SpaRP: Fast 3D Object Reconstruction and Pose Estimation from Sparse Views
Chao Xu, Ang Li, Linghao Chen, Yulin Liu, Ruoxi Shi, Hao Su, Minghua Liu
2024-08-20
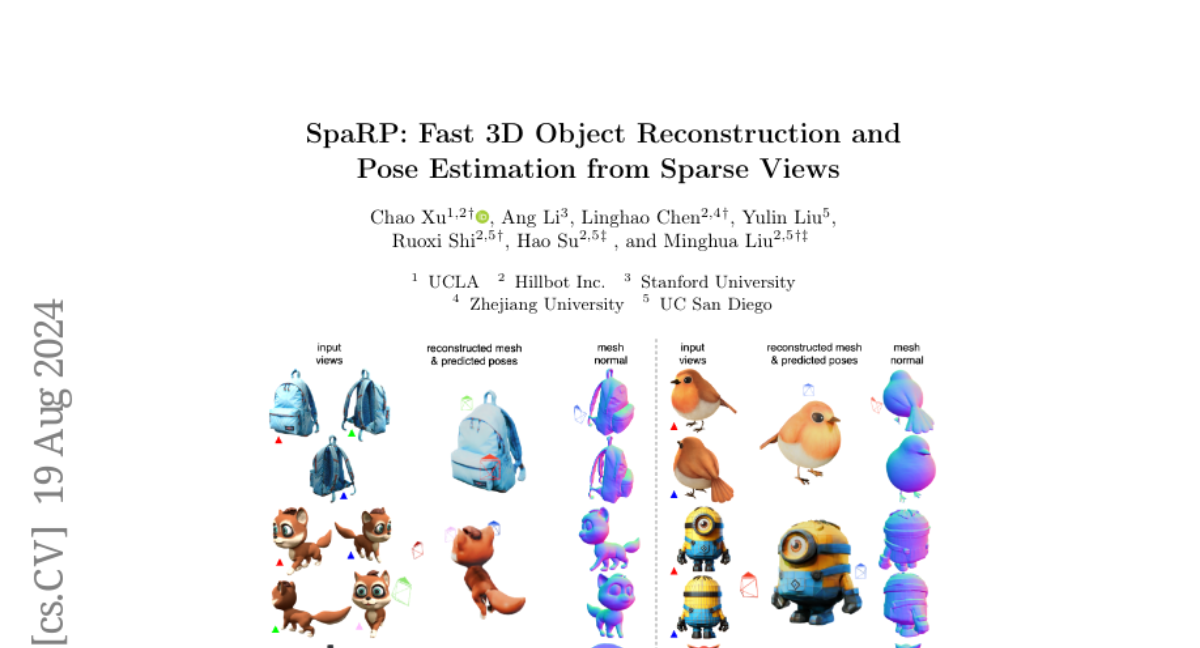
Summary
This paper introduces SpaRP, a new method for quickly reconstructing 3D objects and estimating camera positions using only a few 2D images from different angles.
What's the problem?
Reconstructing 3D objects from images can be difficult, especially when you only have a few pictures that don't show the entire object. Existing methods often require many images and can produce inaccurate results, making it hard to create reliable 3D models.
What's the solution?
SpaRP solves this problem by using a two-step approach. First, it uses a diffusion model to understand the spatial relationships between the sparse images. Then, it reconstructs a detailed 3D model and estimates the camera positions based on those images. This method is efficient and can generate high-quality 3D models in about 20 seconds, significantly faster than traditional methods.
Why it matters?
This research is important because it makes it easier and faster to create accurate 3D models from limited image data. This capability can be useful in various fields such as gaming, virtual reality, and robotics, where quick and reliable 3D reconstruction is needed.
Abstract
Open-world 3D generation has recently attracted considerable attention. While many single-image-to-3D methods have yielded visually appealing outcomes, they often lack sufficient controllability and tend to produce hallucinated regions that may not align with users' expectations. In this paper, we explore an important scenario in which the input consists of one or a few unposed 2D images of a single object, with little or no overlap. We propose a novel method, SpaRP, to reconstruct a 3D textured mesh and estimate the relative camera poses for these sparse-view images. SpaRP distills knowledge from 2D diffusion models and finetunes them to implicitly deduce the 3D spatial relationships between the sparse views. The diffusion model is trained to jointly predict surrogate representations for camera poses and multi-view images of the object under known poses, integrating all information from the input sparse views. These predictions are then leveraged to accomplish 3D reconstruction and pose estimation, and the reconstructed 3D model can be used to further refine the camera poses of input views. Through extensive experiments on three datasets, we demonstrate that our method not only significantly outperforms baseline methods in terms of 3D reconstruction quality and pose prediction accuracy but also exhibits strong efficiency. It requires only about 20 seconds to produce a textured mesh and camera poses for the input views. Project page: https://chaoxu.xyz/sparp.