SlotLifter: Slot-guided Feature Lifting for Learning Object-centric Radiance Fields
Yu Liu, Baoxiong Jia, Yixin Chen, Siyuan Huang
2024-08-14
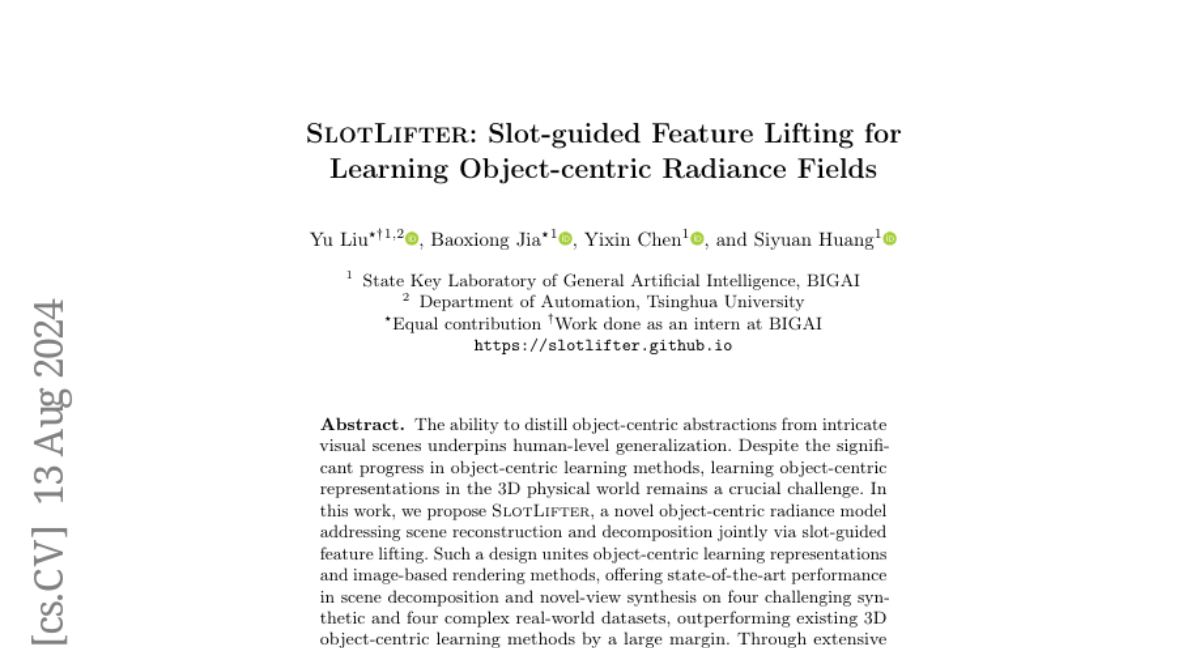
Summary
This paper introduces SlotLifter, a new model designed to improve how we understand and recreate 3D scenes by focusing on individual objects within those scenes.
What's the problem?
Learning to represent and reconstruct 3D objects from complex visual scenes is challenging. Current methods struggle to effectively separate and identify different objects in a scene, which makes it hard to create accurate 3D models.
What's the solution?
SlotLifter combines techniques from object-centric learning and image rendering to address this issue. It uses a method called slot-guided feature lifting, which helps the model focus on individual objects while also considering the entire scene. This approach allows SlotLifter to perform better in breaking down scenes into their components and generating new views of those scenes, outperforming existing methods significantly.
Why it matters?
This research is important because it enhances our ability to create realistic 3D representations of the world. By improving how we can isolate and understand objects in complex scenes, SlotLifter can be applied in various fields such as virtual reality, gaming, and robotics, leading to better simulations and interactions with digital environments.
Abstract
The ability to distill object-centric abstractions from intricate visual scenes underpins human-level generalization. Despite the significant progress in object-centric learning methods, learning object-centric representations in the 3D physical world remains a crucial challenge. In this work, we propose SlotLifter, a novel object-centric radiance model addressing scene reconstruction and decomposition jointly via slot-guided feature lifting. Such a design unites object-centric learning representations and image-based rendering methods, offering state-of-the-art performance in scene decomposition and novel-view synthesis on four challenging synthetic and four complex real-world datasets, outperforming existing 3D object-centric learning methods by a large margin. Through extensive ablative studies, we showcase the efficacy of designs in SlotLifter, revealing key insights for potential future directions.