ShortCircuit: AlphaZero-Driven Circuit Design
Dimitrios Tsaras, Antoine Grosnit, Lei Chen, Zhiyao Xie, Haitham Bou-Ammar, Mingxuan Yuan
2024-08-20
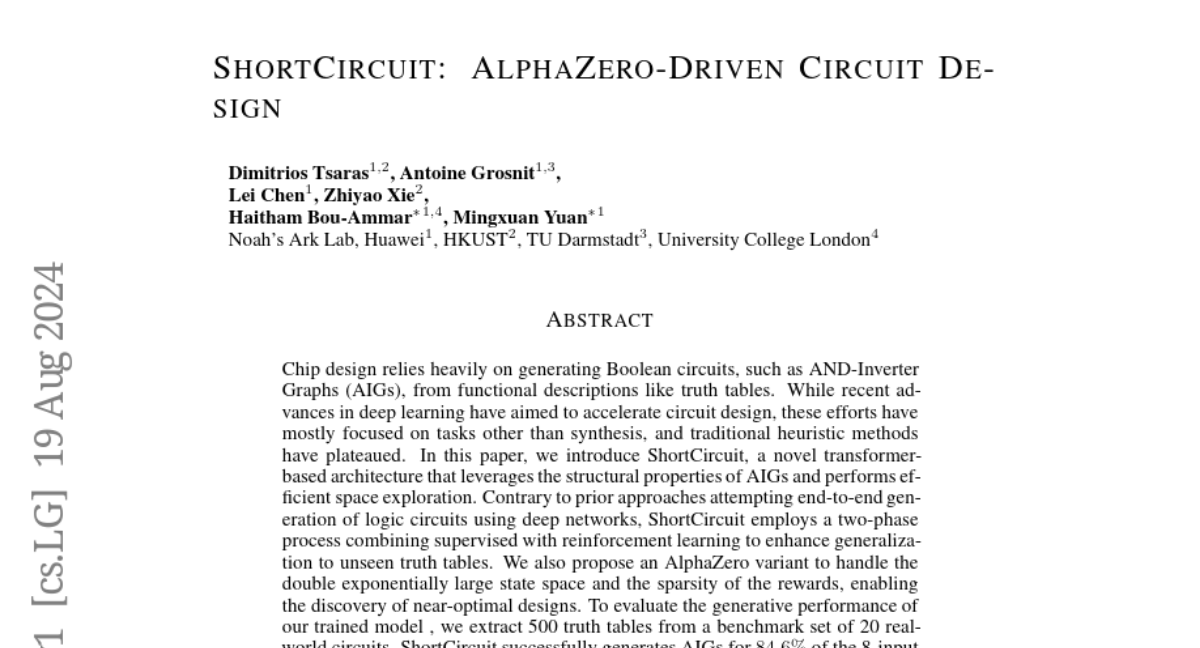
Summary
This paper discusses ShortCircuit, a new approach to designing electronic circuits using a method inspired by AlphaZero, which is a powerful AI model.
What's the problem?
Designing circuits from functional descriptions, like truth tables, can be complex and costly. Traditional methods have limitations and often do not efficiently generate the best circuit designs.
What's the solution?
ShortCircuit introduces a transformer-based architecture that combines supervised learning with reinforcement learning in a two-phase process. This allows the model to explore different circuit designs effectively. It also uses an AlphaZero variant to manage the large number of possible circuit designs and improve the quality of the generated circuits. The model was tested with real-world circuit data and showed significant improvements in generating effective circuit designs.
Why it matters?
This research is important because it advances the field of circuit design by making it faster and more efficient. With better tools for generating circuits, industries can develop new technologies more quickly and at lower costs, which can lead to innovations in electronics and computing.
Abstract
Chip design relies heavily on generating Boolean circuits, such as AND-Inverter Graphs (AIGs), from functional descriptions like truth tables. While recent advances in deep learning have aimed to accelerate circuit design, these efforts have mostly focused on tasks other than synthesis, and traditional heuristic methods have plateaued. In this paper, we introduce ShortCircuit, a novel transformer-based architecture that leverages the structural properties of AIGs and performs efficient space exploration. Contrary to prior approaches attempting end-to-end generation of logic circuits using deep networks, ShortCircuit employs a two-phase process combining supervised with reinforcement learning to enhance generalization to unseen truth tables. We also propose an AlphaZero variant to handle the double exponentially large state space and the sparsity of the rewards, enabling the discovery of near-optimal designs. To evaluate the generative performance of our trained model , we extract 500 truth tables from a benchmark set of 20 real-world circuits. ShortCircuit successfully generates AIGs for 84.6% of the 8-input test truth tables, and outperforms the state-of-the-art logic synthesis tool, ABC, by 14.61% in terms of circuits size.