SocialGPT: Prompting LLMs for Social Relation Reasoning via Greedy Segment Optimization
Wanhua Li, Zibin Meng, Jiawei Zhou, Donglai Wei, Chuang Gan, Hanspeter Pfister
2024-10-30
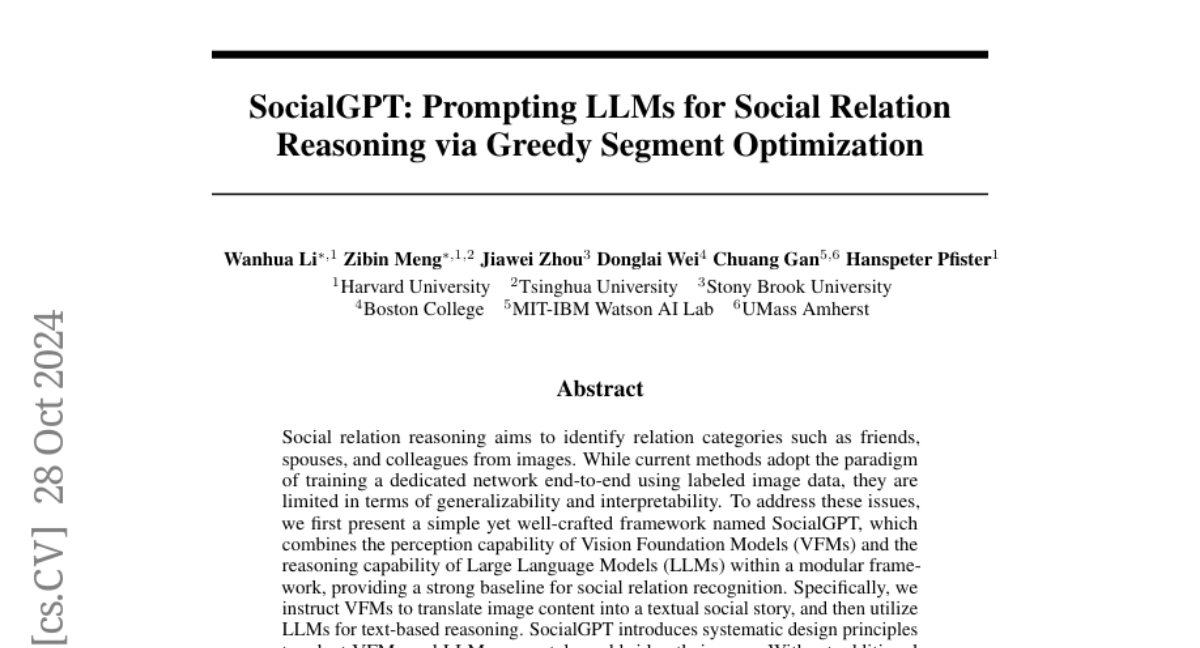
Summary
This paper presents SocialGPT, a framework that helps identify social relationships in images by combining visual understanding with language processing.
What's the problem?
Identifying social relationships, like whether people in an image are friends, family, or colleagues, can be challenging. Current methods rely on training specific models with labeled data, which can be limiting and hard to interpret. This makes it difficult to apply these methods to new situations or different types of images.
What's the solution?
To solve this problem, the authors developed SocialGPT, which integrates Vision Foundation Models (VFMs) that analyze images and Large Language Models (LLMs) that understand and generate text. The VFMs convert image content into a narrative or social story, while the LLMs reason about this text to identify relationships. They also introduced a technique called Greedy Segment Prompt Optimization (GSPO) to improve how prompts are designed for the LLMs, making the process more efficient and effective without needing additional training.
Why it matters?
This research is important because it shows how combining different types of AI can improve our ability to understand complex social interactions in images. By providing interpretable explanations for their decisions, SocialGPT can help researchers and practitioners better analyze social dynamics in various fields, such as psychology, marketing, and social media.
Abstract
Social relation reasoning aims to identify relation categories such as friends, spouses, and colleagues from images. While current methods adopt the paradigm of training a dedicated network end-to-end using labeled image data, they are limited in terms of generalizability and interpretability. To address these issues, we first present a simple yet well-crafted framework named {\name}, which combines the perception capability of Vision Foundation Models (VFMs) and the reasoning capability of Large Language Models (LLMs) within a modular framework, providing a strong baseline for social relation recognition. Specifically, we instruct VFMs to translate image content into a textual social story, and then utilize LLMs for text-based reasoning. {\name} introduces systematic design principles to adapt VFMs and LLMs separately and bridge their gaps. Without additional model training, it achieves competitive zero-shot results on two databases while offering interpretable answers, as LLMs can generate language-based explanations for the decisions. The manual prompt design process for LLMs at the reasoning phase is tedious and an automated prompt optimization method is desired. As we essentially convert a visual classification task into a generative task of LLMs, automatic prompt optimization encounters a unique long prompt optimization issue. To address this issue, we further propose the Greedy Segment Prompt Optimization (GSPO), which performs a greedy search by utilizing gradient information at the segment level. Experimental results show that GSPO significantly improves performance, and our method also generalizes to different image styles. The code is available at https://github.com/Mengzibin/SocialGPT.