SG-I2V: Self-Guided Trajectory Control in Image-to-Video Generation
Koichi Namekata, Sherwin Bahmani, Ziyi Wu, Yash Kant, Igor Gilitschenski, David B. Lindell
2024-11-08
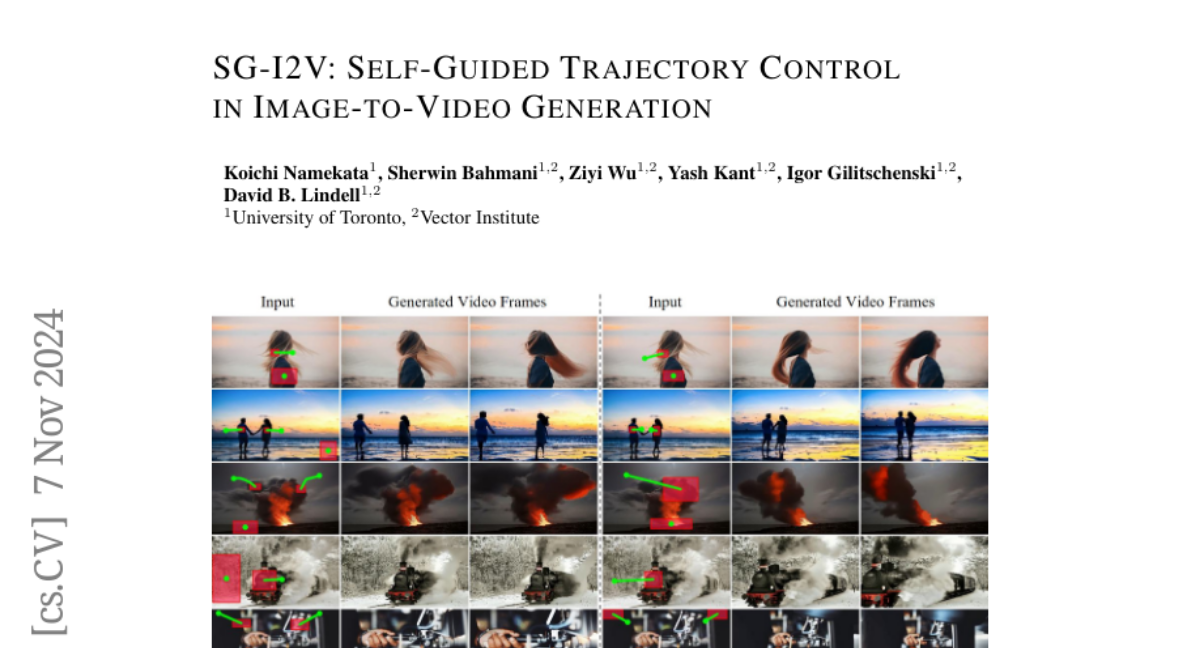
Summary
This paper introduces SG-I2V, a new method for generating videos from images that allows users to control specific elements like object movement and camera angles without needing to retrain the model.
What's the problem?
Generating videos from images can be challenging, especially when trying to adjust details like how objects move or how the camera captures the scene. Current methods often require a lot of trial and error, which can be time-consuming and frustrating. Additionally, fine-tuning models for these adjustments can be expensive and requires special datasets that are hard to obtain.
What's the solution?
SG-I2V offers a self-guided approach that enables users to control video generation without any additional training or external data. It uses a pre-trained image-to-video diffusion model to understand how to manipulate the motion of objects and camera dynamics based on user-defined bounding boxes and trajectories. This means users can specify where they want objects to move in the video, and SG-I2V will generate the video accordingly, all without needing to retrain the model on new data.
Why it matters?
This research is important because it simplifies the process of creating videos from images, making it more accessible for users who want to customize their content quickly. By eliminating the need for complex training procedures, SG-I2V can enhance creative projects in areas like filmmaking, animation, and virtual reality, allowing for more interactive and personalized experiences.
Abstract
Methods for image-to-video generation have achieved impressive, photo-realistic quality. However, adjusting specific elements in generated videos, such as object motion or camera movement, is often a tedious process of trial and error, e.g., involving re-generating videos with different random seeds. Recent techniques address this issue by fine-tuning a pre-trained model to follow conditioning signals, such as bounding boxes or point trajectories. Yet, this fine-tuning procedure can be computationally expensive, and it requires datasets with annotated object motion, which can be difficult to procure. In this work, we introduce SG-I2V, a framework for controllable image-to-video generation that is self-guidedx2013offering zero-shot control by relying solely on the knowledge present in a pre-trained image-to-video diffusion model without the need for fine-tuning or external knowledge. Our zero-shot method outperforms unsupervised baselines while being competitive with supervised models in terms of visual quality and motion fidelity.