Stable Flow: Vital Layers for Training-Free Image Editing
Omri Avrahami, Or Patashnik, Ohad Fried, Egor Nemchinov, Kfir Aberman, Dani Lischinski, Daniel Cohen-Or
2024-11-22
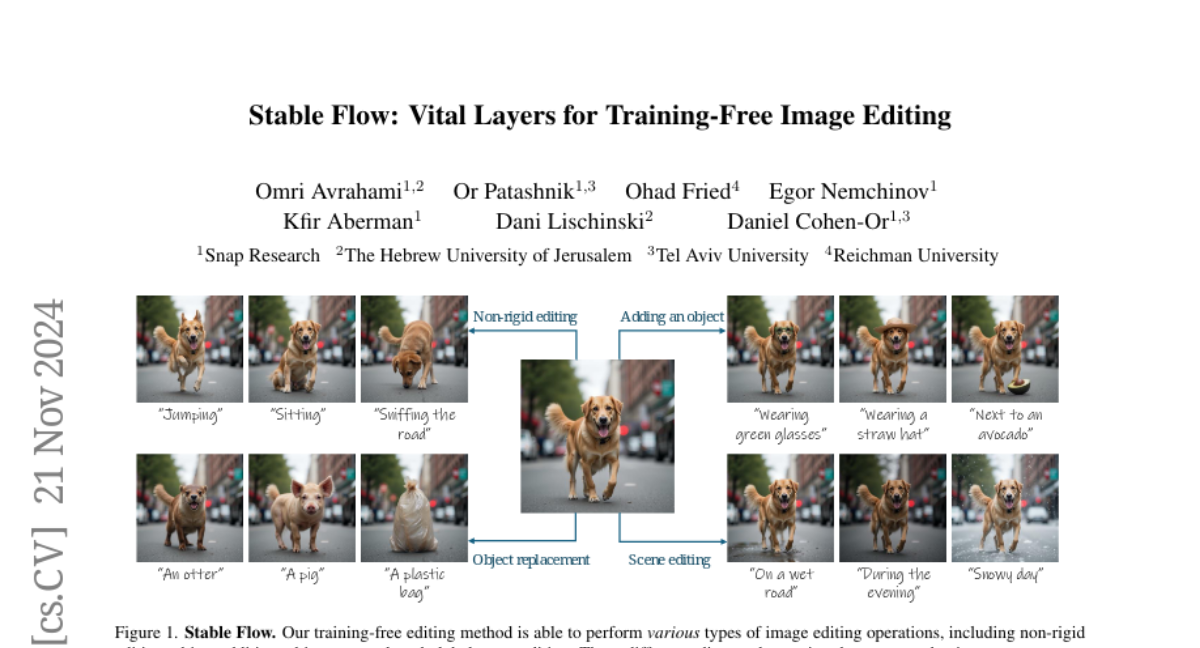
Summary
This paper introduces Stable Flow, a method for editing images using a new approach that allows for training-free image manipulation by identifying important layers in a model called the Diffusion Transformer (DiT).
What's the problem?
Traditional models for image editing often require extensive training and can be complex to use. The new Diffusion Transformer architecture has limitations in generating diverse images and lacks a clear structure for how to edit images effectively, making it difficult to know where to apply changes.
What's the solution?
Stable Flow proposes an automatic method to find 'vital layers' within the DiT that are crucial for creating images. By injecting attention features into these layers, the model can perform consistent edits, such as changing shapes or adding objects, without needing to retrain the model. Additionally, the authors improved the process of editing real images by introducing a better method for transforming images into a format suitable for editing.
Why it matters?
This research is important because it simplifies the process of image editing by allowing users to make changes without extensive training. By focusing on key layers in the model, Stable Flow enables more controlled and stable edits, making it easier for artists and designers to manipulate images effectively. This advancement can lead to more accessible tools for creative professionals and enhance the quality of digital content creation.
Abstract
Diffusion models have revolutionized the field of content synthesis and editing. Recent models have replaced the traditional UNet architecture with the Diffusion Transformer (DiT), and employed flow-matching for improved training and sampling. However, they exhibit limited generation diversity. In this work, we leverage this limitation to perform consistent image edits via selective injection of attention features. The main challenge is that, unlike the UNet-based models, DiT lacks a coarse-to-fine synthesis structure, making it unclear in which layers to perform the injection. Therefore, we propose an automatic method to identify "vital layers" within DiT, crucial for image formation, and demonstrate how these layers facilitate a range of controlled stable edits, from non-rigid modifications to object addition, using the same mechanism. Next, to enable real-image editing, we introduce an improved image inversion method for flow models. Finally, we evaluate our approach through qualitative and quantitative comparisons, along with a user study, and demonstrate its effectiveness across multiple applications. The project page is available at https://omriavrahami.com/stable-flow