Selective Aggregation for Low-Rank Adaptation in Federated Learning
Pengxin Guo, Shuang Zeng, Yanran Wang, Huijie Fan, Feifei Wang, Liangqiong Qu
2024-10-03
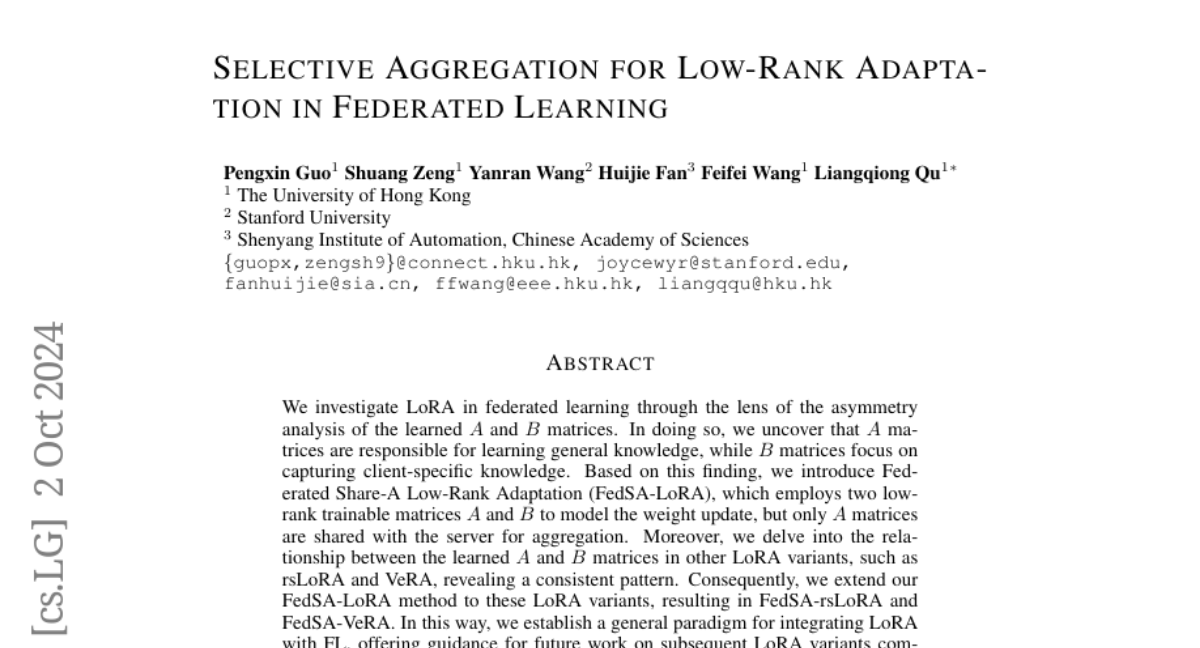
Summary
This paper discusses Selective Aggregation for Low-Rank Adaptation in Federated Learning, a new method that improves how AI models learn from data collected across different devices while keeping the data private.
What's the problem?
In federated learning, multiple devices (like smartphones) train AI models together without sharing their data. However, existing methods for training these models struggle to balance general knowledge and specific knowledge from individual devices. This can lead to inefficiencies and difficulties in learning effectively because the data formats used for training are often incompatible.
What's the solution?
The authors introduce a method called Federated Share-A Low-Rank Adaptation (FedSA-LoRA), which uses two types of low-rank matrices: one for general knowledge (A) and one for client-specific knowledge (B). Only the A matrices are shared with a central server for aggregation, which helps improve the learning process. They also explored how this method can be extended to other similar models (rsLoRA and VeRA) to enhance their performance. The researchers conducted experiments that showed their method significantly improves the accuracy of AI models in understanding and generating language.
Why it matters?
This research is important because it enhances the ability of AI systems to learn from diverse datasets while maintaining user privacy. By improving how models adapt to both general and specific knowledge, FedSA-LoRA can lead to more effective AI applications in various fields, such as healthcare and finance, where data privacy is crucial.
Abstract
We investigate LoRA in federated learning through the lens of the asymmetry analysis of the learned A and B matrices. In doing so, we uncover that A matrices are responsible for learning general knowledge, while B matrices focus on capturing client-specific knowledge. Based on this finding, we introduce Federated Share-A Low-Rank Adaptation (FedSA-LoRA), which employs two low-rank trainable matrices A and B to model the weight update, but only A matrices are shared with the server for aggregation. Moreover, we delve into the relationship between the learned A and B matrices in other LoRA variants, such as rsLoRA and VeRA, revealing a consistent pattern. Consequently, we extend our FedSA-LoRA method to these LoRA variants, resulting in FedSA-rsLoRA and FedSA-VeRA. In this way, we establish a general paradigm for integrating LoRA with FL, offering guidance for future work on subsequent LoRA variants combined with FL. Extensive experimental results on natural language understanding and generation tasks demonstrate the effectiveness of the proposed method.