Sentiment Analysis of Lithuanian Online Reviews Using Large Language Models
Brigita Vileikytė, Mantas Lukoševičius, Lukas Stankevičius
2024-07-30
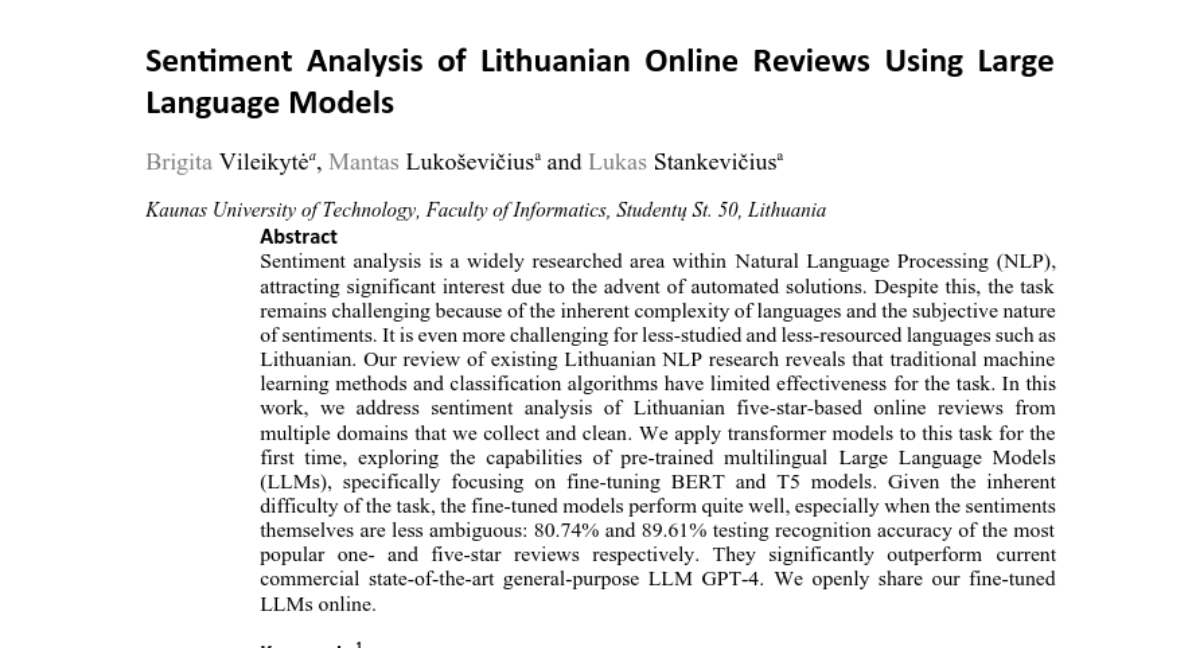
Summary
This paper discusses a new method called Meta-Rewarding that helps large language models (LLMs) improve their ability to judge their own responses. By using a model as a 'meta-judge,' it can refine its judgment skills and enhance overall performance without needing human feedback.
What's the problem?
Improving LLMs typically requires a lot of expensive human-generated data. While some methods allow models to learn by judging their own answers, they mainly focus on improving the responses rather than the judgment process itself. This can lead to quick saturation, where the model stops getting better because it doesn't enhance its ability to evaluate its outputs.
What's the solution?
To address this issue, the authors introduce the Meta-Rewarding method, which allows the model to judge its own judgments. This self-evaluating process helps the model learn to improve both its responses and its judging capabilities. The authors tested this approach on a specific model called Llama-3-8B-Instruct and found significant improvements in its performance on various benchmarks, indicating that this method can lead to better instruction-following abilities.
Why it matters?
This research is important because it suggests a way for AI models to become more capable and aligned with human values without relying heavily on human input. By enabling models to self-improve through their own evaluations, it could make them more effective in various applications, leading to smarter and more reliable AI systems.
Abstract
Sentiment analysis is a widely researched area within Natural Language Processing (NLP), attracting significant interest due to the advent of automated solutions. Despite this, the task remains challenging because of the inherent complexity of languages and the subjective nature of sentiments. It is even more challenging for less-studied and less-resourced languages such as Lithuanian. Our review of existing Lithuanian NLP research reveals that traditional machine learning methods and classification algorithms have limited effectiveness for the task. In this work, we address sentiment analysis of Lithuanian five-star-based online reviews from multiple domains that we collect and clean. We apply transformer models to this task for the first time, exploring the capabilities of pre-trained multilingual Large Language Models (LLMs), specifically focusing on fine-tuning BERT and T5 models. Given the inherent difficulty of the task, the fine-tuned models perform quite well, especially when the sentiments themselves are less ambiguous: 80.74% and 89.61% testing recognition accuracy of the most popular one- and five-star reviews respectively. They significantly outperform current commercial state-of-the-art general-purpose LLM GPT-4. We openly share our fine-tuned LLMs online.