over 540 billion tokens of legal text, implementing a special protocol for following legal instructions, and aligning the model's outputs with human preferences in interpreting legal information. This approach helps the models generate more accurate and relevant responses when dealing with legal texts.
Why it matters?
This research is significant because it enhances the ability of AI systems to work effectively in the legal domain, making them more useful for tasks like legal research, contract analysis, and case summarization. By improving how machines understand legal language, these models can help lawyers and other professionals save time and reduce errors in their work, ultimately making legal services more accessible.
Abstract
In this paper, we introduce SaulLM-54B and SaulLM-141B, two large language models (LLMs) tailored for the legal sector. These models, which feature architectures of 54 billion and 141 billion parameters, respectively, are based on the Mixtral architecture. The development of SaulLM-54B and SaulLM-141B is guided by large-scale domain adaptation, divided into three strategies: (1) the exploitation of continued pretraining involving a base corpus that includes over 540 billion of legal tokens, (2) the implementation of a specialized legal instruction-following protocol, and (3) the alignment of model outputs with human preferences in legal interpretations. The integration of synthetically generated data in the second and third steps enhances the models' capabilities in interpreting and processing legal texts, effectively reaching state-of-the-art performance and outperforming previous open-source models on LegalBench-Instruct. This work explores the trade-offs involved in domain-specific adaptation at this scale, offering insights that may inform future studies on domain adaptation using strong decoder models. Building upon SaulLM-7B, this study refines the approach to produce an LLM better equipped for legal tasks. We are releasing base, instruct, and aligned versions on top of SaulLM-54B and SaulLM-141B under the MIT License to facilitate reuse and collaborative research.