Scaling Granite Code Models to 128K Context
Matt Stallone, Vaibhav Saxena, Leonid Karlinsky, Bridget McGinn, Tim Bula, Mayank Mishra, Adriana Meza Soria, Gaoyuan Zhang, Aditya Prasad, Yikang Shen, Saptha Surendran, Shanmukha Guttula, Hima Patel, Parameswaran Selvam, Xuan-Hong Dang, Yan Koyfman, Atin Sood, Rogerio Feris, Nirmit Desai, David D. Cox, Ruchir Puri, Rameswar Panda
2024-07-19
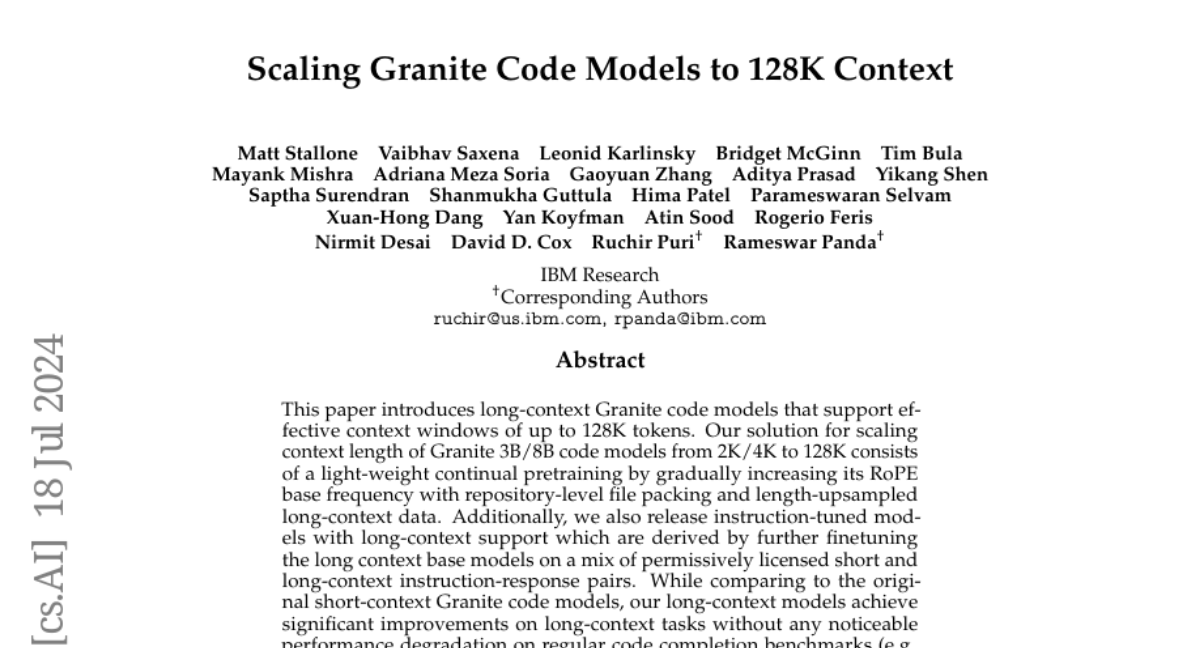
Summary
This paper presents new Granite code models that can handle much longer pieces of text, up to 128,000 tokens. This allows the models to better understand and generate complex code and text compared to older models that could only manage shorter contexts.
What's the problem?
Previous code models had a limited context window, meaning they could only consider a small amount of text at one time (like 2,000 or 4,000 tokens). This limitation made it difficult for them to understand longer pieces of code or text, which is often necessary for complex programming tasks.
What's the solution?
The authors developed a method to extend the context length of Granite code models through a process called continual pretraining. They gradually increased the model's ability to handle longer texts while training it on a large dataset of code. They also fine-tuned these models with both short and long instruction-response pairs to improve their performance. The new models showed significant improvements in handling long-context tasks without losing effectiveness on regular coding tasks.
Why it matters?
This research is important because it enhances the capabilities of AI in programming. By allowing models to understand and generate longer pieces of code, developers can create more sophisticated AI tools that assist with complex coding tasks, leading to increased productivity and better software development.
Abstract
This paper introduces long-context Granite code models that support effective context windows of up to 128K tokens. Our solution for scaling context length of Granite 3B/8B code models from 2K/4K to 128K consists of a light-weight continual pretraining by gradually increasing its RoPE base frequency with repository-level file packing and length-upsampled long-context data. Additionally, we also release instruction-tuned models with long-context support which are derived by further finetuning the long context base models on a mix of permissively licensed short and long-context instruction-response pairs. While comparing to the original short-context Granite code models, our long-context models achieve significant improvements on long-context tasks without any noticeable performance degradation on regular code completion benchmarks (e.g., HumanEval). We release all our long-context Granite code models under an Apache 2.0 license for both research and commercial use.