Shape of Motion: 4D Reconstruction from a Single Video
Qianqian Wang, Vickie Ye, Hang Gao, Jake Austin, Zhengqi Li, Angjoo Kanazawa
2024-07-19
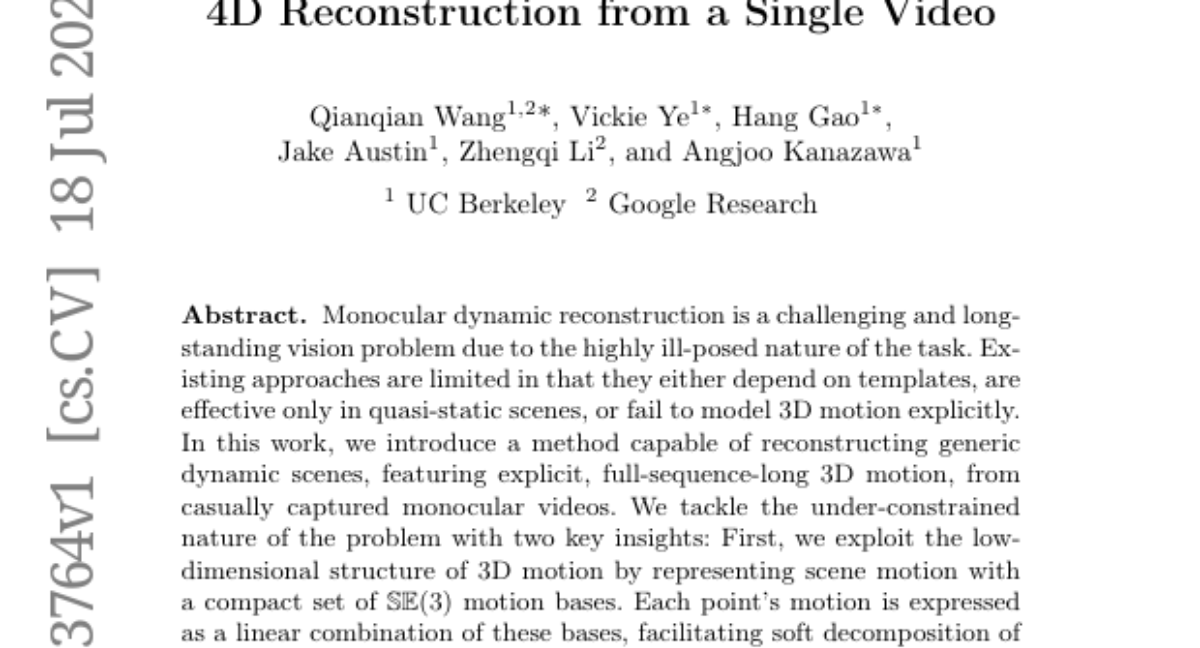
Summary
This paper presents a new method for reconstructing 4D scenes from a single video, allowing for a detailed understanding of dynamic movements in a scene over time. It introduces a way to capture and represent complex motions using only one camera.
What's the problem?
Reconstructing dynamic scenes from videos is very challenging because it's hard to accurately capture all the movements and changes happening in a scene. Existing methods often struggle with this task, either relying on templates or only working well with less dynamic scenes, which limits their effectiveness.
What's the solution?
The authors developed a new approach that uses a compact set of motion bases to represent how objects move in 3D space. They also combined various data sources, like depth maps and 2D motion tracks, to create a clearer picture of the scene. By doing this, they were able to produce accurate 3D representations of dynamic scenes from regular videos without needing special equipment.
Why it matters?
This research is significant because it advances the field of computer vision, making it possible to analyze and understand complex movements in videos more effectively. This could have applications in areas like film production, virtual reality, and robotics, where understanding motion is crucial.
Abstract
Monocular dynamic reconstruction is a challenging and long-standing vision problem due to the highly ill-posed nature of the task. Existing approaches are limited in that they either depend on templates, are effective only in quasi-static scenes, or fail to model 3D motion explicitly. In this work, we introduce a method capable of reconstructing generic dynamic scenes, featuring explicit, full-sequence-long 3D motion, from casually captured monocular videos. We tackle the under-constrained nature of the problem with two key insights: First, we exploit the low-dimensional structure of 3D motion by representing scene motion with a compact set of SE3 motion bases. Each point's motion is expressed as a linear combination of these bases, facilitating soft decomposition of the scene into multiple rigidly-moving groups. Second, we utilize a comprehensive set of data-driven priors, including monocular depth maps and long-range 2D tracks, and devise a method to effectively consolidate these noisy supervisory signals, resulting in a globally consistent representation of the dynamic scene. Experiments show that our method achieves state-of-the-art performance for both long-range 3D/2D motion estimation and novel view synthesis on dynamic scenes. Project Page: https://shape-of-motion.github.io/