Self-Supervised Audio-Visual Soundscape Stylization
Tingle Li, Renhao Wang, Po-Yao Huang, Andrew Owens, Gopala Anumanchipalli
2024-09-24
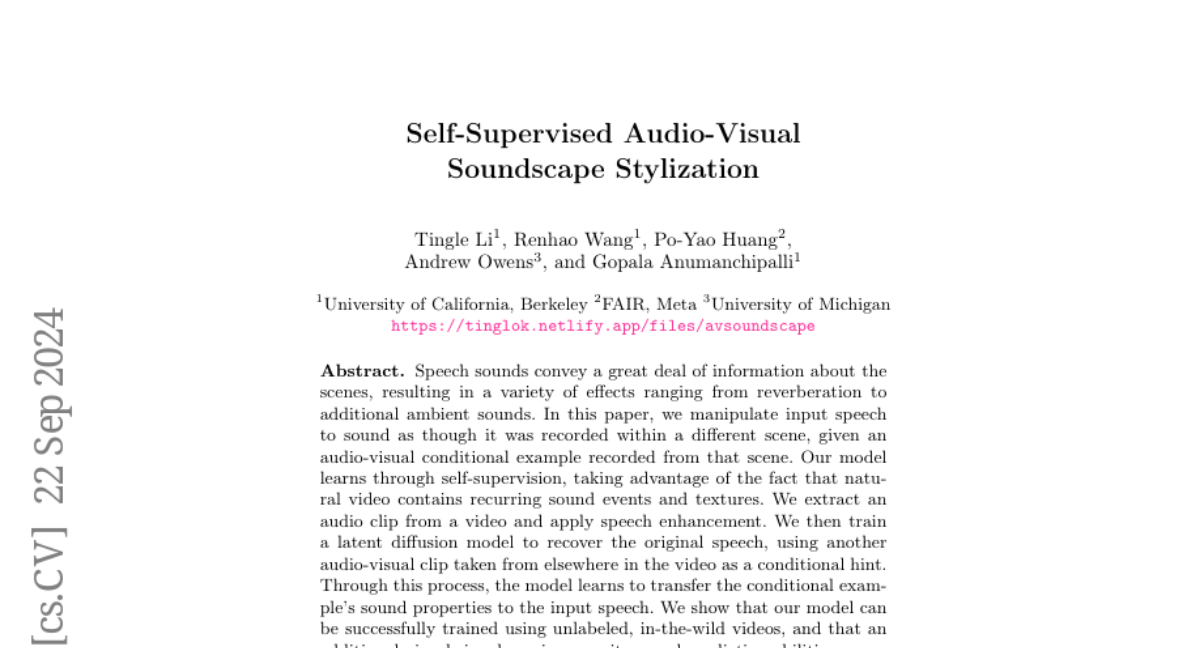
Summary
This paper discusses a new method called Self-Supervised Audio-Visual Soundscape Stylization, which allows speech sounds to be manipulated so they appear as if they were recorded in different environments. The system uses generative AI to enhance audio based on visual context.
What's the problem?
When recording speech sounds, the quality can vary significantly depending on the environment, leading to issues like unwanted echoes or background noise. Existing methods often struggle to accurately recreate how speech would sound in different scenes, making it challenging to produce realistic audio for videos or virtual environments.
What's the solution?
To solve this problem, the researchers developed a model that learns from natural videos, where sounds and visuals are linked. They extract audio clips from videos and enhance them using a process that involves estimating depth and aligning sounds with visual cues. By training their model on a large dataset of unlabeled videos, they enable it to transfer sound properties from one scene to another effectively, improving the overall audio quality.
Why it matters?
This research is important because it enhances how we can manipulate sound in media, making it more realistic and immersive. By improving the quality of audio in various contexts, this technology can benefit filmmakers, game developers, and anyone working with audio-visual content, leading to better user experiences in entertainment and communication.
Abstract
Speech sounds convey a great deal of information about the scenes, resulting in a variety of effects ranging from reverberation to additional ambient sounds. In this paper, we manipulate input speech to sound as though it was recorded within a different scene, given an audio-visual conditional example recorded from that scene. Our model learns through self-supervision, taking advantage of the fact that natural video contains recurring sound events and textures. We extract an audio clip from a video and apply speech enhancement. We then train a latent diffusion model to recover the original speech, using another audio-visual clip taken from elsewhere in the video as a conditional hint. Through this process, the model learns to transfer the conditional example's sound properties to the input speech. We show that our model can be successfully trained using unlabeled, in-the-wild videos, and that an additional visual signal can improve its sound prediction abilities. Please see our project webpage for video results: https://tinglok.netlify.app/files/avsoundscape/