SPARK: Multi-Vision Sensor Perception and Reasoning Benchmark for Large-scale Vision-Language Models
Youngjoon Yu, Sangyun Chung, Byung-Kwan Lee, Yong Man Ro
2024-08-23
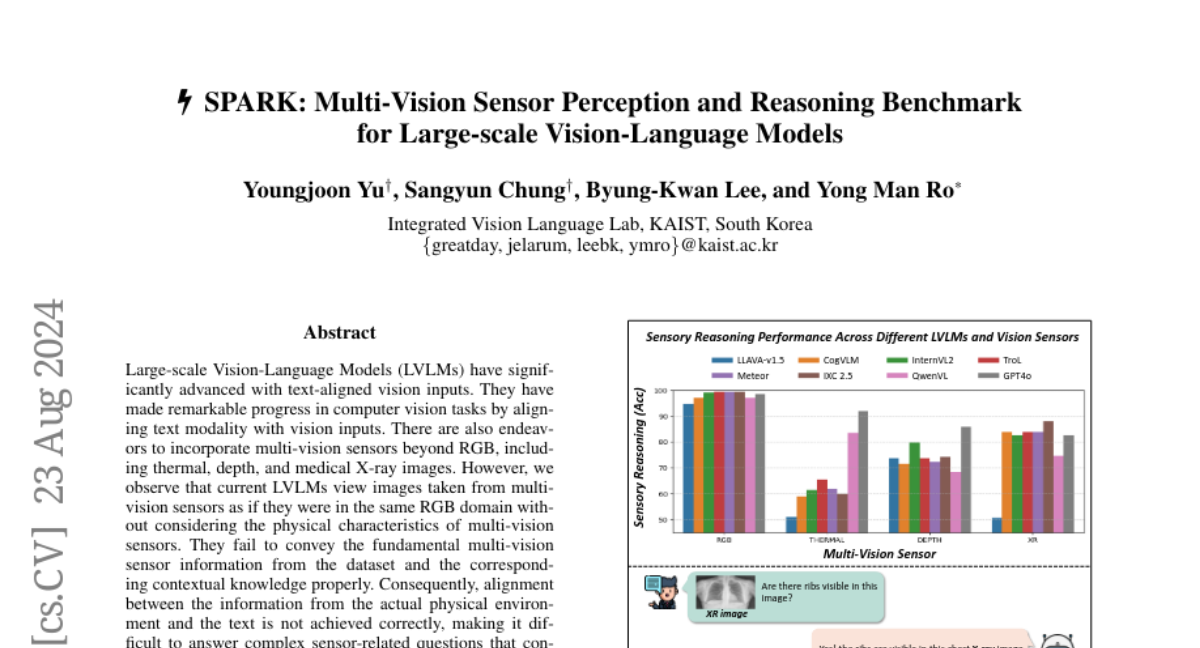
Summary
This paper introduces SPARK, a new benchmark designed to evaluate how well Large-scale Vision-Language Models (LVLMs) understand and reason about information from various types of sensors, not just regular RGB images.
What's the problem?
LVLMs have improved in understanding images when paired with text, but they often treat different types of sensor data (like thermal or depth images) as if they were just regular color images. This oversight means they miss important details that could help them answer questions about the physical world accurately.
What's the solution?
To address this issue, the authors created SPARK, which includes over 6,000 test samples that focus on how well LVLMs can perceive and reason using multi-vision sensor data. They tested ten leading LVLMs to see how well they could handle questions related to these different types of sensor information.
Why it matters?
This research is important because it helps identify the weaknesses in current LVLMs when dealing with complex sensor data. By improving how these models understand various types of visual information, we can enhance their performance in real-world applications like robotics, healthcare imaging, and environmental monitoring.
Abstract
Large-scale Vision-Language Models (LVLMs) have significantly advanced with text-aligned vision inputs. They have made remarkable progress in computer vision tasks by aligning text modality with vision inputs. There are also endeavors to incorporate multi-vision sensors beyond RGB, including thermal, depth, and medical X-ray images. However, we observe that current LVLMs view images taken from multi-vision sensors as if they were in the same RGB domain without considering the physical characteristics of multi-vision sensors. They fail to convey the fundamental multi-vision sensor information from the dataset and the corresponding contextual knowledge properly. Consequently, alignment between the information from the actual physical environment and the text is not achieved correctly, making it difficult to answer complex sensor-related questions that consider the physical environment. In this paper, we aim to establish a multi-vision Sensor Perception And Reasoning benchmarK called SPARK that can reduce the fundamental multi-vision sensor information gap between images and multi-vision sensors. We generated 6,248 vision-language test samples automatically to investigate multi-vision sensory perception and multi-vision sensory reasoning on physical sensor knowledge proficiency across different formats, covering different types of sensor-related questions. We utilized these samples to assess ten leading LVLMs. The results showed that most models displayed deficiencies in multi-vision sensory reasoning to varying extents. Codes and data are available at https://github.com/top-yun/SPARK