ShapeSplat: A Large-scale Dataset of Gaussian Splats and Their Self-Supervised Pretraining
Qi Ma, Yue Li, Bin Ren, Nicu Sebe, Ender Konukoglu, Theo Gevers, Luc Van Gool, Danda Pani Paudel
2024-08-21
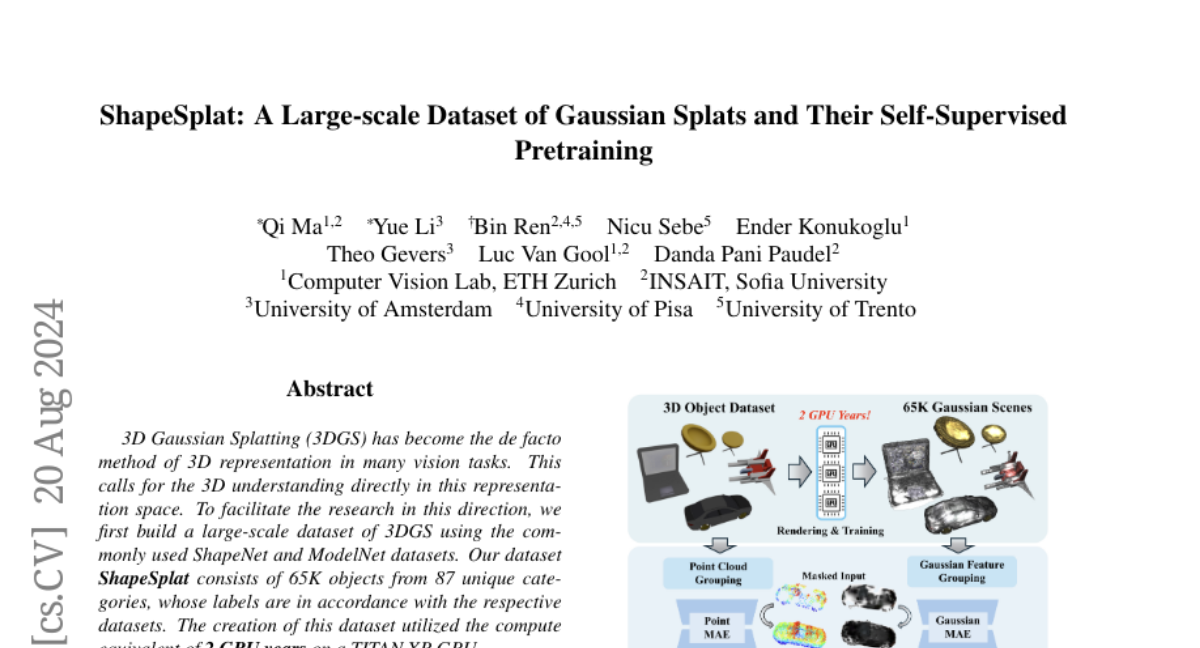
Summary
This paper introduces ShapeSplat, a large dataset designed for improving how machines understand and represent 3D shapes using Gaussian splatting techniques.
What's the problem?
Understanding 3D objects is crucial for many computer vision tasks, but existing methods often struggle to accurately represent and process these shapes. There is a need for a comprehensive dataset that can help train models to better handle 3D representations.
What's the solution?
The authors created the ShapeSplat dataset, which includes 65,000 3D objects from 87 different categories. They used advanced techniques to automatically label these objects and developed a new method called Gaussian-MAE to improve how models learn from Gaussian parameters. This approach helps in both unsupervised pretraining and supervised fine-tuning for tasks like classification and segmentation.
Why it matters?
This research is important because it provides a valuable resource for improving the capabilities of models in understanding 3D shapes. By enhancing how machines learn from complex data, this work can lead to advancements in various applications, such as robotics, virtual reality, and computer graphics.
Abstract
3D Gaussian Splatting (3DGS) has become the de facto method of 3D representation in many vision tasks. This calls for the 3D understanding directly in this representation space. To facilitate the research in this direction, we first build a large-scale dataset of 3DGS using the commonly used ShapeNet and ModelNet datasets. Our dataset ShapeSplat consists of 65K objects from 87 unique categories, whose labels are in accordance with the respective datasets. The creation of this dataset utilized the compute equivalent of 2 GPU years on a TITAN XP GPU. We utilize our dataset for unsupervised pretraining and supervised finetuning for classification and segmentation tasks. To this end, we introduce \textit{Gaussian-MAE}, which highlights the unique benefits of representation learning from Gaussian parameters. Through exhaustive experiments, we provide several valuable insights. In particular, we show that (1) the distribution of the optimized GS centroids significantly differs from the uniformly sampled point cloud (used for initialization) counterpart; (2) this change in distribution results in degradation in classification but improvement in segmentation tasks when using only the centroids; (3) to leverage additional Gaussian parameters, we propose Gaussian feature grouping in a normalized feature space, along with splats pooling layer, offering a tailored solution to effectively group and embed similar Gaussians, which leads to notable improvement in finetuning tasks.