Scaling Smart: Accelerating Large Language Model Pre-training with Small Model Initialization
Mohammad Samragh, Iman Mirzadeh, Keivan Alizadeh Vahid, Fartash Faghri, Minsik Cho, Moin Nabi, Devang Naik, Mehrdad Farajtabar
2024-09-20
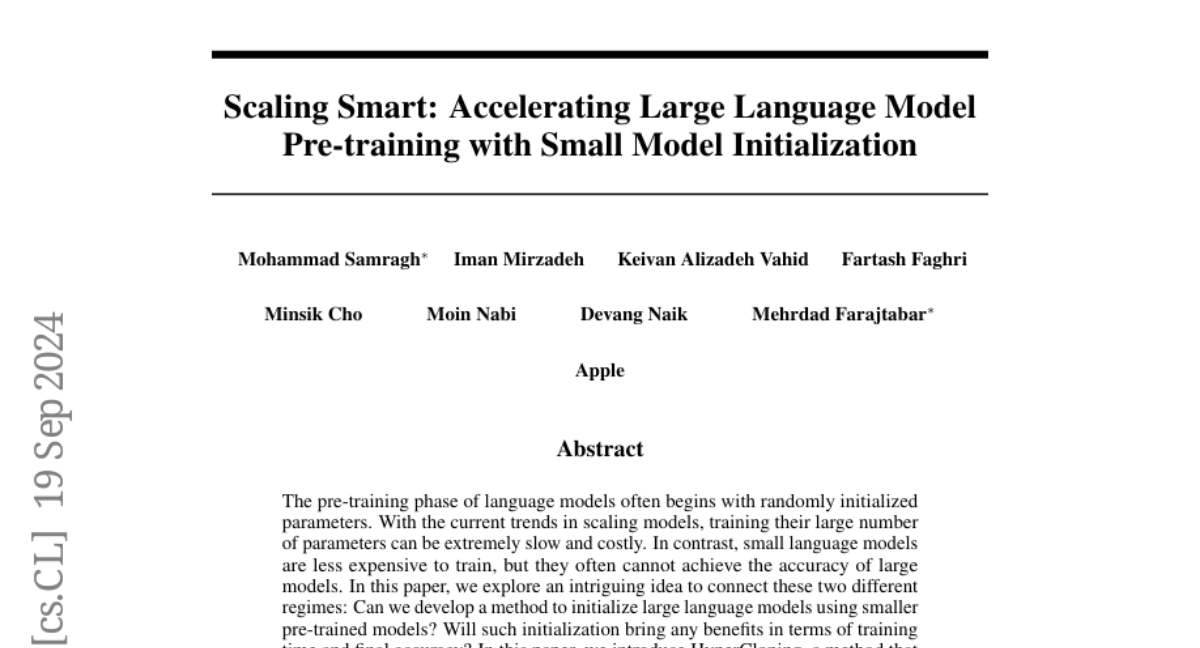
Summary
This paper discusses a new method called HyperCloning that helps speed up the training of large language models (LLMs) by starting with smaller, pre-trained models instead of random initial settings.
What's the problem?
Training large language models from scratch can be very slow and expensive because they have many parameters to adjust. While smaller models are cheaper and faster to train, they usually don't perform as well as larger ones. This creates a challenge: how can we efficiently train large models without starting from zero?
What's the solution?
The authors introduce HyperCloning, a technique that allows them to use the knowledge from smaller, already trained models to initialize larger models. This means that the larger model starts with some understanding of language, making it quicker to learn and achieve better accuracy. HyperCloning expands the smaller model's parameters while keeping its capabilities intact, leading to faster training times and less computational cost. Their experiments show that this method can speed up training by 2 to 4 times compared to traditional methods.
Why it matters?
This research is important because it provides a more efficient way to train large language models, which are crucial for many AI applications like chatbots, translation services, and content generation. By improving the training process, HyperCloning can help make advanced AI technologies more accessible and effective.
Abstract
The pre-training phase of language models often begins with randomly initialized parameters. With the current trends in scaling models, training their large number of parameters can be extremely slow and costly. In contrast, small language models are less expensive to train, but they often cannot achieve the accuracy of large models. In this paper, we explore an intriguing idea to connect these two different regimes: Can we develop a method to initialize large language models using smaller pre-trained models? Will such initialization bring any benefits in terms of training time and final accuracy? In this paper, we introduce HyperCloning, a method that can expand the parameters of a pre-trained language model to those of a larger model with increased hidden dimensions. Our method ensures that the larger model retains the functionality of the smaller model. As a result, the larger model already inherits the predictive power and accuracy of the smaller model before the training starts. We demonstrate that training such an initialized model results in significant savings in terms of GPU hours required for pre-training large language models.