SHAKTI: A 2.5 Billion Parameter Small Language Model Optimized for Edge AI and Low-Resource Environments
Syed Abdul Gaffar Shakhadri, Kruthika KR, Rakshit Aralimatti
2024-10-21
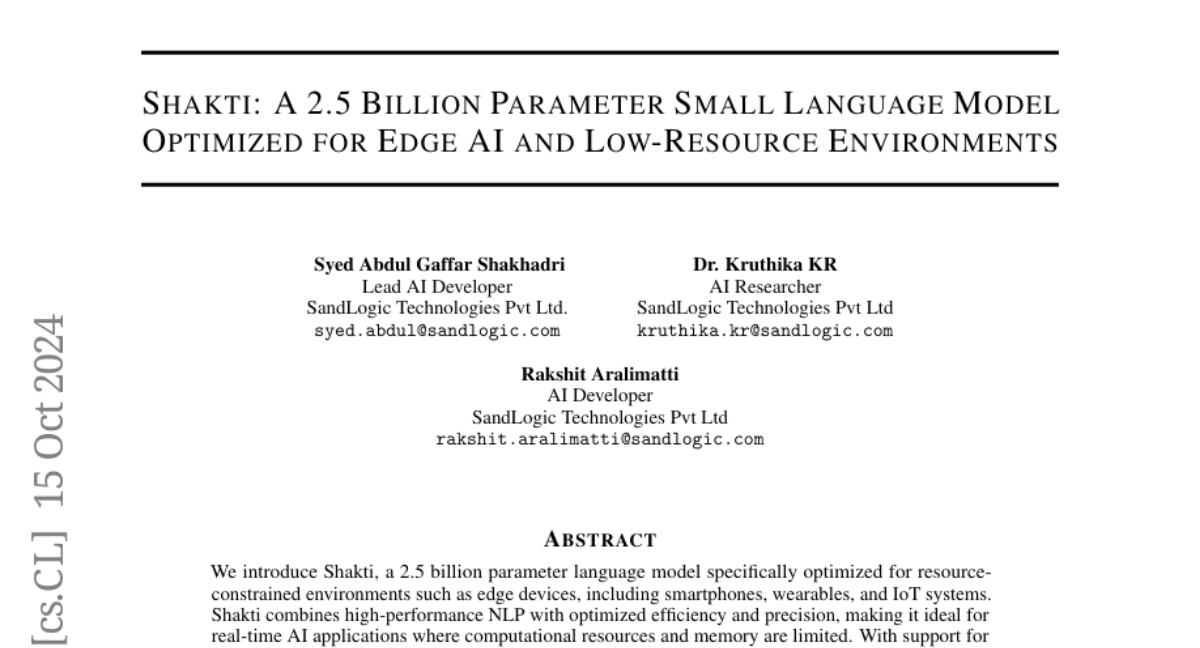
Summary
This paper investigates the effectiveness of AI detectors that identify machine-generated texts and questions whether their high accuracy scores are truly reliable or just a result of poor-quality datasets.
What's the problem?
As AI language models improve, the need for reliable detectors to distinguish between human-written and AI-generated text has become crucial. While some detectors claim to be highly accurate in controlled tests, they often perform poorly in real-world situations. This raises concerns about whether these detectors can be trusted, especially if their success comes from testing on low-quality datasets.
What's the solution?
The authors conducted a survey of various datasets used for training AI detectors and proposed methods for evaluating the quality of these datasets. They emphasized the importance of creating robust evaluation methods to ensure that detectors are effective in identifying AI-generated content. Additionally, they explored how using high-quality generated data could improve both the training of detection models and the datasets themselves.
Why it matters?
This research is important because as AI-generated content becomes more common, ensuring the reliability of tools that identify such content is essential. By improving how we evaluate AI detectors and their training data, we can create more trustworthy systems that help maintain the integrity of information in various fields, such as journalism, education, and online content creation.
Abstract
We introduce Shakti, a 2.5 billion parameter language model specifically optimized for resource-constrained environments such as edge devices, including smartphones, wearables, and IoT systems. Shakti combines high-performance NLP with optimized efficiency and precision, making it ideal for real-time AI applications where computational resources and memory are limited. With support for vernacular languages and domain-specific tasks, Shakti excels in industries such as healthcare, finance, and customer service. Benchmark evaluations demonstrate that Shakti performs competitively against larger models while maintaining low latency and on-device efficiency, positioning it as a leading solution for edge AI.