ShieldGemma: Generative AI Content Moderation Based on Gemma
Wenjun Zeng, Yuchi Liu, Ryan Mullins, Ludovic Peran, Joe Fernandez, Hamza Harkous, Karthik Narasimhan, Drew Proud, Piyush Kumar, Bhaktipriya Radharapu, Olivia Sturman, Oscar Wahltinez
2024-08-01
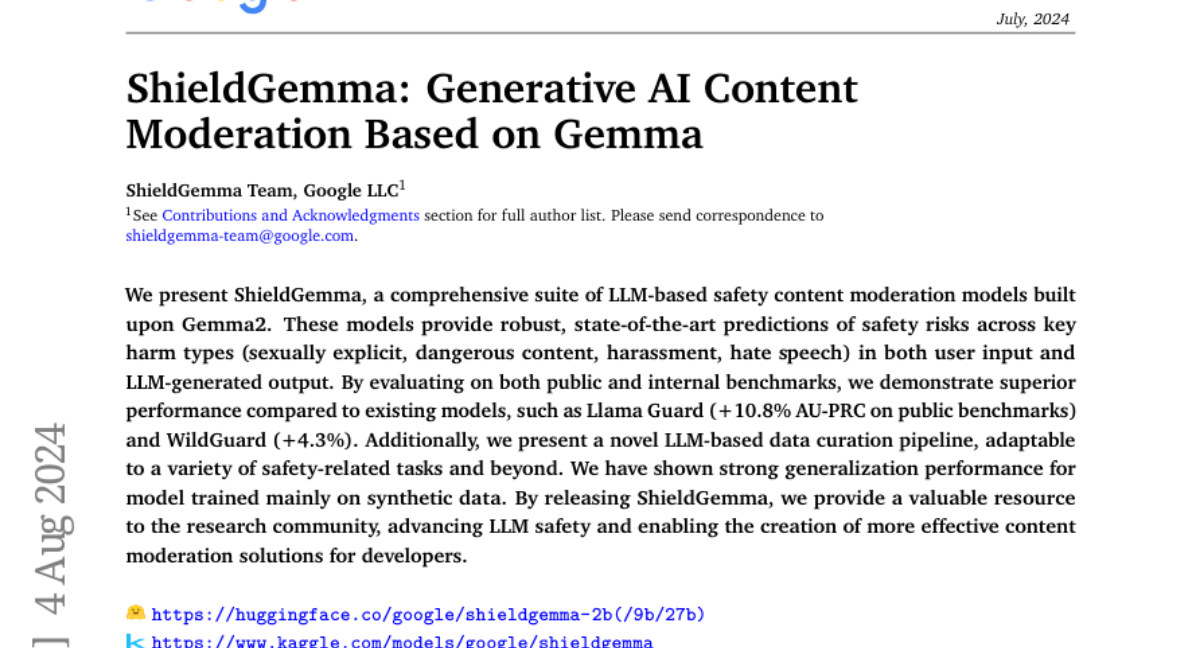
Summary
This paper introduces ShieldGemma, a new system for content moderation that uses advanced AI models to identify and filter harmful content like hate speech, harassment, and explicit material in both user inputs and AI-generated outputs.
What's the problem?
As AI systems become more popular, ensuring that they produce safe and appropriate content is crucial. Many existing moderation systems struggle to accurately detect harmful content, which can lead to negative experiences for users. This is especially important in environments where safety and respect are paramount, such as online communities and educational platforms.
What's the solution?
To tackle this problem, the authors developed ShieldGemma, which is based on the Gemma2 architecture. This system uses a suite of language models that have been trained to predict safety risks across various types of harmful content. ShieldGemma has been tested against other models and has shown better performance in identifying unsafe content. Additionally, it includes a new method for curating data that helps improve the accuracy of moderation tasks across different contexts.
Why it matters?
This research is significant because it enhances the effectiveness of content moderation systems, making online spaces safer for everyone. By providing a reliable tool for identifying harmful content, ShieldGemma can help developers create more responsible AI applications. This is crucial for maintaining trust in AI technologies and ensuring that they are used positively in society.
Abstract
We present ShieldGemma, a comprehensive suite of LLM-based safety content moderation models built upon Gemma2. These models provide robust, state-of-the-art predictions of safety risks across key harm types (sexually explicit, dangerous content, harassment, hate speech) in both user input and LLM-generated output. By evaluating on both public and internal benchmarks, we demonstrate superior performance compared to existing models, such as Llama Guard (+10.8\% AU-PRC on public benchmarks) and WildCard (+4.3\%). Additionally, we present a novel LLM-based data curation pipeline, adaptable to a variety of safety-related tasks and beyond. We have shown strong generalization performance for model trained mainly on synthetic data. By releasing ShieldGemma, we provide a valuable resource to the research community, advancing LLM safety and enabling the creation of more effective content moderation solutions for developers.