Scaling Proprioceptive-Visual Learning with Heterogeneous Pre-trained Transformers
Lirui Wang, Xinlei Chen, Jialiang Zhao, Kaiming He
2024-10-01
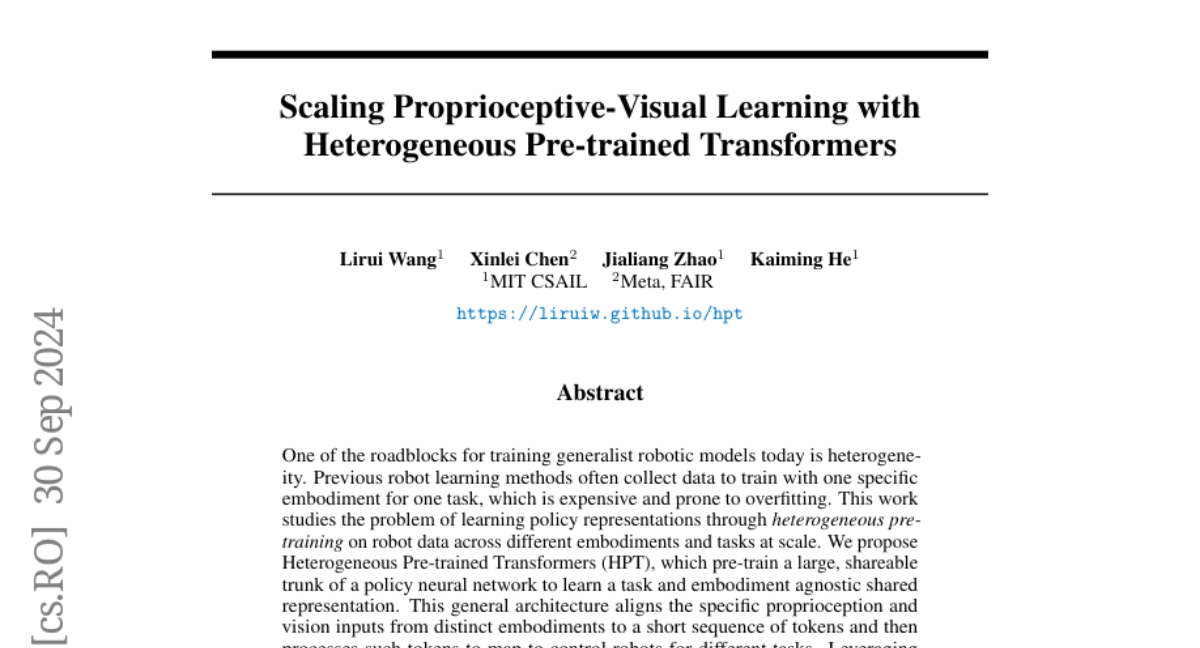
Summary
This paper introduces Heterogeneous Pre-trained Transformers (HPT), a new method for training robots that helps them learn to perform tasks better by using diverse data from different types of robots and environments.
What's the problem?
Training robots has been challenging because most methods focus on one specific type of robot and task, which can be costly and lead to overfitting. This means the robots might only perform well in the situations they were trained on and struggle with new tasks or environments.
What's the solution?
HPT addresses this issue by using a unified approach that combines data from various robot types and tasks. It creates a shared model that learns from different inputs, like camera images and sensor data, allowing it to adapt to new situations more easily. The researchers tested HPT on a large dataset with 52 different sources, showing that it significantly improved robot performance by over 20% on tasks they hadn't seen before.
Why it matters?
This research is important because it opens up new possibilities for training robots to be more versatile and capable in real-world applications. By learning from a wider range of experiences, robots can become more adaptable, making them useful in many fields such as manufacturing, healthcare, and service industries.
Abstract
One of the roadblocks for training generalist robotic models today is heterogeneity. Previous robot learning methods often collect data to train with one specific embodiment for one task, which is expensive and prone to overfitting. This work studies the problem of learning policy representations through heterogeneous pre-training on robot data across different embodiments and tasks at scale. We propose Heterogeneous Pre-trained Transformers (HPT), which pre-train a large, shareable trunk of a policy neural network to learn a task and embodiment agnostic shared representation. This general architecture aligns the specific proprioception and vision inputs from distinct embodiments to a short sequence of tokens and then processes such tokens to map to control robots for different tasks. Leveraging the recent large-scale multi-embodiment real-world robotic datasets as well as simulation, deployed robots, and human video datasets, we investigate pre-training policies across heterogeneity. We conduct experiments to investigate the scaling behaviors of training objectives, to the extent of 52 datasets. HPTs outperform several baselines and enhance the fine-tuned policy performance by over 20% on unseen tasks in multiple simulator benchmarks and real-world settings. See the project website (https://liruiw.github.io/hpt/) for code and videos.