Sketch2Scene: Automatic Generation of Interactive 3D Game Scenes from User's Casual Sketches
Yongzhi Xu, Yonhon Ng, Yifu Wang, Inkyu Sa, Yunfei Duan, Yang Li, Pan Ji, Hongdong Li
2024-08-09
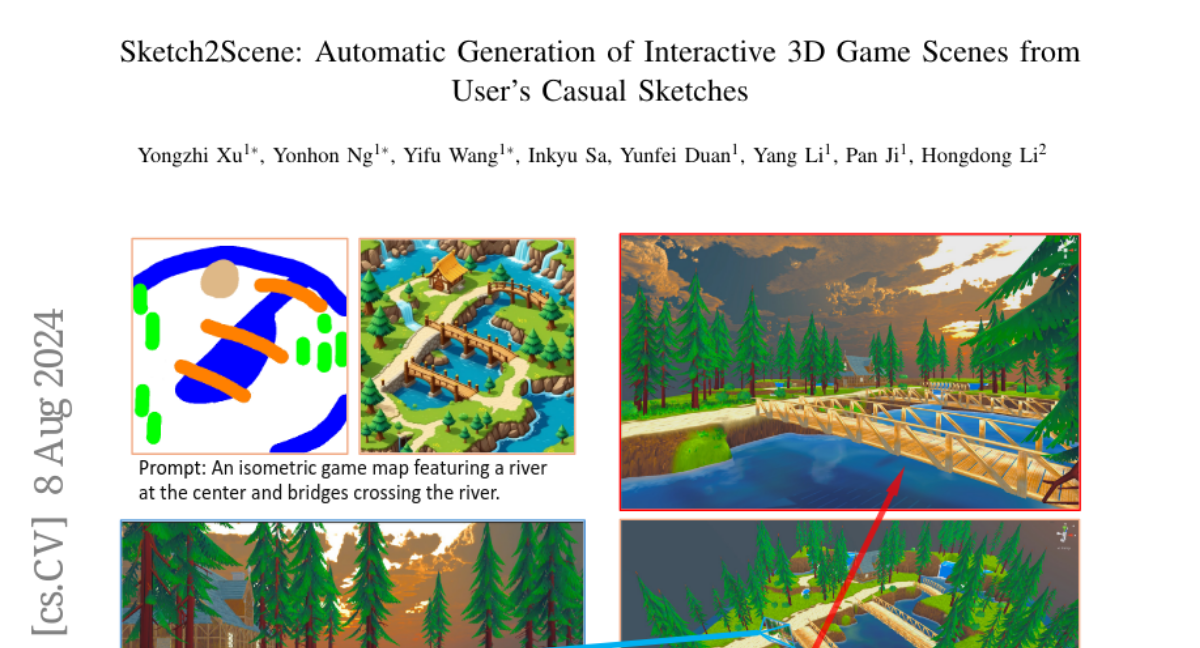
Summary
This paper introduces Sketch2Scene, a new method that allows users to create interactive 3D game scenes just by drawing simple sketches.
What's the problem?
Creating detailed 3D game scenes can be challenging and time-consuming, especially when there isn't enough data available to train models effectively. Traditional methods often require a lot of complex input and can be difficult for casual users who may not have advanced skills in 3D design.
What's the solution?
The authors developed Sketch2Scene, which uses a deep learning approach to automatically generate 3D scenes from hand-drawn sketches. The method first creates a 2D image based on the sketch, which serves as a guide. Then, it breaks down this image into different parts like trees and buildings, and uses these elements to build a complete 3D scene that can be played in game engines like Unity or Unreal. This process simplifies the creation of 3D environments and makes it accessible to more people.
Why it matters?
This research is important because it empowers users to create their own game scenes easily without needing extensive technical knowledge. By allowing anyone to turn their ideas into playable environments quickly, Sketch2Scene can enhance creativity in game development and make the process more inclusive.
Abstract
3D Content Generation is at the heart of many computer graphics applications, including video gaming, film-making, virtual and augmented reality, etc. This paper proposes a novel deep-learning based approach for automatically generating interactive and playable 3D game scenes, all from the user's casual prompts such as a hand-drawn sketch. Sketch-based input offers a natural, and convenient way to convey the user's design intention in the content creation process. To circumvent the data-deficient challenge in learning (i.e. the lack of large training data of 3D scenes), our method leverages a pre-trained 2D denoising diffusion model to generate a 2D image of the scene as the conceptual guidance. In this process, we adopt the isometric projection mode to factor out unknown camera poses while obtaining the scene layout. From the generated isometric image, we use a pre-trained image understanding method to segment the image into meaningful parts, such as off-ground objects, trees, and buildings, and extract the 2D scene layout. These segments and layouts are subsequently fed into a procedural content generation (PCG) engine, such as a 3D video game engine like Unity or Unreal, to create the 3D scene. The resulting 3D scene can be seamlessly integrated into a game development environment and is readily playable. Extensive tests demonstrate that our method can efficiently generate high-quality and interactive 3D game scenes with layouts that closely follow the user's intention.