Spectra: A Comprehensive Study of Ternary, Quantized, and FP16 Language Models
Ayush Kaushal, Tejas Pandey, Tejas Vaidhya, Aaryan Bhagat, Irina Rish
2024-07-18
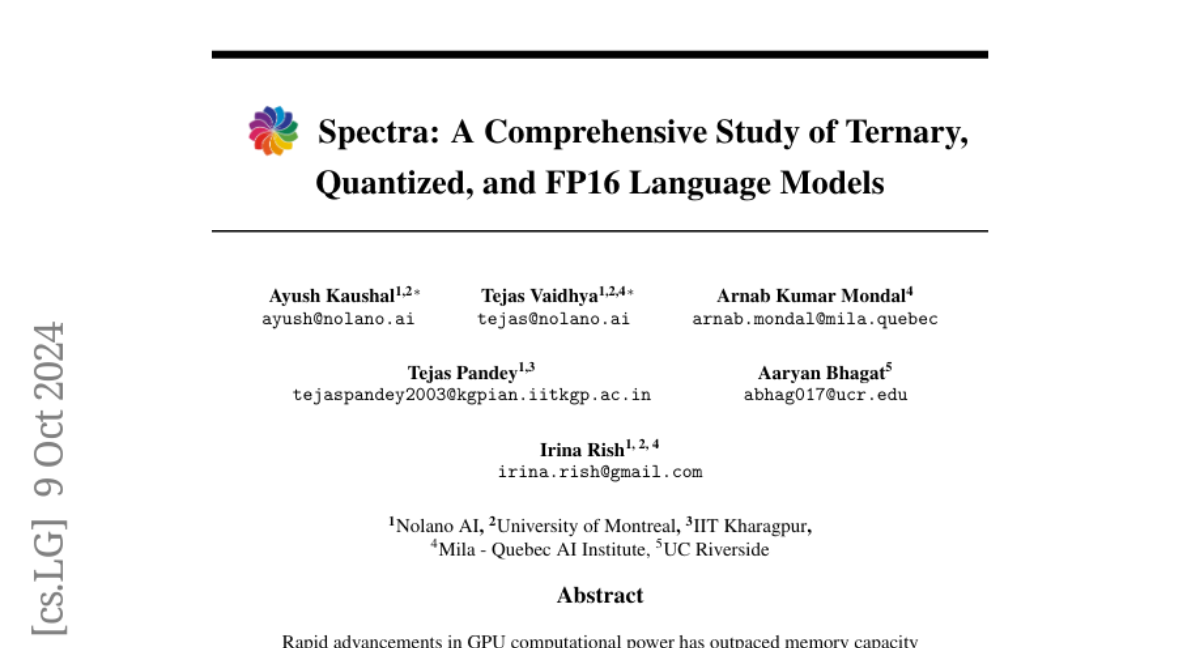
Summary
This paper presents Spectra, a suite of language models that explores different ways to compress and optimize large language models (LLMs) using ternary and quantized formats.
What's the problem?
Large language models require a lot of memory and processing power, which can be a problem when trying to use them efficiently. Traditional methods for reducing their size, like post-training quantization, often lead to a drop in performance when using very low bit precision (like below 4 bits). There is also limited understanding of how well models trained directly at low bit widths (like ternary or binary models) perform compared to standard models.
What's the solution?
To tackle these issues, the authors created the Spectra LLM suite, which includes 54 different language models ranging from 99 million to 3.9 billion parameters. This suite features FloatLMs (traditional floating-point models), QuantLMs (quantized models at various bit widths), and TriLMs (ternary models). The TriLMs were specifically designed to perform well even with fewer bits, showing that they can match or exceed the performance of larger floating-point models in certain tasks while being smaller in size. They also released over 500 checkpoints of these models for further research.
Why it matters?
This research is significant because it helps improve our understanding of how different model compression techniques affect performance. By providing a comprehensive suite of models and data, Spectra enables researchers to develop more efficient AI systems that can operate effectively even with limited resources, making advanced language processing technology more accessible.
Abstract
Post-training quantization is the leading method for addressing memory-related bottlenecks in LLM inference, but unfortunately, it suffers from significant performance degradation below 4-bit precision. An alternative approach involves training compressed models directly at a low bitwidth (e.g., binary or ternary models). However, the performance, training dynamics, and scaling trends of such models are not yet well understood. To address this issue, we train and openly release the Spectra LLM suite consisting of 54 language models ranging from 99M to 3.9B parameters, trained on 300B tokens. Spectra includes FloatLMs, post-training quantized QuantLMs (3, 4, 6, and 8 bits), and ternary LLMs (TriLMs) - our improved architecture for ternary language modeling, which significantly outperforms previously proposed ternary models of a given size (in bits), matching half-precision models at scale. For example, TriLM 3.9B is (bit-wise) smaller than the half-precision FloatLM 830M, but matches half-precision FloatLM 3.9B in commonsense reasoning and knowledge benchmarks. However, TriLM 3.9B is also as toxic and stereotyping as FloatLM 3.9B, a model six times larger in size. Additionally, TriLM 3.9B lags behind FloatLM in perplexity on validation splits and web-based corpora but performs better on less noisy datasets like Lambada and PennTreeBank. To enhance understanding of low-bitwidth models, we are releasing 500+ intermediate checkpoints of the Spectra suite at https://github.com/NolanoOrg/SpectraSuite{https://github.com/NolanoOrg/SpectraSuite}.