SMITE: Segment Me In TimE
Amirhossein Alimohammadi, Sauradip Nag, Saeid Asgari Taghanaki, Andrea Tagliasacchi, Ghassan Hamarneh, Ali Mahdavi Amiri
2024-10-25
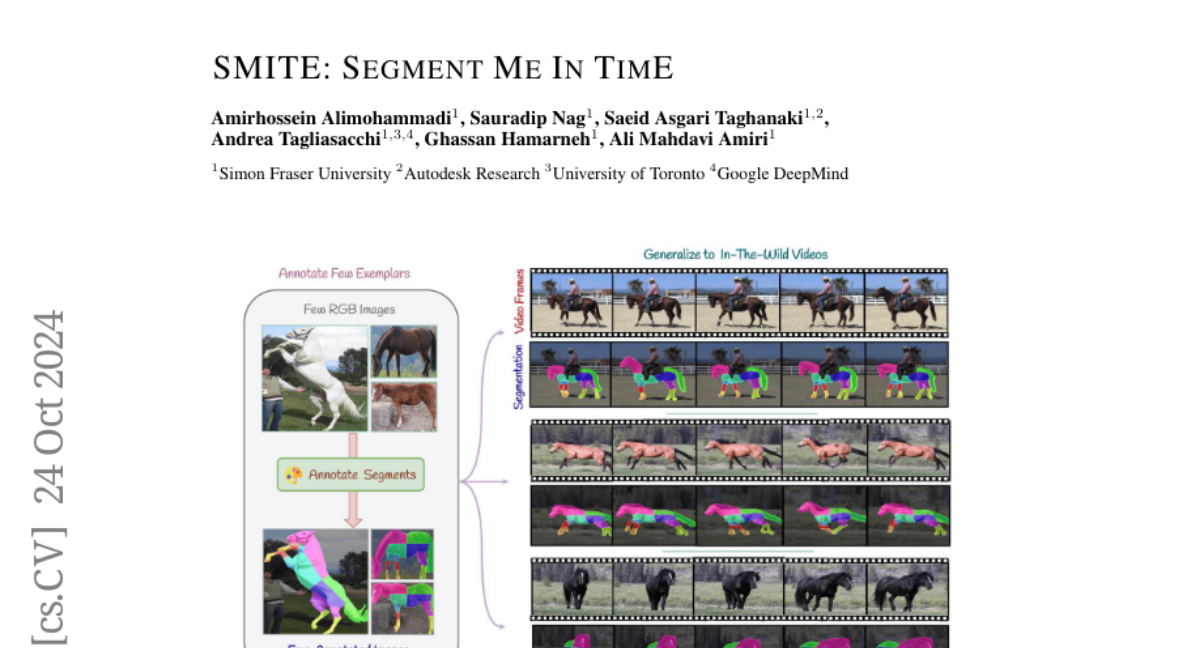
Summary
This paper introduces SMITE, a new method for segmenting objects in videos that ensures consistent labeling across frames while allowing for flexible segmentation based on user input.
What's the problem?
Segmenting objects in videos is challenging because each pixel needs to be accurately labeled, and these labels must remain consistent as the video progresses. The difficulty increases when the number of segments can vary or when only a few sample images are available for reference, making it hard to track objects accurately over time.
What's the solution?
The authors propose SMITE, which uses a pre-trained text-to-image diffusion model combined with a tracking mechanism to improve segmentation. This approach allows the system to manage different segmentation scenarios effectively and ensures that labels remain consistent across video frames. By leveraging existing models and adding tracking capabilities, SMITE can adapt to various segmentation needs without requiring extensive new training.
Why it matters?
This research is important because it enhances the ability to accurately segment and track objects in videos, which is crucial for applications like video editing, surveillance, and autonomous driving. By improving how we can label and track objects over time, SMITE can lead to better performance in many fields that rely on video analysis.
Abstract
Segmenting an object in a video presents significant challenges. Each pixel must be accurately labelled, and these labels must remain consistent across frames. The difficulty increases when the segmentation is with arbitrary granularity, meaning the number of segments can vary arbitrarily, and masks are defined based on only one or a few sample images. In this paper, we address this issue by employing a pre-trained text to image diffusion model supplemented with an additional tracking mechanism. We demonstrate that our approach can effectively manage various segmentation scenarios and outperforms state-of-the-art alternatives.