SciPrompt: Knowledge-augmented Prompting for Fine-grained Categorization of Scientific Topics
Zhiwen You, Kanyao Han, Haotian Zhu, Bertram Ludäscher, Jana Diesner
2024-10-04
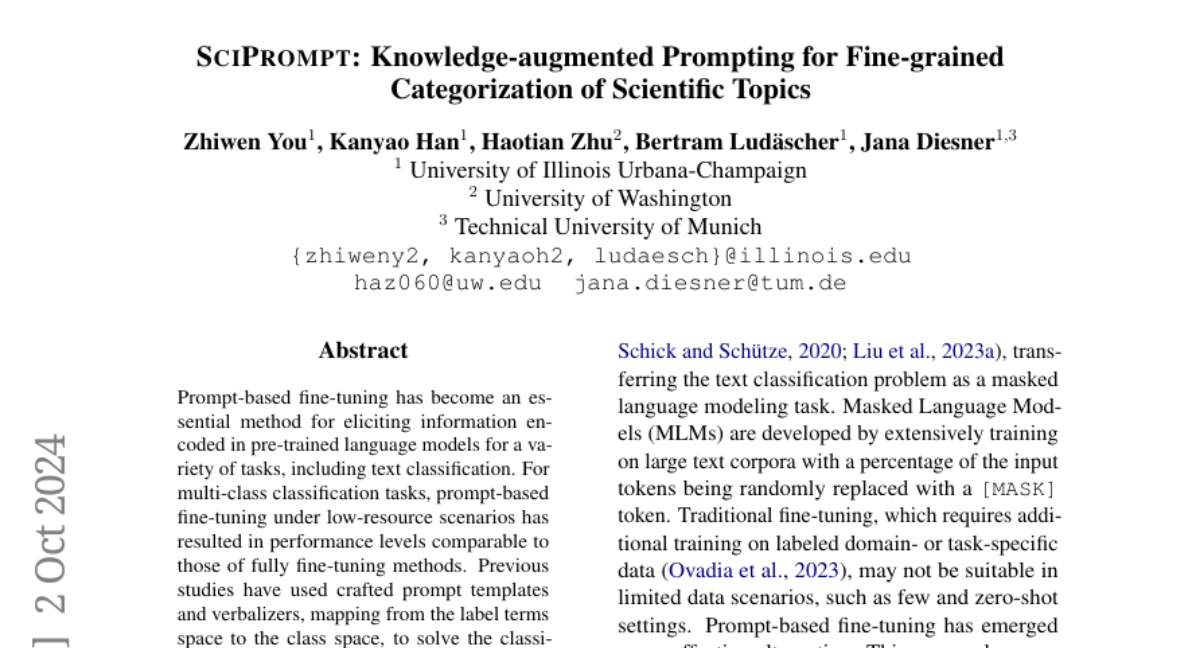
Summary
This paper introduces SciPrompt, a new framework designed to improve the classification of scientific texts into specific topics by automatically retrieving and using relevant scientific terms.
What's the problem?
Classifying scientific texts can be challenging, especially when there is limited labeled data available. Traditional methods often rely on manually created prompts and terms, which can be time-consuming and require expert knowledge. This makes it difficult to categorize texts accurately, particularly for fine-grained topics that need more specific labels.
What's the solution?
To solve this problem, the authors developed SciPrompt, which automatically gathers scientific topic-related terms from existing literature. This framework enhances the classification process by selecting relevant terms that help the model understand the context better. They also introduced a new way of verbalizing these terms that uses correlation scores to improve predictions. By doing this, SciPrompt allows language models to perform better in classifying scientific texts, even when only a few examples are provided for training.
Why it matters?
This research is important because it shows how automated methods can make scientific text classification more efficient and accurate. By reducing the reliance on manual input and leveraging existing knowledge, SciPrompt can help researchers and scientists categorize their work more effectively, leading to better organization and accessibility of scientific information.
Abstract
Prompt-based fine-tuning has become an essential method for eliciting information encoded in pre-trained language models for a variety of tasks, including text classification. For multi-class classification tasks, prompt-based fine-tuning under low-resource scenarios has resulted in performance levels comparable to those of fully fine-tuning methods. Previous studies have used crafted prompt templates and verbalizers, mapping from the label terms space to the class space, to solve the classification problem as a masked language modeling task. However, cross-domain and fine-grained prompt-based fine-tuning with an automatically enriched verbalizer remains unexplored, mainly due to the difficulty and costs of manually selecting domain label terms for the verbalizer, which requires humans with domain expertise. To address this challenge, we introduce SciPrompt, a framework designed to automatically retrieve scientific topic-related terms for low-resource text classification tasks. To this end, we select semantically correlated and domain-specific label terms within the context of scientific literature for verbalizer augmentation. Furthermore, we propose a new verbalization strategy that uses correlation scores as additional weights to enhance the prediction performance of the language model during model tuning. Our method outperforms state-of-the-art, prompt-based fine-tuning methods on scientific text classification tasks under few and zero-shot settings, especially in classifying fine-grained and emerging scientific topics.