SeerAttention: Learning Intrinsic Sparse Attention in Your LLMs
Yizhao Gao, Zhichen Zeng, Dayou Du, Shijie Cao, Hayden Kwok-Hay So, Ting Cao, Fan Yang, Mao Yang
2024-10-21
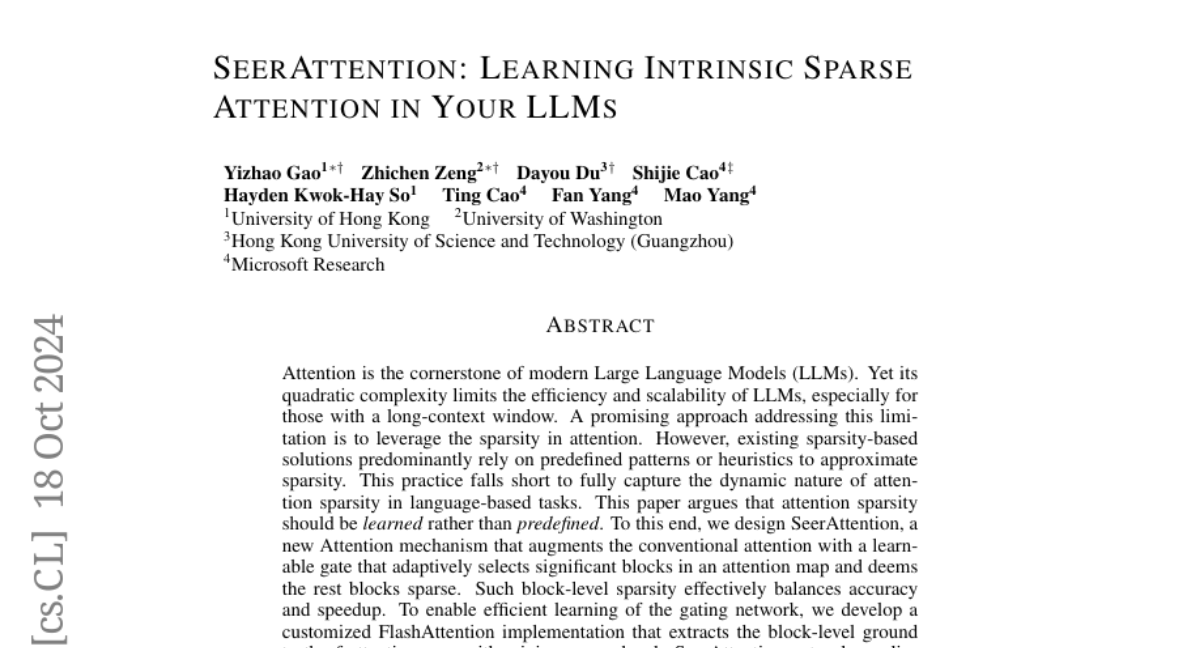
Summary
This paper introduces SeerAttention, a new method for improving the efficiency of large language models (LLMs) by learning how to focus on important parts of the input data while ignoring less relevant information.
What's the problem?
Large language models use a mechanism called attention to understand the relationships between words in a sentence. However, this attention process can be very slow and resource-intensive, especially when dealing with long pieces of text. Current methods for making attention more efficient often rely on fixed patterns, which don't adapt well to different situations or types of data.
What's the solution?
To solve this problem, the authors developed SeerAttention, which learns to identify which parts of the input are most important and focuses on those while ignoring the rest. This is done by adding a learnable gate that decides which blocks of information to pay attention to. They also created a special version of an existing attention mechanism called FlashAttention that helps train this new system efficiently. Their experiments showed that SeerAttention can significantly speed up processing times while maintaining high accuracy, even when handling long texts.
Why it matters?
This research is important because it makes large language models faster and more efficient, which is crucial for real-world applications where speed matters, such as chatbots, translation services, and content generation. By improving how these models handle attention, SeerAttention helps pave the way for more powerful and practical AI systems.
Abstract
Attention is the cornerstone of modern Large Language Models (LLMs). Yet its quadratic complexity limits the efficiency and scalability of LLMs, especially for those with a long-context window. A promising approach addressing this limitation is to leverage the sparsity in attention. However, existing sparsity-based solutions predominantly rely on predefined patterns or heuristics to approximate sparsity. This practice falls short to fully capture the dynamic nature of attention sparsity in language-based tasks. This paper argues that attention sparsity should be learned rather than predefined. To this end, we design SeerAttention, a new Attention mechanism that augments the conventional attention with a learnable gate that adaptively selects significant blocks in an attention map and deems the rest blocks sparse. Such block-level sparsity effectively balances accuracy and speedup. To enable efficient learning of the gating network, we develop a customized FlashAttention implementation that extracts the block-level ground truth of attention map with minimum overhead. SeerAttention not only applies to post-training, but also excels in long-context fine-tuning. Our results show that at post-training stages, SeerAttention significantly outperforms state-of-the-art static or heuristic-based sparse attention methods, while also being more versatile and flexible to adapt to varying context lengths and sparsity ratios. When applied to long-context fine-tuning with YaRN, SeerAttention can achieve a remarkable 90% sparsity ratio at a 32k context length with minimal perplexity loss, offering a 5.67x speedup over FlashAttention-2.