Scaling Diffusion Language Models via Adaptation from Autoregressive Models
Shansan Gong, Shivam Agarwal, Yizhe Zhang, Jiacheng Ye, Lin Zheng, Mukai Li, Chenxin An, Peilin Zhao, Wei Bi, Jiawei Han, Hao Peng, Lingpeng Kong
2024-10-24
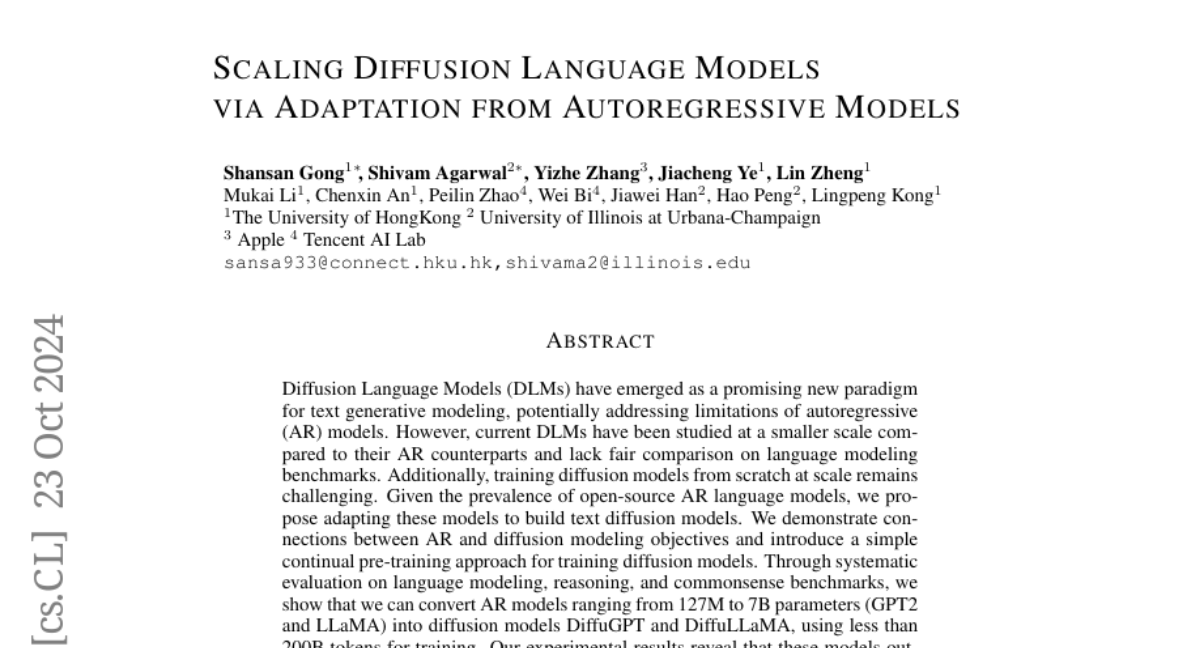
Summary
This paper discusses a new method called MIA-DPO, which helps large vision-language models (LVLMs) better understand and predict human preferences when analyzing multiple images at once.
What's the problem?
Current methods for training LVLMs mainly focus on single images and struggle with the complexity of multiple images. This is because there is not enough diverse training data available, and collecting data for multi-image scenarios can be expensive and time-consuming.
What's the solution?
MIA-DPO addresses these challenges by using a technique that enhances single-image data with unrelated images arranged in creative formats, like grid collages. This approach reduces the need for extensive human annotations while still allowing the model to learn effectively. The method also uses attention values from the model to filter out incorrect responses, ensuring that the model learns from the right examples without needing extra data or human input.
Why it matters?
This research is important because it improves how LVLMs analyze multiple images, making them more effective for real-world applications like image recognition and understanding complex visual information. By enhancing these models' performance, MIA-DPO can create AI systems that better align with human preferences and needs.
Abstract
Diffusion Language Models (DLMs) have emerged as a promising new paradigm for text generative modeling, potentially addressing limitations of autoregressive (AR) models. However, current DLMs have been studied at a smaller scale compared to their AR counterparts and lack fair comparison on language modeling benchmarks. Additionally, training diffusion models from scratch at scale remains challenging. Given the prevalence of open-source AR language models, we propose adapting these models to build text diffusion models. We demonstrate connections between AR and diffusion modeling objectives and introduce a simple continual pre-training approach for training diffusion models. Through systematic evaluation on language modeling, reasoning, and commonsense benchmarks, we show that we can convert AR models ranging from 127M to 7B parameters (GPT2 and LLaMA) into diffusion models DiffuGPT and DiffuLLaMA, using less than 200B tokens for training. Our experimental results reveal that these models outperform earlier DLMs and are competitive with their AR counterparts. We release a suite of DLMs (with 127M, 355M, and 7B parameters) capable of generating fluent text, performing in-context learning, filling in the middle without prompt re-ordering, and following instructions https://github.com/HKUNLP/DiffuLLaMA.