Scalable Ranked Preference Optimization for Text-to-Image Generation
Shyamgopal Karthik, Huseyin Coskun, Zeynep Akata, Sergey Tulyakov, Jian Ren, Anil Kag
2024-10-24
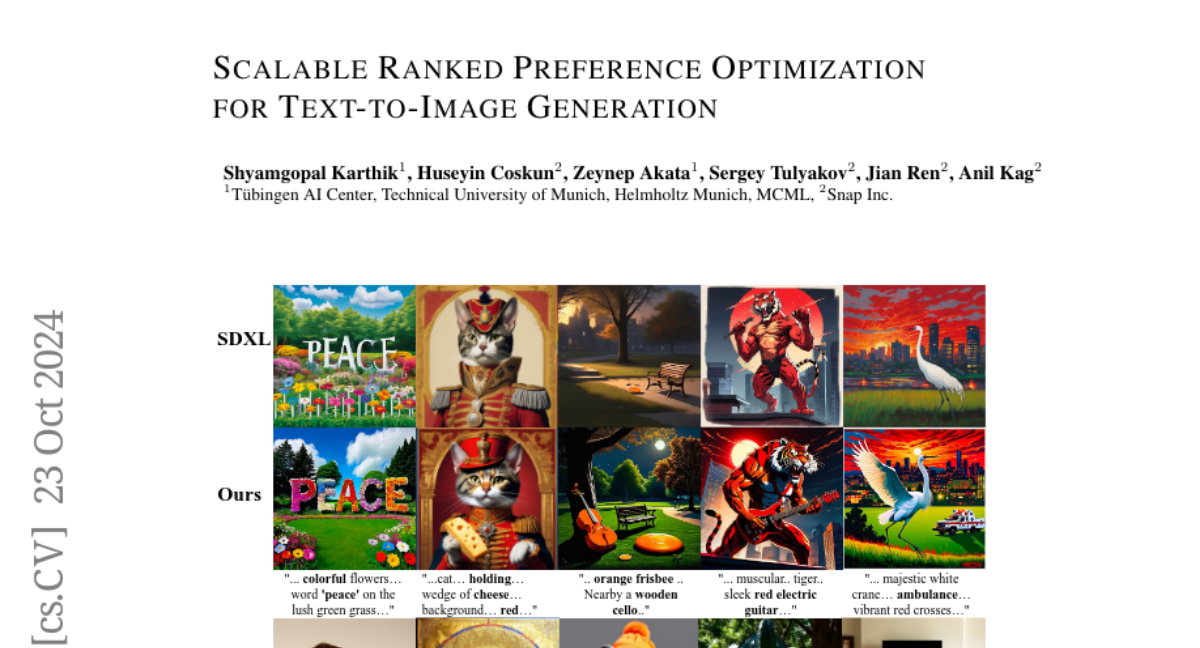
Summary
This paper discusses a new method called Scalable Ranked Preference Optimization (SRPO) for improving text-to-image generation models by using synthetic datasets to better align them with human preferences.
What's the problem?
Text-to-image models need to be trained on large datasets that show human preferences for different generated images. However, collecting and labeling these datasets is very resource-intensive and time-consuming, especially as the quality of models improves quickly, making older datasets less useful.
What's the solution?
The authors propose a scalable approach that generates synthetic datasets without needing human input for labeling. They use a pre-trained reward function to create preferences for paired images automatically. Additionally, they introduce a new method called RankDPO, which allows the model to learn from ranked preferences instead of just pairwise comparisons. This means the model can understand which images are preferred overall rather than just comparing two at a time.
Why it matters?
This research is important because it makes it easier and cheaper to create high-quality datasets that can improve text-to-image models. By enhancing how these models learn from human preferences, we can produce better and more accurate generated images, which can be used in various applications like art creation, advertising, and virtual reality.
Abstract
Direct Preference Optimization (DPO) has emerged as a powerful approach to align text-to-image (T2I) models with human feedback. Unfortunately, successful application of DPO to T2I models requires a huge amount of resources to collect and label large-scale datasets, e.g., millions of generated paired images annotated with human preferences. In addition, these human preference datasets can get outdated quickly as the rapid improvements of T2I models lead to higher quality images. In this work, we investigate a scalable approach for collecting large-scale and fully synthetic datasets for DPO training. Specifically, the preferences for paired images are generated using a pre-trained reward function, eliminating the need for involving humans in the annotation process, greatly improving the dataset collection efficiency. Moreover, we demonstrate that such datasets allow averaging predictions across multiple models and collecting ranked preferences as opposed to pairwise preferences. Furthermore, we introduce RankDPO to enhance DPO-based methods using the ranking feedback. Applying RankDPO on SDXL and SD3-Medium models with our synthetically generated preference dataset ``Syn-Pic'' improves both prompt-following (on benchmarks like T2I-Compbench, GenEval, and DPG-Bench) and visual quality (through user studies). This pipeline presents a practical and scalable solution to develop better preference datasets to enhance the performance of text-to-image models.