Splatfacto-W: A Nerfstudio Implementation of Gaussian Splatting for Unconstrained Photo Collections
Congrong Xu, Justin Kerr, Angjoo Kanazawa
2024-07-18
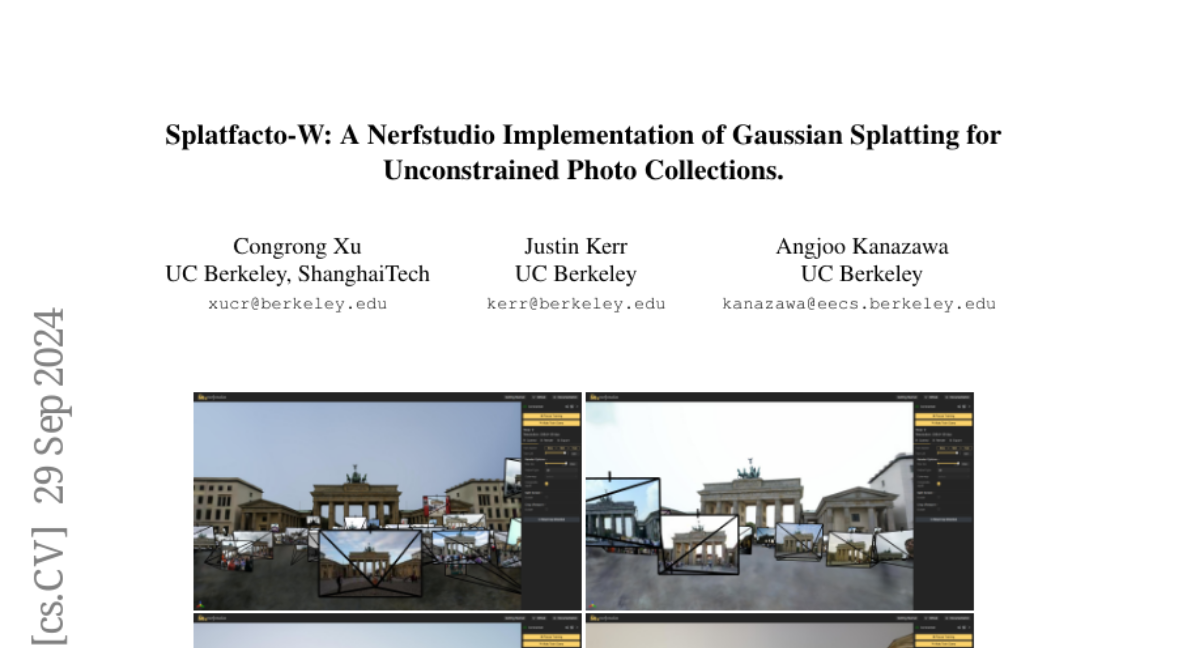
Summary
This paper introduces Splatfacto-W, a new method for creating 3D representations from collections of photos taken in everyday situations, using a technique called Gaussian splatting.
What's the problem?
Reconstructing 3D scenes from photos that are not taken under controlled conditions (like in a studio) is very challenging. Issues such as changing lighting and objects blocking the view (occlusions) make it hard to accurately recreate the scene. Traditional methods struggle with these variations, leading to poor quality 3D models.
What's the solution?
Splatfacto-W addresses these challenges by using Gaussian splatting, which represents the scene as flexible 3D blobs (Gaussians) instead of rigid shapes. This allows the system to adapt better to different appearances and lighting conditions. The method also includes advanced techniques for modeling backgrounds and handling temporary objects that may block the view. As a result, Splatfacto-W can create high-quality 3D scenes in real-time, improving both the speed and accuracy of the reconstruction process compared to previous methods.
Why it matters?
This research is important because it significantly enhances how we can create 3D models from everyday photos, making it easier to visualize real-world environments. This has applications in fields like virtual reality, gaming, and urban planning, where accurate and quick scene reconstruction is crucial. By improving the ability to work with 'in-the-wild' images, Splatfacto-W opens up new possibilities for using 3D modeling in various industries.
Abstract
Novel view synthesis from unconstrained in-the-wild image collections remains a significant yet challenging task due to photometric variations and transient occluders that complicate accurate scene reconstruction. Previous methods have approached these issues by integrating per-image appearance features embeddings in Neural Radiance Fields (NeRFs). Although 3D Gaussian Splatting (3DGS) offers faster training and real-time rendering, adapting it for unconstrained image collections is non-trivial due to the substantially different architecture. In this paper, we introduce Splatfacto-W, an approach that integrates per-Gaussian neural color features and per-image appearance embeddings into the rasterization process, along with a spherical harmonics-based background model to represent varying photometric appearances and better depict backgrounds. Our key contributions include latent appearance modeling, efficient transient object handling, and precise background modeling. Splatfacto-W delivers high-quality, real-time novel view synthesis with improved scene consistency in in-the-wild scenarios. Our method improves the Peak Signal-to-Noise Ratio (PSNR) by an average of 5.3 dB compared to 3DGS, enhances training speed by 150 times compared to NeRF-based methods, and achieves a similar rendering speed to 3DGS. Additional video results and code integrated into Nerfstudio are available at https://kevinxu02.github.io/splatfactow/.