Smaller Language Models Are Better Instruction Evolvers
Tingfeng Hui, Lulu Zhao, Guanting Dong, Yaqi Zhang, Hua Zhou, Sen Su
2024-12-17
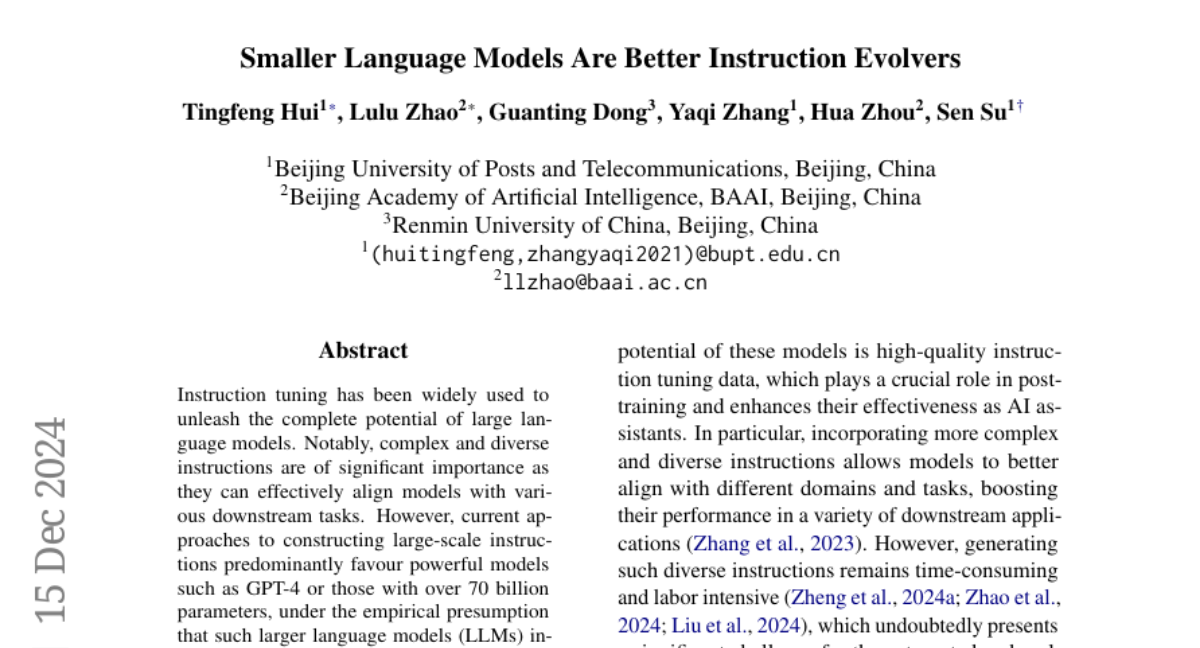
Summary
This paper discusses how smaller language models (SLMs) can be more effective than larger ones (LLMs) when it comes to evolving and improving instructions for various tasks. It challenges the common belief that bigger models are always better.
What's the problem?
Many researchers assume that larger language models, like GPT-4, are superior for generating complex instructions because they have more parameters. However, this assumption may not hold true, as these large models can struggle with creating effective and diverse instructions, which are crucial for aligning models with different tasks.
What's the solution?
The authors conducted experiments comparing SLMs and LLMs in evolving instructions. They found that SLMs can create better and more varied instructions than LLMs. To measure this effectively, they introduced a new evaluation method called Instruction Complex-Aware IFD (IC-IFD), which takes into account the complexity of the instructions being generated. This allows for a more accurate assessment of how well the models perform in instruction evolution.
Why it matters?
This research is important because it suggests that smaller language models can be just as effective, if not better, than larger ones in specific tasks like instruction generation. This could lead to more efficient use of resources in AI development, making it easier and cheaper to create powerful models without needing massive computational power.
Abstract
Instruction tuning has been widely used to unleash the complete potential of large language models. Notably, complex and diverse instructions are of significant importance as they can effectively align models with various downstream tasks. However, current approaches to constructing large-scale instructions predominantly favour powerful models such as GPT-4 or those with over 70 billion parameters, under the empirical presumption that such larger language models (LLMs) inherently possess enhanced capabilities. In this study, we question this prevalent assumption and conduct an in-depth exploration into the potential of smaller language models (SLMs) in the context of instruction evolution. Extensive experiments across three scenarios of instruction evolution reveal that smaller language models (SLMs) can synthesize more effective instructions than LLMs. Further analysis demonstrates that SLMs possess a broader output space during instruction evolution, resulting in more complex and diverse variants. We also observe that the existing metrics fail to focus on the impact of the instructions. Thus, we propose Instruction Complex-Aware IFD (IC-IFD), which introduces instruction complexity in the original IFD score to evaluate the effectiveness of instruction data more accurately. Our source code is available at: https://github.com/HypherX/Evolution-Analysis{https://github.com/HypherX/Evolution-Analysis}