SciLitLLM: How to Adapt LLMs for Scientific Literature Understanding
Sihang Li, Jin Huang, Jiaxi Zhuang, Yaorui Shi, Xiaochen Cai, Mingjun Xu, Xiang Wang, Linfeng Zhang, Guolin Ke, Hengxing Cai
2024-09-02
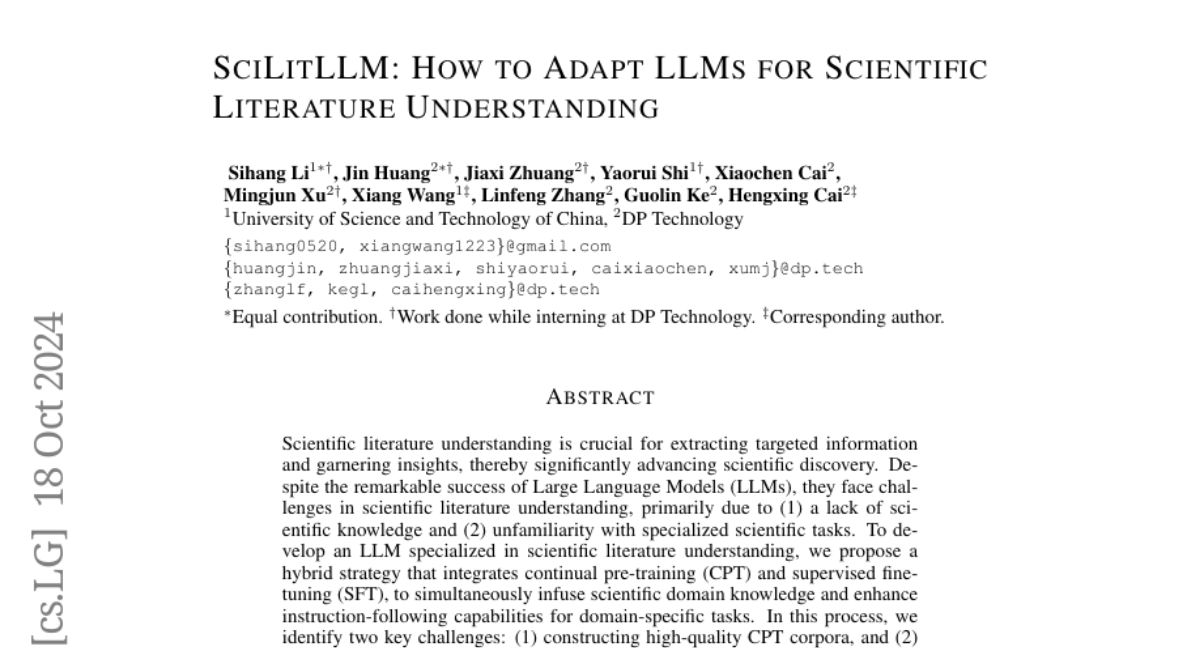
Summary
This paper introduces SciLitLLM, a method for improving how Large Language Models (LLMs) understand scientific literature, making them better at extracting useful information from research papers.
What's the problem?
While LLMs have been successful in many areas, they struggle with scientific texts because they often lack specific scientific knowledge and don't perform well on specialized tasks. This makes it hard for them to accurately interpret and summarize complex research findings.
What's the solution?
To solve this issue, the authors propose a hybrid approach that combines continual pre-training (CPT) and supervised fine-tuning (SFT). This method helps LLMs gain scientific knowledge and learn how to follow instructions for specific scientific tasks. They tackle challenges like creating high-quality training data and generating diverse instructions for different scientific domains. The result is a suite of models called SciLitLLM that performs well on benchmarks for understanding scientific literature.
Why it matters?
This research is significant because it enhances the ability of AI to process and understand scientific texts, which can accelerate discoveries in various fields. By improving how LLMs work with research papers, it can help scientists and researchers access important information more efficiently.
Abstract
Scientific literature understanding is crucial for extracting targeted information and garnering insights, thereby significantly advancing scientific discovery. Despite the remarkable success of Large Language Models (LLMs), they face challenges in scientific literature understanding, primarily due to (1) a lack of scientific knowledge and (2) unfamiliarity with specialized scientific tasks. To develop an LLM specialized in scientific literature understanding, we propose a hybrid strategy that integrates continual pre-training (CPT) and supervised fine-tuning (SFT), to simultaneously infuse scientific domain knowledge and enhance instruction-following capabilities for domain-specific tasks.cIn this process, we identify two key challenges: (1) constructing high-quality CPT corpora, and (2) generating diverse SFT instructions. We address these challenges through a meticulous pipeline, including PDF text extraction, parsing content error correction, quality filtering, and synthetic instruction creation. Applying this strategy, we present a suite of LLMs: SciLitLLM, specialized in scientific literature understanding. These models demonstrate promising performance on scientific literature understanding benchmarks. Our contributions are threefold: (1) We present an effective framework that integrates CPT and SFT to adapt LLMs to scientific literature understanding, which can also be easily adapted to other domains. (2) We propose an LLM-based synthesis method to generate diverse and high-quality scientific instructions, resulting in a new instruction set -- SciLitIns -- for supervised fine-tuning in less-represented scientific domains. (3) SciLitLLM achieves promising performance improvements on scientific literature understanding benchmarks.