ScienceAgentBench: Toward Rigorous Assessment of Language Agents for Data-Driven Scientific Discovery
Ziru Chen, Shijie Chen, Yuting Ning, Qianheng Zhang, Boshi Wang, Botao Yu, Yifei Li, Zeyi Liao, Chen Wei, Zitong Lu, Vishal Dey, Mingyi Xue, Frazier N. Baker, Benjamin Burns, Daniel Adu-Ampratwum, Xuhui Huang, Xia Ning, Song Gao, Yu Su, Huan Sun
2024-10-08
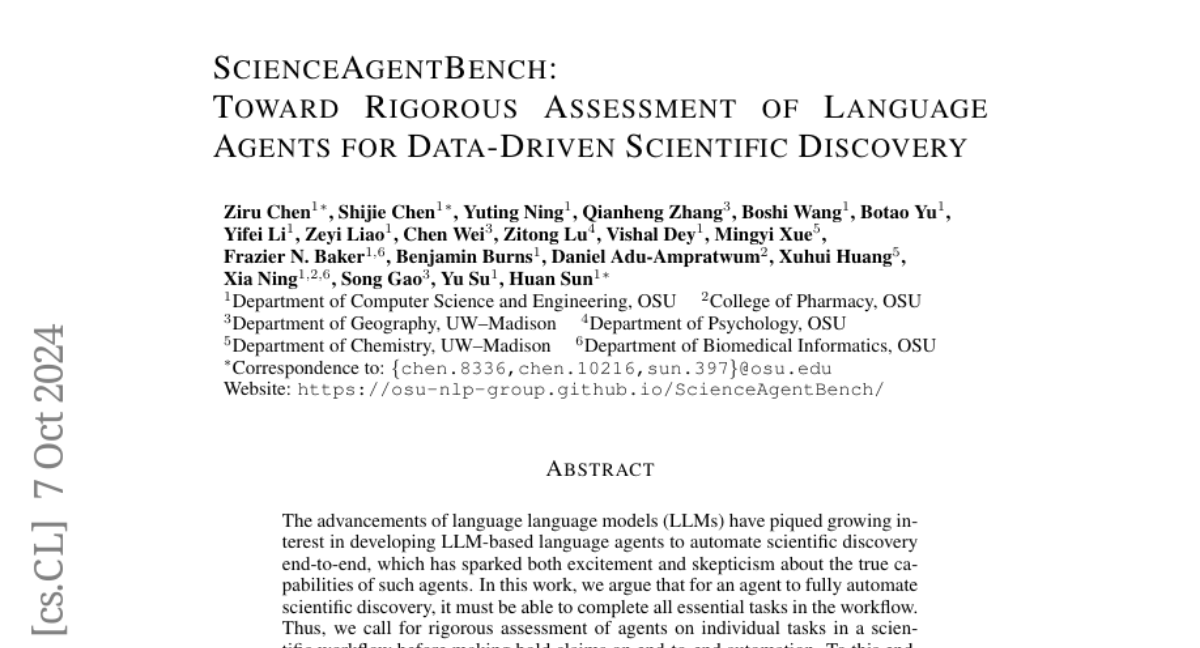
Summary
This paper introduces ScienceAgentBench, a new benchmark designed to evaluate the performance of language agents in automating scientific discovery tasks, ensuring that these agents can effectively handle all necessary steps in the scientific workflow.
What's the problem?
As language models (LLMs) become more advanced, there's excitement about their potential to automate scientific research. However, there is skepticism about whether these models can truly perform all the tasks needed for complete scientific discovery. Many existing assessments do not rigorously evaluate how well these agents can handle individual tasks within a scientific workflow, which is crucial for understanding their true capabilities.
What's the solution?
To address this issue, the authors developed ScienceAgentBench, which consists of 102 carefully selected tasks drawn from peer-reviewed scientific publications across various fields. They worked with experts to validate these tasks and ensured that each task's output is a self-contained Python program. The benchmark uses multiple evaluation metrics to assess the quality of the generated programs and their execution results. This rigorous approach helps to accurately measure how well language agents can perform specific scientific tasks.
Why it matters?
This research is important because it sets a standard for evaluating language agents in scientific contexts. By providing a comprehensive and validated benchmark, ScienceAgentBench helps researchers understand the limitations and capabilities of current language models. This could lead to improvements in how these models assist scientists, ultimately enhancing productivity in data-driven scientific discovery.
Abstract
The advancements of language language models (LLMs) have piqued growing interest in developing LLM-based language agents to automate scientific discovery end-to-end, which has sparked both excitement and skepticism about the true capabilities of such agents. In this work, we argue that for an agent to fully automate scientific discovery, it must be able to complete all essential tasks in the workflow. Thus, we call for rigorous assessment of agents on individual tasks in a scientific workflow before making bold claims on end-to-end automation. To this end, we present ScienceAgentBench, a new benchmark for evaluating language agents for data-driven scientific discovery. To ensure the scientific authenticity and real-world relevance of our benchmark, we extract 102 tasks from 44 peer-reviewed publications in four disciplines and engage nine subject matter experts to validate them. We unify the target output for every task to a self-contained Python program file and employ an array of evaluation metrics to examine the generated programs, execution results, and costs. Each task goes through multiple rounds of manual validation by annotators and subject matter experts to ensure its annotation quality and scientific plausibility. We also propose two effective strategies to mitigate data contamination concerns. Using our benchmark, we evaluate five open-weight and proprietary LLMs, each with three frameworks: direct prompting, OpenHands, and self-debug. Given three attempts for each task, the best-performing agent can only solve 32.4% of the tasks independently and 34.3% with expert-provided knowledge. These results underscore the limited capacities of current language agents in generating code for data-driven discovery, let alone end-to-end automation for scientific research.