Should We Really Edit Language Models? On the Evaluation of Edited Language Models
Qi Li, Xiang Liu, Zhenheng Tang, Peijie Dong, Zeyu Li, Xinglin Pan, Xiaowen Chu
2024-10-25
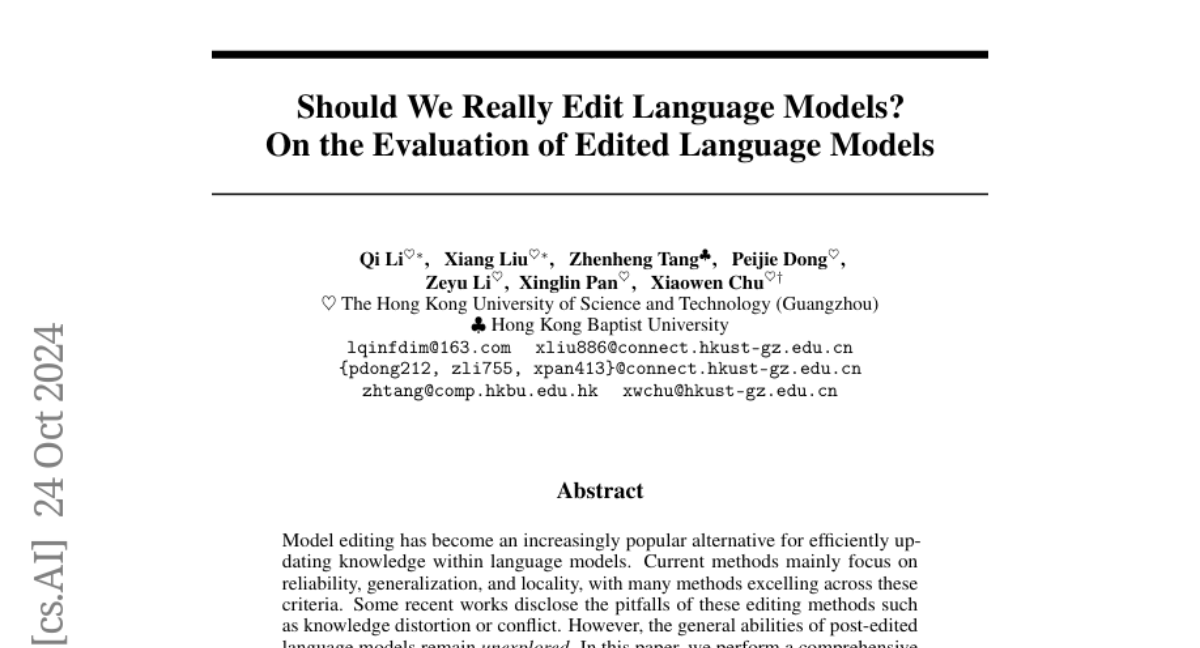
Summary
This paper examines the effectiveness of editing methods used to update knowledge in language models, highlighting the limitations and potential issues that arise from these edits.
What's the problem?
As language models are used more widely, there is a growing need to update their knowledge efficiently. Current editing methods aim to improve reliability and accuracy, but they often lead to problems like knowledge distortion or conflicts. However, how these edits affect the overall abilities of the models has not been thoroughly explored, which raises concerns about their reliability after multiple edits.
What's the solution?
The authors conducted a comprehensive evaluation of various editing methods across different language models. They found that while some models can handle a few edits without significant performance loss, too many edits can disrupt the model's internal knowledge structure. They also discovered that instruction-tuned models are better at maintaining performance after editing, and larger models tend to resist negative effects from edits more effectively. Importantly, they noted that the safety features of edited models are often weakened, even in those designed with safety in mind.
Why it matters?
This research is crucial because it informs developers and researchers about the limitations of current editing techniques for language models. Understanding these limitations can lead to better practices for updating model knowledge and encourage further research into more effective and reliable editing methods, ensuring that language models remain accurate and safe for users.
Abstract
Model editing has become an increasingly popular alternative for efficiently updating knowledge within language models. Current methods mainly focus on reliability, generalization, and locality, with many methods excelling across these criteria. Some recent works disclose the pitfalls of these editing methods such as knowledge distortion or conflict. However, the general abilities of post-edited language models remain unexplored. In this paper, we perform a comprehensive evaluation on various editing methods and different language models, and have following findings. (1) Existing editing methods lead to inevitable performance deterioration on general benchmarks, indicating that existing editing methods maintain the general abilities of the model within only a few dozen edits. When the number of edits is slightly large, the intrinsic knowledge structure of the model is disrupted or even completely damaged. (2) Instruction-tuned models are more robust to editing, showing less performance drop on general knowledge after editing. (3) Language model with large scale is more resistant to editing compared to small model. (4) The safety of the edited model, is significantly weakened, even for those safety-aligned models. Our findings indicate that current editing methods are only suitable for small-scale knowledge updates within language models, which motivates further research on more practical and reliable editing methods. The details of code and reproduction can be found in https://github.com/lqinfdim/EditingEvaluation.