Simplifying, Stabilizing and Scaling Continuous-Time Consistency Models
Cheng Lu, Yang Song
2024-10-17
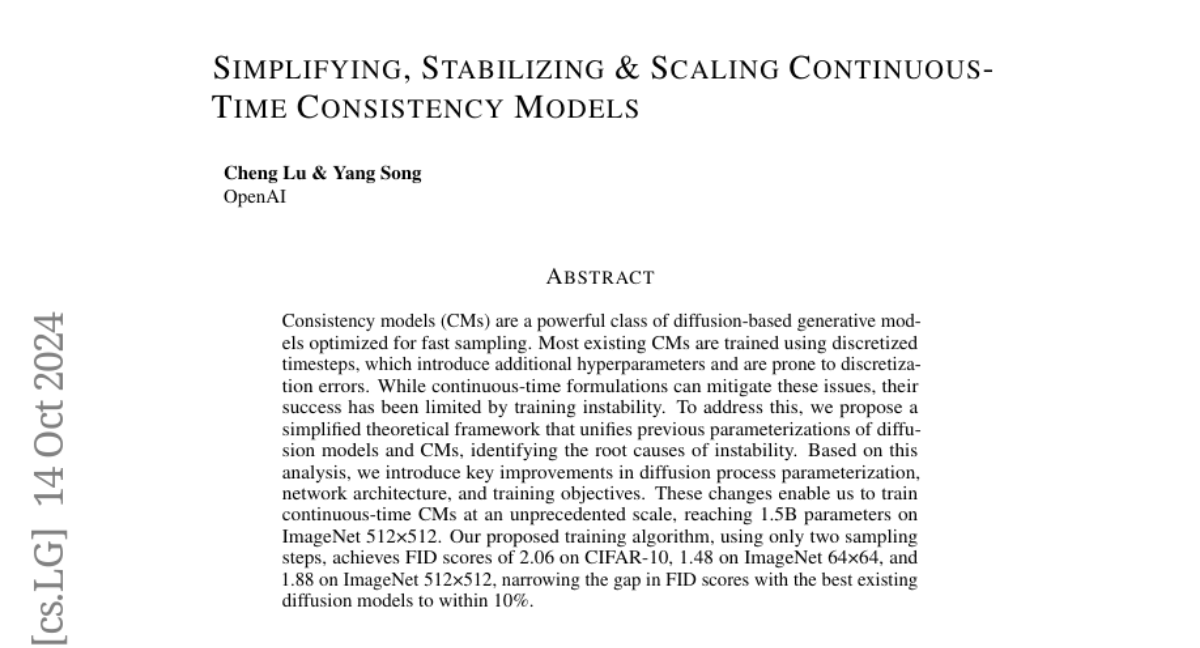
Summary
This paper discusses a new approach called sCM that improves continuous-time consistency models, which are used for generating high-quality images quickly and efficiently.
What's the problem?
Continuous-time consistency models (CMs) have the potential to generate images faster than traditional methods, but they often face issues with training instability and complexity. Many existing models use discrete time steps, which can lead to errors and require complicated settings, making them less efficient.
What's the solution?
To solve these problems, the authors propose a simplified framework that combines previous methods and identifies the main causes of instability in training. They introduce several improvements, such as better ways to set up the diffusion process and adjustments in the model architecture. This allows them to train CMs at a much larger scale—up to 1.5 billion parameters—while achieving high-quality results with only two sampling steps. Their experiments show that this approach significantly narrows the performance gap compared to the best existing models.
Why it matters?
This research is important because it enhances the ability of AI systems to generate images quickly and accurately, which is crucial for applications in fields like video game design, virtual reality, and automated content creation. By making these models more efficient, we can improve their usability in real-world scenarios.
Abstract
Consistency models (CMs) are a powerful class of diffusion-based generative models optimized for fast sampling. Most existing CMs are trained using discretized timesteps, which introduce additional hyperparameters and are prone to discretization errors. While continuous-time formulations can mitigate these issues, their success has been limited by training instability. To address this, we propose a simplified theoretical framework that unifies previous parameterizations of diffusion models and CMs, identifying the root causes of instability. Based on this analysis, we introduce key improvements in diffusion process parameterization, network architecture, and training objectives. These changes enable us to train continuous-time CMs at an unprecedented scale, reaching 1.5B parameters on ImageNet 512x512. Our proposed training algorithm, using only two sampling steps, achieves FID scores of 2.06 on CIFAR-10, 1.48 on ImageNet 64x64, and 1.88 on ImageNet 512x512, narrowing the gap in FID scores with the best existing diffusion models to within 10%.